Invited Talks
Large datasets are increasing used to train AI models for addressing social problems, including problems in health. The societal impact of biased AI models has been widely discussed. However, sometimes missing in the conversation is the role of historical policies and injustices in shaping available data and outcomes. Evaluating data and algorithms through a historical lens could be critical for social change.
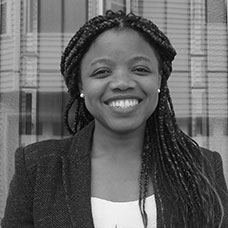
Elaine Nsoesie
Elaine Nsoesie is an Associate Professor in the Department of Global Health at the Boston University School of Public Health. She also leads the Racial Data Tracker project at the Boston University Center for Antiracist Research. She is a Data Science Faculty Fellow and was a Founding Faculty of the Boston University Faculty of Computing and Data Sciences. She currently co-leads the Artificial Intelligence/Machine Learning Consortium to Advance Health Equity and Researcher Diversity (AIM-AHEAD) Program at the National Institutes of Health through the Intergovernmental Personnel Act (IPA) Mobility Program.
Her research is primarily focused on the use of data and technology to advance health equity. She has published extensively in peer-reviewed literature about opportunities and challenges involved in the use of data from social media, search engines, mobile phones, and other digital technologies for public health surveillance.
Her work approaches health equity from multiple angles, including increasing representation of communities typically underrepresented in data science through programs like Data Science Africa and AIM-AHEAD; addressing bias in health data and algorithms; and using data and policy to advance racial equity. She has collaborated with local departments of health in the U.S. to improve disease surveillance systems, international organizations like UNICEF and UNDP, and served as a Data & Innovation Fellow in the Directorate of Science, Technology, and Innovation (DSTI), The President’s Office, Sierra Leone.
Nsoesie was born and raised in Cameroon.
Nsoesie completed her PhD in Computational Epidemiology from the Genetics, Bioinformatics and Computational Biology program at Virginia Tech, and her PhD dissertation, Sensitivity Analysis and Forecasting in Network Epidemiology Models, at the Network Dynamics and Simulations Science Lab at Virginia Tech BioComplexity Institute. After postdoctoral associate positions at Harvard Medical School and Boston Children’s Hospital, she joined the faculty of the Institute for Health Metrics and Evaluation (IHME) at the University of Washington.
Sofia Crespo shares about her artistic practice and journey using generative systems, especially neural networks, as a means to explore speculative lifeforms, and how technology can bring us closer to the natural world.
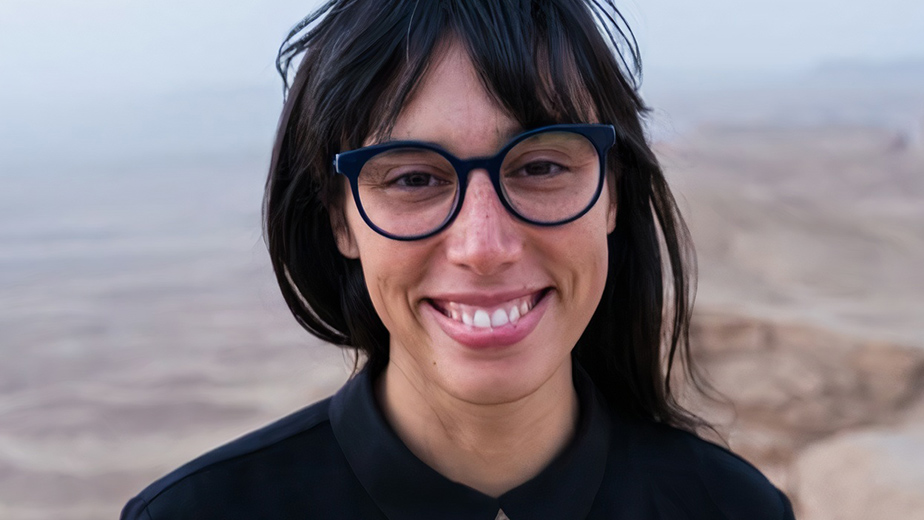
Sofia Crespo
Sofia Crespo is an artist working with a huge interest in biology-inspired technologies. One of her main focuses is the way organic life uses artificial mechanisms to simulate itself and evolve, this implying the idea that technologies are a biased product of the organic life that created them and not a completely separated object. Crespo looks at the similarities between techniques of AI image formation, and the way that humans express themselves creatively and cognitively recognize their world.
Her work brings into question the potential of AI in artistic practice and its ability to reshape our understandings of creativity. On the side, she is also hugely concerned with the dynamic change in the role of the artists working with machine learning techniques. She’s also the co-founder of Entangled Others Studio.
For reliable machine learning, overcoming the distribution shift is one of the most important challenges. In this talk, I will first give an overview of the classical importance weighting approach to distribution shift adaptation, which consists of an importance estimation step and an importance-weighted training step. Then, I will present a more recent approach that simultaneously estimates the importance weight and trains a predictor. Finally, I will discuss a more challenging scenario of continuous distribution shifts, where the data distributions change continuously over time.
Masashi Sugiyama
Masashi Sugiyama is Director of the RIKEN Center for Advanced Intelligence Project and Professor of Complexity Science and Engineering at the University of Tokyo. His research interests include the theory, algorithms, and applications of machine learning. He has written several books on machine learning, including Density Ratio Estimation in Machine Learning (Cambridge, 2012). He served as program co-chair and general co-chair of the NIPS conference in 2015 and 2016, respectively, and received the Japan Academy Medal in 2017.
Recent large language models (LLMs) have enabled significant advancements for open-domain dialogue systems due to their ability to generate coherent natural language responses to any user request. Their ability to memorize and perform compositional reasoning has enabled accurate execution of dialogue related tasks, such as language understanding and response generation. However, these models suffer from limitations, such as, hallucination, undesired capturing of biases, difficulty in generalization to specific policies, and lack of interpretability.. To tackle these issues, the natural language processing community proposed methods, such as, injecting knowledge into language models during training or inference, retrieving related knowledge using multi-step inference and API/tools, and so on. In this talk, I plan to provide an overview of our and other work that aim to address these challenges.
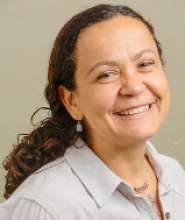
Dilek Hakkani-Tur
Dilek Hakkani-Tür is a senior principal scientist at Amazon Alexa AI focusing on enabling natural dialogues with machines. Prior to joining Amazon, she was leading the dialogue research group at Google (2016-2018), a principal researcher at Microsoft Research (2010-2016), International Computer Science Institute (ICSI, 2006-2010) and AT&T Labs-Research (2001-2005). She received her BSc degree from Middle East Technical Univ, in 1994, and MSc and PhD degrees from Bilkent Univ., Department of Computer Engineering, in 1996 and 2000, respectively. Her research interests include conversational AI, natural language and speech processing, spoken dialogue systems, and machine learning for language processing. She has over 80 patents that were granted and co-authored more than 300 papers in natural language and speech processing. She received several best paper awards for publications she co-authored on conversational systems, including her earlier work on active learning for dialogue systems, from IEEE Signal Processing Society, ISCA and EURASIP. She served as an associate editor for IEEE Transactions on Audio, Speech and Language Processing (2005-2008), member of the IEEE Speech and Language Technical Committee (2009-2014), area editor for speech and language processing for Elsevier's Digital Signal Processing Journal and IEEE Signal Processing Letters (2011-2013), and served on the ISCA Advisory Council (2015-2019). She also served as the Editor-in-Chief of the IEEE/ACM Transactions on Audio, Speech and Language Processing (2019-2021), an IEEE Distinguished Industry Speaker (2021) and is a fellow of the IEEE (2014) and ISCA (2014).
The success of deep learning has hinged on learned functions dramatically outperforming hand-designed functions for many tasks. However, we still train models using hand designed optimizers acting on hand designed loss functions. I will argue that these hand designed components are typically mismatched to the desired behavior, and that we can expect meta-learned optimizers to perform much better. I will discuss the challenges and pathologies that make meta-training learned optimizers difficult. These include: chaotic and high variance meta-loss landscapes; extreme computational costs for meta-training; lack of comprehensive meta-training datasets; challenges designing learned optimizers with the right inductive biases; challenges interpreting the method of action of learned optimizers. I will share solutions to some of these challenges. I will show experimental results where learned optimizers outperform hand-designed optimizers in many contexts, and I will discuss novel capabilities that are enabled by meta-training learned optimizers.

Jascha Sohl-Dickstein
I am a principal scientist in Google DeepMind, where I lead a research team with interests spanning machine learning, physics, and neuroscience. I'm most (in)famous for inventing diffusion models. My recent work has focused on theory of overparameterized neural networks, meta-training of learned optimizers, and understanding the capabilities of large language models. Before working at Google I was a visiting scholar in Surya Ganguli's lab at Stanford University, and an academic resident at Khan Academy. I earned my PhD in 2012 in the Redwood Center for Theoretical Neuroscience at UC Berkeley, in Bruno Olshausen's lab. Prior to my PhD, I worked on Mars.
With a growing trend of employing machine learning (ML) models to assist decision making, it is vital to inspect both the models and their corresponding data for potential systematic deviations in order to achieve trustworthy ML applications. Such inspected data may be used in training, testing or generated by the models themselves. Understanding of systematic deviations is particularly crucial in resource-limited and/or error-sensitive domains, such as healthcare. In this talk, I reflect on our recent work which has utilized automated identification and characterization of systematic deviations for various tasks in healthcare, including; data quality understanding; temporal drift; heterogeneous intervention effects analysis; and new class detection. Moreover, AI-driven scientific discovery is increasingly being facilitated using generative models. And I will share how our data-centric and multi-level evaluation framework helps to quantify the capabilities of generative models in both domain-agnostic and interpretable ways, using material science as a use case. Beyond the analysis of curated datasets which are often utilized to train ML models, similar data-centric analysis should also be considered on traditional data sources, such as textbooks. To this end I will conclude by presenting a recent collaborative work on automated representation analysis in dermatology academic materials.

Girmaw Abebe Tadesse
Girmaw is a Principal Research Scientist and Manager at Microsoft AI for Good Research Lab which aims to develop AI solutions for critical problems across sectors including agriculture, healthcare, biodiversity, etc. Prior to that he was a Staff Research Scientist at IBM Research Africa working on detecting and characterizing systematic deviations in data and machine learning models. At IBM Research, Girmaw led multiple projects in trustworthy AI including evaluation of generative models, representation analysis in academic materials and data-driven insight extraction from public healthy surveys, with active collaborations with external institutions such as Bill & Melinda Gates Foundation, Stanford University, Oxford University and Harvard University. Previously, Girmaw also worked as a Postdoctoral Researcher at the University of Oxford, where he primarily developed deep learning techniques to assist diagnosis of multiple diseases, with collaborations with clinicians and hospitals in China and Vietnam. Girmaw completed his PhD at Queen Mary University of London, under the Erasmus Mundus Double Doctorate Program in Interactive and Cognitive Environments, with a focus on computer vision and machine learning algorithms for human activity recognition using wearable cameras. He has interned/worked in various research groups across Europe, including the UPC-BarcelonaTech (Spain), KU Leuven (Belgium), and INESC-ID (Portugal). Girmaw is an Executive Member for IEEE Kenya Section, and he is currently serving as a reviewer and program committee member for multiple top-tier AI focused journals and conferences.