The Eleventh International Conference on Learning Representations
Kigali Rwanda
Mon May 1 — Fri May 5
Registration
Pricing » Registration 2023 Registration Cancellation Policy »
Conference Site
Start Here Invited Talks Schedule Papers Workshops
Announcements
-
ICLR.cc will be down for server maintenance intermittantly over the weekend of April 19th starting at 6:30 pm pacific time.
-
ICLR 2024 Hotel Reservations available HERE
- Local Poster Printing Service - Free Delivery to Venue - Order HERE
- Allowable Poster Dimensions - HERE
- Call for Socials - applications closed
- Careers site is open! Check out opportunities HERE
- Press Accrediation now OPEN
- Authors of eligible TMLR publications can submit a request to present in ICLR conference! Find more here.
- BEWARE of Predatory ICLR conferences being promoted through the World Academy of Science, Engineering and Technology organization.
Sponsors
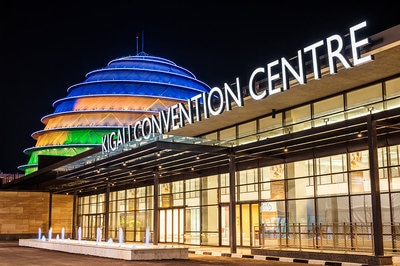
We are very excited to be holding the ICLR 2024 annual conference in Kigali, Rwanda this year from May 1-5, 2023. The conference will be located at the beautiful Kigali Convention Centre / Radisson Blu Hotel location which was recently built and opened for events and visitors in 2016. The Kigali Convention Centre is located 5 kilometers from the Kigali International Airport.
The in-person conference will also provide viewing and virtual participation for those attendees who are unable to come to Kigali, including a static virtual exhibitor booth for most sponsors.
We look forward to answering any questions you may have, and hopefully seeing you in Kigali.
Latest ICLR Blog Entries [ All Entries ]
Oct 09, 2024 | Assisting ICLR 2025 reviewers with feedback |
Aug 22, 2024 | Extended partnership pilot with TMLR for ICLR 2025 |
May 07, 2024 | ICLR 2024 Test of Time Award |
May 07, 2024 | ICLR 2024 Outstanding Paper Awards |
May 06, 2024 | Code of Ethics Cases at ICLR 2024 |
May 01, 2024 | ICLR 2024 Mentoring Chats |
Apr 22, 2024 | Hugging Face Demo Site |
Apr 15, 2024 | Announcing ICLR 2024 Invited Speakers |
Apr 02, 2024 | Blogposts Track ICLR 2024 : Announcing Accepted Blogposts |
Jan 08, 2024 | Announcing the Accepted Workshops at ICLR 2024 |
Important Dates
About Us
The International Conference on Learning Representations (ICLR) is the premier gathering of professionals dedicated to the advancement of the branch of artificial intelligence called representation learning, but generally referred to as deep learning.
ICLR is globally renowned for presenting and publishing cutting-edge research on all aspects of deep learning used in the fields of artificial intelligence, statistics and data science, as well as important application areas such as machine vision, computational biology, speech recognition, text understanding, gaming, and robotics.
Participants at ICLR span a wide range of backgrounds, from academic and industrial researchers, to entrepreneurs and engineers, to graduate students and postdocs.
A non-exhaustive list of relevant topics explored at the conference include:
- unsupervised, semi-supervised, and supervised representation learning
- representation learning for planning and reinforcement learning
- representation learning for computer vision and natural language processing
- metric learning and kernel learning
- sparse coding and dimensionality expansion
- hierarchical models
- optimization for representation learning
- learning representations of outputs or states
- optimal transport
- theoretical issues in deep learning
- societal considerations of representation learning including fairness, safety, privacy, and interpretability, and explainability
- visualization or interpretation of learned representations
- implementation issues, parallelization, software platforms, hardware
- climate, sustainability
- applications in audio, speech, robotics, neuroscience, biology, or any other field