[ AD11 ]
Large language models (LMs) have been shown to memorize parts of their training data, and when prompted appropriately, they will emit the memorized training data verbatim. This is undesirable because memorization violates privacy (exposing user data), degrades utility (repeated easy-to-memorize text is often low quality), and hurts fairness (some texts are memorized over others).We describe three log-linear relationships that quantify the degree to which LMs emit memorized training data. Memorization significantly grows as we increase (1) the capacity of a model, (2) the number of times an example has been duplicated, and (3) the number of tokens of context used to prompt the model. Surprisingly, we find the situation becomes complicated when generalizing these results across model families. On the whole, we find that memorization in LMs is more prevalent than previously believed and will likely get worse as models continues to scale, at least without active mitigations.
[ AD4 ]
Dynamical systems are found in innumerable forms across the physical and biological sciences, yet all these systems fall naturally into equivalence classes: conservative or dissipative, stable or unstable, compressible or incompressible. Predicting these classes from data remains an essential open challenge in computational physics on which existing time-series classification methods struggle. Here, we propose, phase2vec, an embedding method that learns high-quality, physically-meaningful representations of low-dimensional dynamical systems without supervision. Our embeddings are produced by a convolutional backbone that extracts geometric features from flow data and minimizes a physically-informed vector field reconstruction loss. The trained architecture can not only predict the equations of unseen data, but also produces embeddings that encode meaningful physical properties of input data (e.g. stability of fixed points, conservation of energy, and the incompressibility of flows) more faithfully than standard blackbox classifiers and state-of-the-art time series classification techniques. We additionally apply our embeddings to the analysis of meteorological data, showing we can detect climatically meaningful features. Collectively, our results demonstrate the viability of embedding approaches for the discovery of dynamical features in physical systems.
[ AD12 ]
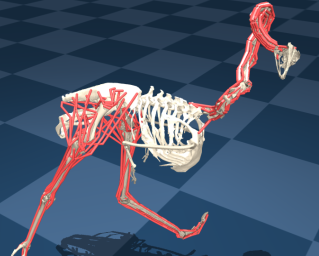
Muscle-actuated organisms are capable of learning an unparalleled diversity of dexterous movements despite their vast amount of muscles. Reinforcement learning (RL) on large musculoskeletal models, however, has not been able to show similar performance. We conjecture that ineffective exploration in large overactuated action spaces is a key problem.This is supported by the finding that common exploration noise strategies are inadequate in synthetic examples of overactuated systems. We identify differential extrinsic plasticity (DEP), a method from the domain of self-organization, as being able to induce state-space covering exploration within seconds of interaction. By integrating DEP into RL, we achieve fast learning of reaching and locomotion in musculoskeletal systems, outperforming current approaches in all considered tasks in sample efficiency and robustness.
[ AD10 ]
Sparse Neural Networks (SNNs) have received voluminous attention predominantly due to growing computational and memory footprints of consistently exploding parameter count in large-scale models. Similar to their dense counterparts, recent SNNs generalize just as well and are equipped with numerous favorable benefits (e.g., low complexity, high scalability, and robustness), sometimes even better than the original dense networks. As research effort is focused on developing increasingly sophisticated sparse algorithms, it is startling that a comprehensive benchmark to evaluate the effectiveness of these algorithms has been highly overlooked. In absence of a carefully crafted evaluation benchmark, most if not all, sparse algorithms are evaluated against fairly simple and naive tasks (eg. CIFAR-10/100, ImageNet, GLUE, etc.), which can potentially camouflage many advantages as well unexpected predicaments of SNNs. In pursuit of a more general evaluation and unveiling the true potential of sparse algorithms, we introduce “Sparsity May Cry” Benchmark (SMC-Bench), a collection of carefully-curated 4 diverse tasks with 10 datasets, that accounts for capturing a wide range of domain-specific and sophisticated knowledge. Our systemic evaluation of the most representative sparse algorithms reveals an important obscured observation: the state-of-the-art magnitude- and/or gradient-based sparse algorithms seemingly fail to perform on SMC-Bench when applied out-of-the-box, sometimes …
[ AD1 ]
Latent manifolds provide a compact characterization of neural population activity and of shared co-variability across brain areas. Nonetheless, existing statistical tools for extracting neural manifolds face limitations in terms of interpretability of latents with respect to task variables, and can be hard to apply to datasets with no trial repeats. Here we propose a novel probabilistic framework that allows for interpretable partitioning of population variability within and across areas in the context of naturalistic behavior. Our approach for task aligned manifold estimation (TAME-GP) explicitly partitions variability into private and shared sources which can themselves be subdivided in task-relevant and task irrelevant components, uses a realistic Poisson noise model, and introduces temporal smoothing of latent trajectories in the form of a Gaussian Process prior. This TAME-GP graphical model allows for robust estimation of task-relevant variability in local population responses, and of shared co-variability between brain areas. We demonstrate the efficiency of our estimator on within model and biologically motivated simulated data. We also apply it to several datasets of neural population recordings during behavior. Overall, our results demonstrate the capacity of TAME-GP to capture meaningful intra- and inter-area neural variability with single trial resolution.
[ AD1 ]
Deep Reinforcement Learning (RL) has emerged as a powerful paradigm for training neural policies to solve complex control tasks. However, these policies tend to be overfit to the exact specifications of the task and environment they were trained on, and thus do not perform well when conditions deviate slightly or when composed hierarchically to solve even more complex tasks. Recent work has shown that training a mixture of policies, as opposed to a single one, that are driven to explore different regions of the state-action space can address this shortcoming by generating a diverse set of behaviors, referred to as skills, that can be collectively used to great effect in adaptation tasks or for hierarchical planning. This is typically realized by including a diversity term - often derived from information theory - in the objective function optimized by RL. However these approaches often require careful hyperparameter tuning to be effective. In this work, we demonstrate that less widely-used neuroevolution methods, specifically Quality Diversity (QD), are a competitive alternative to information-theory-augmented RL for skill discovery. Through an extensive empirical evaluation comparing eight state-of-the-art algorithms (four flagship algorithms from each line of work) on the basis of (i) metrics directly evaluating the …
[ AD12 ]

Reinforcement learning (RL) agents can leverage batches of previously collected data to extract a reasonable control policy. An emerging issue in this offline RL setting, however, is that the bootstrapping update underlying many of our methods suffers from insufficient action-coverage: standard max operator may select a maximal action that has not been seen in the dataset. Bootstrapping from these inaccurate values can lead to overestimation and even divergence. There are a growing number of methods that attempt to approximate an in-sample max, that only uses actions well-covered by the dataset. We highlight a simple fact: it is more straightforward to approximate an in-sample softmax using only actions in the dataset. We show that policy iteration based on the in-sample softmax converges, and that for decreasing temperatures it approaches the in-sample max. We derive an In-Sample Actor-Critic (AC), using this in-sample softmax, and show that it is consistently better or comparable to existing offline RL methods, and is also well-suited to fine-tuning. We release the code at github.com/hwang-ua/inac_pytorch.
[ Auditorium ]
We introduce Token Merging (ToMe), a simple method to increase the throughput of existing ViT models without needing to train. ToMe gradually combines similar tokens in a transformer using a general and light-weight matching algorithm that is as fast as pruning while being more accurate. Off-the-shelf, ToMe can 2x the throughput of state-of-the-art ViT-L @ 512 and ViT-H @ 518 models on images and 2.2x the throughput of ViT-L on video with only a 0.2-0.3% accuracy drop in each case. ToMe can also easily be applied during training, improving in practice training speed up to 2x for MAE fine-tuning on video. Training with ToMe further minimizes accuracy drop, leading to 2x the throughput of ViT-B on audio for only a 0.4% mAP drop. Qualitatively, we find that ToMe merges object parts into one token, even over multiple frames of video. Overall, ToMe’s accuracy and speed are competitive with state-of-the-art on images, video, and audio.
[ AD10 ]
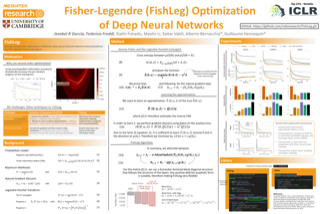
Incorporating second-order gradient information (curvature) into optimization can dramatically reduce the number of iterations required to train machine learning models. In natural gradient descent, such information comes from the Fisher information matrix which yields a number of desirable properties. As exact natural gradient updates are intractable for large models, successful methods such as KFAC and sequels approximate the Fisher in a structured form that can easily be inverted. However, this requires model/layer-specific tensor algebra and certain approximations that are often difficult to justify. Here, we use ideas from Legendre-Fenchel duality to learn a direct and efficiently evaluated model for the product of the inverse Fisher with any vector, in an online manner, leading to natural gradient steps that get progressively more accurate over time despite noisy gradients. We prove that the resulting “Fisher-Legendre” (FishLeg) optimizer converges to a (global) minimum of non-convex functions satisfying the PL condition, which applies in particular to deep linear networks. On standard auto-encoder benchmarks, we show empirically that FishLeg outperforms standard first-order optimization methods, and performs on par with or better than other second-order methods, especially when using small batches. Thanks to its generality, we expect our approach to facilitate the handling of a variety …
[ AD11 ]
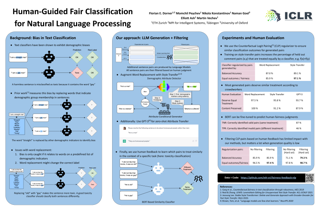
Text classifiers have promising applications in high-stake tasks such as resume screening and content moderation. These classifiers must be fair and avoid discriminatory decisions by being invariant to perturbations of sensitive attributes such as gender or ethnicity. However, there is a gap between human intuition about these perturbations and the formal similarity specifications capturing them. While existing research has started to address this gap, current methods are based on hardcoded word replacements, resulting in specifications with limited expressivity or ones that fail to fully align with human intuition (e.g., in cases of asymmetric counterfactuals). This work proposes novel methods for bridging this gap by discovering expressive and intuitive individual fairness specifications. We show how to leverage unsupervised style transfer and GPT-3's zero-shot capabilities to automatically generate expressive candidate pairs of semantically similar sentences that differ along sensitive attributes. We then validate the generated pairs via an extensive crowdsourcing study, which confirms that a lot of these pairs align with human intuition about fairness in the context of toxicity classification. Finally, we show how limited amounts of human feedback can be leveraged to learn a similarity specification that can be used to train downstream fairness-aware models.
[ Auditorium ]
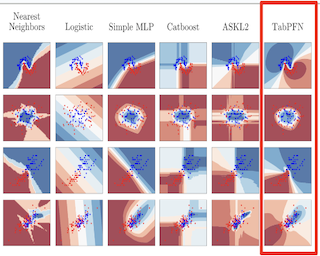
[ AD11 ]
[ AD4 ]
Weather and climate simulations produce petabytes of high-resolution data that are later analyzed by researchers in order to understand climate change or severe weather. We propose a new method of compressing this multidimensional weather and climate data: a coordinate-based neural network is trained to overfit the data, and the resulting parameters are taken as a compact representation of the original grid-based data. While compression ratios range from 300x to more than 3,000x, our method outperforms the state-of-the-art compressor SZ3 in terms of weighted RMSE, MAE. It can faithfully preserve important large scale atmosphere structures and does not introduce significant artifacts.When using the resulting neural network as a 790x compressed dataloader to train the WeatherBench forecasting model, its RMSE increases by less than 2%. The three orders of magnitude compression democratizes access to high-resolution climate data and enables numerous new research directions.
[ AD1 ]
Neurons in the brain are often finely tuned for specific task variables. Moreover, such disentangled representations are highly sought after in machine learning. Here we mathematically prove that simple biological constraints on neurons, namely nonnegativity and energy efficiency in both activity and weights, promote such sought after disentangled representations by enforcing neurons to become selective for single factors of task variation. We demonstrate these constraints lead to disentanglement in a variety of tasks and architectures, including variational autoencoders. We also use this theory to explain why the brain partitions its cells into distinct cell types such as grid and object-vector cells, and also explain when the brain instead entangles representations in response to entangled task factors. Overall, this work provides a mathematical understanding of why single neurons in the brain often represent single human-interpretable factors, and steps towards an understanding task structure shapes the structure of brain representation.
[ AD10 ]
Recent empirical studies on domain generalization (DG) have shown that DG algorithms that perform well on some distribution shifts fail on others, and no state-of-the-art DG algorithm performs consistently well on all shifts. Moreover, real-world data often has multiple distribution shifts over different attributes; hence we introduce multi-attribute distribution shift datasets and find that the accuracy of existing DG algorithms falls even further. To explain these results, we provide a formal characterization of generalization under multi-attribute shifts using a canonical causal graph. Based on the relationship between spurious attributes and the classification label, we obtain realizations of the canonical causal graph that characterize common distribution shifts and show that each shift entails different independence constraints over observed variables. As a result, we prove that any algorithm based on a single, fixed constraint cannot work well across all shifts, providing theoretical evidence for mixed empirical results on DG algorithms. Based on this insight, we develop Causally Adaptive Constraint Minimization (CACM), an algorithm that uses knowledge about the data-generating process to adaptively identify and apply the correct independence constraints for regularization. Results on fully synthetic, MNIST, small NORB, and Waterbirds datasets, covering binary and multi-valued attributes and labels, show that adaptive dataset-dependent …
[ AD12 ]
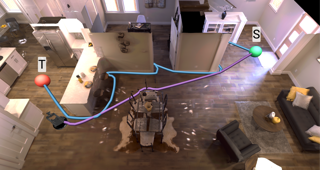
[ AD4 ]
Kohn-Sham Density Functional Theory (KS-DFT) has been traditionally solved by the Self-Consistent Field (SCF) method. Behind the SCF loop is the physics intuition of solving a system of non-interactive single-electron wave functions under an effective potential. In this work, we propose a deep learning approach to KS-DFT. First, in contrast to the conventional SCF loop, we propose to directly minimize the total energy by reparameterizing the orthogonal constraint as a feed-forward computation. We prove that such an approach has the same expressivity as the SCF method, yet reduces the computational complexity from O(N^4) to O(N^3). Second, the numerical integration which involves a summation over the quadrature grids can be amortized to the optimization steps. At each step, stochastic gradient descent (SGD) is performed with a sampled minibatch of the grids. Extensive experiments are carried out to demonstrate the advantage of our approach in terms of efficiency and stability. In addition, we show that our approach enables us to explore more complex neural-based wave functions.
[ AD12 ]
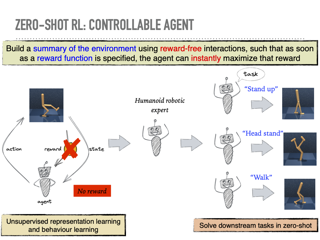
[ AD1 ]
Recent approximations to backpropagation (BP) have mitigated many of BP's computational inefficiencies and incompatibilities with biology, but important limitations still remain. Moreover, the approximations significantly decrease accuracy in benchmarks, suggesting that an entirely different approach may be more fruitful. Here, grounded on recent theory for Hebbian learning in soft winner-take-all networks, we present multilayer SoftHebb, i.e. an algorithm that trains deep neural networks, without any feedback, target, or error signals. As a result, it achieves efficiency by avoiding weight transport, non-local plasticity, time-locking of layer updates, iterative equilibria, and (self-) supervisory or other feedback signals – which were necessary in other approaches. Its increased efficiency and biological compatibility do not trade off accuracy compared to state-of-the-art bio-plausible learning, but rather improve it. With up to five hidden layers and an added linear classifier, accuracies on MNIST, CIFAR-10, STL-10, and ImageNet, respectively reach 99.4%, 80.3%, 76.2%, and 27.3%. In conclusion, SoftHebb shows with a radically different approach from BP that Deep Learning over few layers may be plausible in the brain and increases the accuracy of bio-plausible machine learning. Code is available at https://github.com/NeuromorphicComputing/SoftHebb.
[ AD11 ]
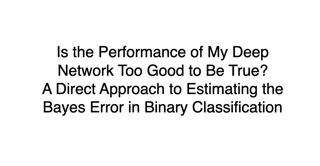
There is a fundamental limitation in the prediction performance that a machine learning model can achieve due to the inevitable uncertainty of the prediction target. In classification problems, this can be characterized by the Bayes error, which is the best achievable error with any classifier. The Bayes error can be used as a criterion to evaluate classifiers with state-of-the-art performance and can be used to detect test set overfitting. We propose a simple and direct Bayes error estimator, where we just take the mean of the labels that show \emph{uncertainty} of the class assignments. Our flexible approach enables us to perform Bayes error estimation even for weakly supervised data. In contrast to others, our method is model-free and even instance-free. Moreover, it has no hyperparameters and gives a more accurate estimate of the Bayes error than several baselines empirically. Experiments using our method suggest that recently proposed deep networks such as the Vision Transformer may have reached, or is about to reach, the Bayes error for benchmark datasets. Finally, we discuss how we can study the inherent difficulty of the acceptance/rejection decision for scientific articles, by estimating the Bayes error of the ICLR papers from 2017 to 2023.
[ AD10 ]
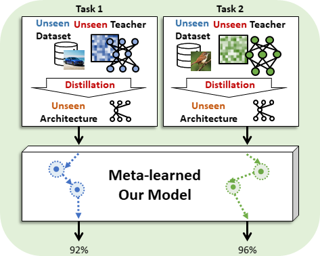
Distillation-aware Network Architecture Search (DaNAS) aims to search for an optimal student architecture that obtains the best performance and/or efficiency when distilling the knowledge from a given teacher model. Previous DaNAS methods have mostly tackled the search for the network architecture for fixed source/target tasks and the teacher, which are not generalized well on a new task, thus need to perform a costly search for any new combination of the domains and the teachers. For standard NAS tasks without KD, meta-learning-based computationally efficient NAS methods have been proposed, which learn the generalized search process over multiple tasks and transfer the knowledge obtained over those tasks to a new task. However, since they assume learning from scratch without KD from a teacher, they might not be ideal for DaNAS scenarios, which could significantly affect the final performances of the architectures obtained from the search. To eliminate the excessive computational cost of DaNAS methods and the sub-optimality of rapid NASmethods, we propose a distillation-aware meta accuracy prediction model, DaSS (Distillation-aware Student Search), which can predict a given architecture’s final performances on a dataset when performing KD with a given teacher, without having actually to train it on the target task. The experimental …
[ Auditorium ]
Partitioning a set of elements into subsets of a priori unknown sizes is essential in many applications. These subset sizes are rarely explicitly learned - be it the cluster sizes in clustering applications or the number of shared versus independent generative latent factors in weakly-supervised learning. Probability distributions over correct combinations of subset sizes are non-differentiable due to hard constraints, which prohibit gradient-based optimization. In this work, we propose the differentiable hypergeometric distribution. The hypergeometric distribution models the probability of different group sizes based on their relative importance. We introduce reparameterizable gradients to learn the importance between groups and highlight the advantage of explicitly learning the size of subsets in two typical applications: weakly-supervised learning and clustering. In both applications, we outperform previous approaches, which rely on suboptimal heuristics to model the unknown size of groups.
[ AD4 ]

Antibody design is valuable for therapeutic usage and biological research. Existing deep-learning-based methods encounter several key issues: 1) incomplete context for Complementarity-Determining Regions (CDRs) generation; 2) incapability of capturing the entire 3D geometry of the input structure; 3) inefficient prediction of the CDR sequences in an autoregressive manner. In this paper, we propose Multi-channel Equivariant Attention Network (MEAN) to co-design 1D sequences and 3D structures of CDRs. To be specific, MEAN formulates antibody design as a conditional graph translation problem by importing extra components including the target antigen and the light chain of the antibody. Then, MEAN resorts to E(3)-equivariant message passing along with a proposed attention mechanism to better capture the geometrical correlation between different components. Finally, it outputs both the 1D sequences and 3D structure via a multi-round progressive full-shot scheme, which enjoys more efficiency and precision against previous autoregressive approaches. Our method significantly surpasses state-of-the-art models in sequence and structure modeling, antigen-binding CDR design, and binding affinity optimization. Specifically, the relative improvement to baselines is about 23\% in antigen-binding CDR design and 34\% for affinity optimization.
[ AD11 ]
The backdoor attack, where the adversary uses inputs stamped with triggers (e.g., a patch) to activate pre-planted malicious behaviors, is a severe threat to Deep Neural Network (DNN) models. Trigger inversion is an effective way of identifying backdoor models and understanding embedded adversarial behaviors. A challenge of trigger inversion is that there are many ways of constructing the trigger. Existing methods cannot generalize to various types of triggers by making certain assumptions or attack-specific constraints. The fundamental reason is that existing work does not formally define the trigger and the inversion problem. This work formally defines and analyzes the trigger and the inversion problem. Then, it proposes a unified framework to invert backdoor triggers based on the formalization of triggers and the identified inner behaviors of backdoor models from our analysis. Our prototype UNICORN is general and effective in inverting backdoor triggers in DNNs. The code can be found at https://github.com/RU-System-Software-and-Security/UNICORN.
[ AD10 ]
Neural Representations have recently been shown to effectively reconstruct a wide range of signals from 3D meshes and shapes to images and videos. We show that, when adapted correctly, neural representations can be used to directly represent the weights of a pre-trained convolutional neural network, resulting in a Neural Representation for Neural Networks (NeRN). Inspired by coordinate inputs of previous neural representation methods, we assign a coordinate to each convolutional kernel in our network based on its position in the architecture, and optimize a predictor network to map coordinates to their corresponding weights. Similarly to the spatial smoothness of visual scenes, we show that incorporating a smoothness constraint over the original network's weights aids NeRN towards a better reconstruction. In addition, since slight perturbations in pre-trained model weights can result in a considerable accuracy loss, we employ techniques from the field of knowledge distillation to stabilize the learning process. We demonstrate the effectiveness of NeRN in reconstructing widely used architectures on CIFAR-10, CIFAR-100, and ImageNet. Finally, we present two applications using NeRN, demonstrating the capabilities of the learned representations.
[ AD12 ]
Inverse reinforcement learning (IRL) methods assume that the expert data is generated by an agent optimizing some reward function. However, in many settings, the agent may optimize a reward function subject to some constraints, where the constraints induce behaviors that may be otherwise difficult to express with just a reward function. We consider the setting where the reward function is given, and the constraints are unknown, and propose a method that is able to recover these constraints satisfactorily from the expert data. While previous work has focused on recovering hard constraints, our method can recover cumulative soft constraints that the agent satisfies on average per episode. In IRL fashion, our method solves this problem by adjusting the constraint function iteratively through a constrained optimization procedure, until the agent behavior matches the expert behavior. We demonstrate our approach on synthetic environments, robotics environments and real world highway driving scenarios.
[ Auditorium ]
Reliable generalization lies at the heart of safe ML and AI. However, understanding when and how neural networks generalize remains one of the most important unsolved problems in the field. In this work, we conduct an extensive empirical study (20'910 models, 15 tasks) to investigate whether insights from the theory of computation can predict the limits of neural network generalization in practice. We demonstrate that grouping tasks according to the Chomsky hierarchy allows us to forecast whether certain architectures will be able to generalize to out-of-distribution inputs. This includes negative results where even extensive amounts of data and training time never lead to any non-trivial generalization, despite models having sufficient capacity to fit the training data perfectly. Our results show that, for our subset of tasks, RNNs and Transformers fail to generalize on non-regular tasks, LSTMs can solve regular and counter-language tasks, and only networks augmented with structured memory (such as a stack or memory tape) can successfully generalize on context-free and context-sensitive tasks.
[ AD1 ]
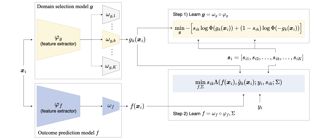
The domain generalization (DG) setup considers the problem where models are trained on data sampled from multiple domains and evaluated on test domains unseen during training. In this paper, we formulate DG as a sample selection problem where each domain is sampled from a common underlying population through non-random sampling probabilities that correlate with both the features and the outcome. Under this setting, the fundamental iid assumption of the empirical risk minimization (ERM) is violated, so it often performs worse on test domains whose non-random sampling probabilities differ from the domains in the training dataset. We propose a Selection-Guided DG (SGDG) framework to learn the selection probability of each domain and the joint distribution of the outcome and domain selection variables. The proposed SGDG is domain generalizable as it intends to minimize the risk under the population distribution. We theoretically proved that, under certain regular conditions, SGDG can achieve smaller risk than ERM. Furthermore, we present a class of parametric SGDG (HeckmanDG) estimators applicable to continuous, binary, and multinomial outcomes. We also demonstrated its efficacy empirically through simulations and experiments on a set of benchmark datasets comparing with other well-known DG methods.
[ AD12 ]
The potential of offline reinforcement learning (RL) is that high-capacity models trained on large, heterogeneous datasets can lead to agents that generalize broadly, analogously to similar advances in vision and NLP. However, recent works argue that offline RL methods encounter unique challenges to scaling up model capacity. Drawing on the learnings from these works, we re-examine previous design choices and find that with appropriate choices: ResNets, cross-entropy based distributional backups, and feature normalization, offline Q-learning algorithms exhibit strong performance that scales with model capacity. Using multi-task Atari as a testbed for scaling and generalization, we train a single policy on 40 games with near-human performance using up-to 80 million parameter networks, finding that model performance scales favorably with capacity. In contrast to prior work, we extrapolate beyond dataset performance even when trained entirely on a large (400M transitions) but highly suboptimal dataset (51% human-level performance). Compared to return-conditioned supervised approaches, offline Q-learning scales similarly with model capacity and has better performance, especially when the dataset is suboptimal. Finally, we show that offline Q-learning with a diverse dataset is sufficient to learn powerful representations that facilitate rapid transfer to novel games and fast online learning on new variations of a training …
[ Auditorium ]
This paper studies learning on text-attributed graphs (TAGs), where each node is associated with a text description. An ideal solution for such a problem would be integrating both the text and graph structure information with large language models and graph neural networks (GNNs). However, the problem becomes very challenging when graphs are large due to the high computational complexity brought by training large language models and GNNs together. In this paper, we propose an efficient and effective solution to learning on large text-attributed graphs by fusing graph structure and language learning with a variational Expectation-Maximization (EM) framework, called GLEM. Instead of simultaneously training large language models and GNNs on big graphs, GLEM proposes to alternatively update the two modules in the E-step and M-step. Such a procedure allows training the two modules separately while simultaneously allowing the two modules to interact and mutually enhance each other. Extensive experiments on multiple data sets demonstrate the efficiency and effectiveness of the proposed approach.
[ AD4 ]
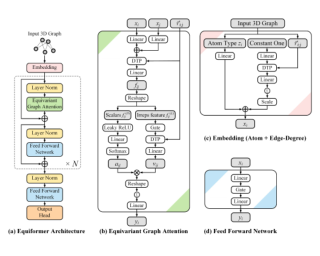
Despite their widespread success in various domains, Transformer networks have yet to perform well across datasets in the domain of 3D atomistic graphs such as molecules even when 3D-related inductive biases like translational invariance and rotational equivariance are considered. In this paper, we demonstrate that Transformers can generalize well to 3D atomistic graphs and present Equiformer, a graph neural network leveraging the strength of Transformer architectures and incorporating SE(3)/E(3)-equivariant features based on irreducible representations (irreps). First, we propose a simple and effective architecture by only replacing original operations in Transformers with their equivariant counterparts and including tensor products. Using equivariant operations enables encoding equivariant information in channels of irreps features without complicating graph structures. With minimal modifications to Transformers, this architecture has already achieved strong empirical results. Second, we propose a novel attention mechanism called equivariant graph attention, which improves upon typical attention in Transformers through replacing dot product attention with multi-layer perceptron attention and including non-linear message passing. With these two innovations, Equiformer achieves competitive results to previous models on QM9, MD17 and OC20 datasets.
[ AD11 ]
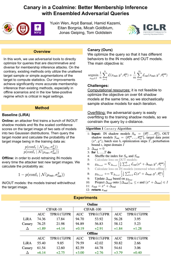
As industrial applications are increasingly automated by machine learning models, enforcing personal data ownership and intellectual property rights requires tracing training data back to their rightful owners. Membership inference algorithms approach this problem by using statistical techniques to discern whether a target sample was included in a model's training set. However, existing methods only utilize the unaltered target sample or simple augmentations of the target to compute statistics. Such a sparse sampling of the model's behavior carries little information, leading to poor inference capabilities. In this work, we use adversarial tools to directly optimize for queries that are discriminative and diverse. Our improvements achieve significantly more accurate membership inference than existing methods, especially in offline scenarios and in the low false-positive regime which is critical in legal settings.
[ AD10 ]
Domain Adaptation of Black-box Predictors (DABP) aims to learn a model on an unlabeled target domain supervised by a black-box predictor trained on a source domain. It does not require access to both the source-domain data and the predictor parameters, thus addressing the data privacy and portability issues of standard domain adaptation methods. Existing DABP approaches mostly rely on knowledge distillation (KD) from the black-box predictor, i.e., training the model with its noisy target-domain predictions, which however inevitably introduces the confirmation bias accumulated from the prediction noises and leads to degrading performance. To mitigate such bias, we propose a new strategy, \textit{divide-to-adapt}, that purifies cross-domain knowledge distillation by proper domain division. This is inspired by an observation we make for the first time in domain adaptation: the target domain usually contains easy-to-adapt and hard-to-adapt samples that have different levels of domain discrepancy w.r.t. the source domain, and deep models tend to fit easy-to-adapt samples first. Leveraging easy-to-adapt samples with less noise can help KD alleviate the negative effect of prediction noises from black-box predictors. In this sense, the target domain can be divided into an easy-to-adapt subdomain with less noise and a hard-to-adapt subdomain at the early stage of training. …
[ AD10 ]
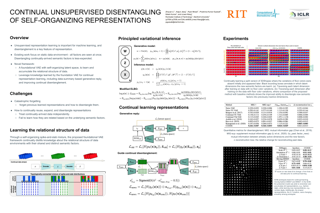
Limited progress has been made in continual unsupervised learning of representations, especially in reusing, expanding, and continually disentangling learned semantic factors across data environments. We argue that this is because existing approaches treat continually-arrived data independently, without considering how they are related based on the underlying semantic factors. We address this by a new generative model describing a topologically-connected mixture of spike-and-slab distributions in the latent space, learned end-to-end in a continual fashion via principled variational inference. The learned mixture is able to automatically discover the active semantic factors underlying each data environment and to accumulate their relational structure based on that. This distilled knowledge of different data environments can further be used for generative replay and guiding continual disentangling of new semantic factors. We tested the presented method on a split version of 3DShapes to provide the first quantitative disentanglement evaluation of continually learned representations, and further demonstrated its ability to continually disentangle new representations in benchmark datasets.
[ AD12 ]
[ AD11 ]
Transformers have become a default architecture in computer vision, but understanding what drives their predictions remains a challenging problem. Current explanation approaches rely on attention values or input gradients, but these provide a limited view of a model’s dependencies. Shapley values offer a theoretically sound alternative, but their computational cost makes them impractical for large, high-dimensional models. In this work, we aim to make Shapley values practical for vision transformers (ViTs). To do so, we first leverage an attention masking approach to evaluate ViTs with partial information, and we then develop a procedure to generate Shapley value explanations via a separate, learned explainer model. Our experiments compare Shapley values to many baseline methods (e.g., attention rollout, GradCAM, LRP), and we find that our approach provides more accurate explanations than existing methods for ViTs.
[ AD4 ]
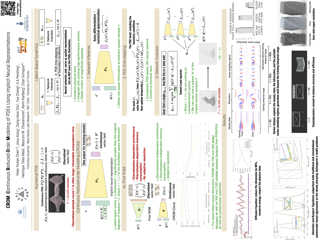
[ AD11 ]

[ AD1 ]
Reliable application of machine learning-based decision systems in the wild is one of the major challenges currently investigated by the field. A large portion of established approaches aims to detect erroneous predictions by means of assigning confidence scores. This confidence may be obtained by either quantifying the model's predictive uncertainty, learning explicit scoring functions, or assessing whether the input is in line with the training distribution. Curiously, while these approaches all state to address the same eventual goal of detecting failures of a classifier upon real-world application, they currently constitute largely separated research fields with individual evaluation protocols, which either exclude a substantial part of relevant methods or ignore large parts of relevant failure sources. In this work, we systematically reveal current pitfalls caused by these inconsistencies and derive requirements for a holistic and realistic evaluation of failure detection. To demonstrate the relevance of this unified perspective, we present a large-scale empirical study for the first time enabling benchmarking confidence scoring functions w.r.t all relevant methods and failure sources. The revelation of a simple softmax response baseline as the overall best performing method underlines the drastic shortcomings of current evaluation in the plethora of publicized research on confidence scoring. Code …
[ AD4 ]
We present a novel neural-networks-based algorithm to compute optimal transport maps and plans for strong and weak transport costs. To justify the usage of neural networks, we prove that they are universal approximators of transport plans between probability distributions. We evaluate the performance of our optimal transport algorithm on toy examples and on the unpaired image-to-image translation.
[ AD12 ]
Diffusion models have been recently studied as powerful generative inverse problem solvers, owing to their high quality reconstructions and the ease of combining existing iterative solvers. However, most works focus on solving simple linear inverse problems in noiseless settings, which significantly under-represents the complexity of real-world problems. In this work, we extend diffusion solvers to efficiently handle general noisy (non)linear inverse problems via the Laplace approximation of the posterior sampling. Interestingly, the resulting posterior sampling scheme is a blended version of diffusion sampling with the manifold constrained gradient without a strict measurement consistency projection step, yielding a more desirable generative path in noisy settings compared to the previous studies. Our method demonstrates that diffusion models can incorporate various measurement noise statistics such as Gaussian and Poisson, and also efficiently handle noisy nonlinear inverse problems such as Fourier phase retrieval and non-uniform deblurring.
[ AD10 ]
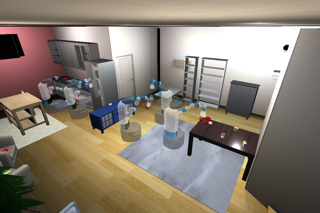
We study a modular approach to tackle long-horizon mobile manipulation tasks for object rearrangement, which decomposes a full task into a sequence of subtasks. To tackle the entire task, prior work chains multiple stationary manipulation skills with a point-goal navigation skill, which are learned individually on subtasks. Although more effective than monolithic end-to-end RL policies, this framework suffers from compounding errors in skill chaining, e.g., navigating to a bad location where a stationary manipulation skill can not reach its target to manipulate. To this end, we propose that the manipulation skills should include mobility to have flexibility in interacting with the target object from multiple locations and at the same time the navigation skill could have multiple end points which lead to successful manipulation. We operationalize these ideas by implementing mobile manipulation skills rather than stationary ones and training a navigation skill trained with region goal instead of point goal. We evaluate our multi-skill mobile manipulation method M3 on 3 challenging long-horizon mobile manipulation tasks in the Home Assistant Benchmark (HAB), and show superior performance as compared to the baselines.
[ AD11 ]
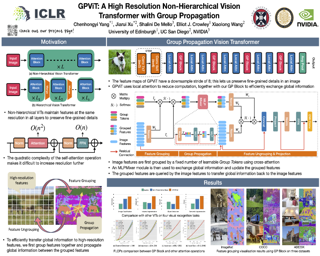
We present the Group Propagation Vision Transformer (GPViT): a novel non- hierarchical (i.e. non-pyramidal) transformer model designed for general visual recognition with high-resolution features. High-resolution features (or tokens) are a natural fit for tasks that involve perceiving fine-grained details such as detection and segmentation, but exchanging global information between these features is expensive in memory and computation because of the way self-attention scales. We provide a highly efficient alternative Group Propagation Block (GP Block) to exchange global information. In each GP Block, features are first grouped to- gether by a fixed number of learnable group tokens; we then perform Group Propagation where global information is exchanged between the grouped fea- tures; finally, global information in the updated grouped features is returned back to the image features through a transformer decoder. We evaluate GPViT on a variety of visual recognition tasks including image classification, semantic seg- mentation, object detection, and instance segmentation. Our method achieves significant performance gains over previous works across all tasks, especially on tasks that require high-resolution outputs, for example, our GPViT-L3 out- performs Swin Transformer-B by 2.0 mIoU on ADE20K semantic segmentation with only half as many parameters. Code and pre-trained models are available at https://github.com/ChenhongyiYang/GPViT.
[ Auditorium ]
[ Auditorium ]
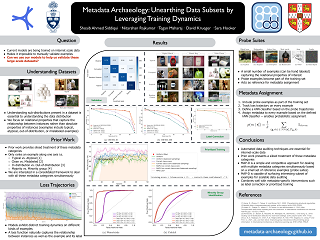
Modern machine learning research relies on relatively few carefully curated datasets. Even in these datasets, and typically in `untidy' or raw data, practitioners are faced with significant issues of data quality and diversity which can be prohibitively labor intensive to address. Existing methods for dealing with these challenges tend to make strong assumptions about the particular issues at play, and often require a priori knowledge or metadata such as domain labels. Our work is orthogonal to these methods: we instead focus on providing a unified and efficient framework for Metadata Archaeology -- uncovering and inferring metadata of examples in a dataset. We curate different subsets of data that might exist in a dataset (e.g. mislabeled, atypical, or out-of-distribution examples) using simple transformations, and leverage differences in learning dynamics between these probe suites to infer metadata of interest. Our method is on par with far more sophisticated mitigation methods across different tasks: identifying and correcting mislabeled examples, classifying minority-group samples, prioritizing points relevant for training and enabling scalable human auditing of relevant examples.
[ AD4 ]
[ AD12 ]
Recent large-scale text-driven synthesis diffusion models have attracted much attention thanks to their remarkable capabilities of generating highly diverse images that follow given text prompts. Therefore, it is only natural to build upon these synthesis models to provide text-driven image editing capabilities. However, Editing is challenging for these generative models, since an innate property of an editing technique is to preserve some content from the original image, while in the text-based models, even a small modification of the text prompt often leads to a completely different outcome. State-of-the-art methods mitigate this by requiring the users to provide a spatial mask to localize the edit, hence, ignoring the original structure and content within the masked region. In this paper, we pursue an intuitive prompt-to-prompt editing framework, where the edits are controlled by text only. We analyze a text-conditioned model in depth and observe that the cross-attention layers are the key to controlling the relation between the spatial layout of the image to each word in the prompt. With this observation, we propose to control the attention maps along the diffusion process. Our approach enables us to monitor the synthesis process by editing the textual prompt only, paving the way to a …
[ AD10 ]

Extensive work has demonstrated that equivariant neural networks can significantly improve sample efficiency and generalization by enforcing an inductive bias in the network architecture. These applications typically assume that the domain symmetry is fully described by explicit transformations of the model inputs and outputs. However, many real-life applications contain only latent or partial symmetries which cannot be easily described by simple transformations of the input. In these cases, it is necessary to learn symmetry in the environment instead of imposing it mathematically on the network architecture. We discover, surprisingly, that imposing equivariance constraints that do not exactly match the domain symmetry is very helpful in learning the true symmetry in the environment. We differentiate between extrinsic and incorrect symmetry constraints and show that while imposing incorrect symmetry can impede the model's performance, imposing extrinsic symmetry can actually improve performance. We demonstrate that an equivariant model can significantly outperform non-equivariant methods on domains with latent symmetries both in supervised learning and in reinforcement learning for robotic manipulation and control problems.
[ AD1 ]
[ AD4 ]
[ AD10 ]

The rise of generalist large-scale models in natural language and vision has made us expect that a massive data-driven approach could achieve broader generalization in other domains such as continuous control. In this work, we explore a method for learning a single policy that manipulates various forms of agents to solve various tasks by distilling a large amount of proficient behavioral data. In order to align input-output (IO) interface among multiple tasks and diverse agent morphologies while preserving essential 3D geometric relations, we introduce morphology-task graph, which treats observations, actions and goals/task in a unified graph representation. We also develop MxT-Bench for fast large-scale behavior generation, which supports procedural generation of diverse morphology-task combinations with a minimal blueprint and hardware-accelerated simulator. Through efficient representation and architecture selection on MxT-Bench, we find out that a morphology-task graph representation coupled with Transformer architecture improves the multi-task performances compared to other baselines including recent discrete tokenization, and provides better prior knowledge for zero-shot transfer or sample efficiency in downstream multi-task imitation learning. Our work suggests large diverse offline datasets, unified IO representation, and policy representation and architecture selection through supervised learning form a promising approach for studying and advancing morphology-task generalization.
[ AD12 ]
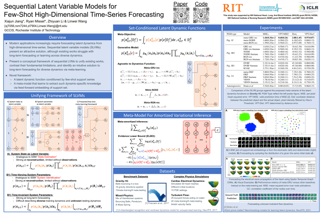
Modern applications increasingly require learning and forecasting latent dynamics from high-dimensional time-series. Compared to univariate time-series forecasting, this adds a new challenge of reasoning about the latent dynamics of an unobserved abstract state. Sequential latent variable models (LVMs) present an attractive solution, although existing works either struggle with long-term forecasting or have difficulty learning across diverse dynamics. In this paper, we first present a conceptual framework of sequential LVMs to unify existing works, contrast their fundamental limitations, and identify an intuitive solution to long-term forecasting for diverse dynamics via meta-learning. We then present the first framework of few-shot forecasting for high-dimensional time-series: instead of learning a single dynamic function, we leverage data of diverse dynamics and learn to adapt latent dynamic functions to few-shot support series. This is realized via Bayesian meta-learning underpinned by: 1) a latent dynamic function conditioned on knowledge derived from few-shot support series, and 2) a meta-model that learns to extract such dynamic-specific knowledge via feed-forward embedding of support set. We compared the presented framework with a comprehensive set of baseline models trained 1) globally on the large meta-training set with diverse dynamics, and 2) individually on single dynamics, both with and without fine-tuning to k-shot …
[ Auditorium ]
Motivated by various practical applications, we propose a novel and general formulation of targeted multi-objective hyperparameter optimization. Our formulation allows a clear specification of an automatable optimization goal using lexicographic preference over multiple objectives. We then propose a randomized directed search method named LexiFlow to solve this problem. We demonstrate the strong empirical performance of the proposed algorithm in multiple hyperparameter optimization tasks.
[ AD1 ]

[ AD11 ]
[ AD1 ]
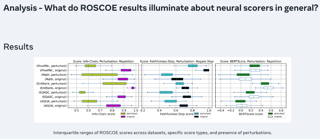
Large language models show improved downstream task performance when prompted to generate step-by-step reasoning to justify their final answers. These reasoning steps greatly improve model interpretability and verification, but objectively studying their correctness (independent of the final answer) is difficult without reliable methods for automatic evaluation. We simply do not know how often the stated reasoning steps actually support the final end task predictions. In this work, we present ROSCOE, a suite of interpretable, unsupervised automatic scores that improve and extend previous text generation evaluation metrics. To evaluate ROSCOE against baseline metrics, we design a typology of reasoning errors and collect synthetic and human evaluation scores on commonly used reasoning datasets. In contrast with existing metrics, ROSCOE can measure semantic consistency, logicality, informativeness, fluency, and factuality — among other traits — by leveraging properties of step-by-step rationales. We empirically verify the strength of our metrics on five human annotated and six programmatically perturbed diagnostics datasets - covering a diverse set of tasks that require reasoning skills and show that ROSCOE can consistently outperform baseline metrics.
[ AD12 ]
Diffusion models achieve outstanding generative performance in various domains. Despite their great success, they lack semantic latent space which is essential for controlling the generative process. To address the problem, we propose asymmetric reverse process (Asyrp) which discovers the semantic latent space in frozen pretrained diffusion models. Our semantic latent space, named h-space, has nice properties for accommodating semantic image manipulation: homogeneity, linearity, robustness, and consistency across timesteps. In addition, we measure editing strength and quality deficiency of a generative process at timesteps to provide a principled design of the process for versatility and quality improvements. Our method is applicable to various architectures (DDPM++, iDDPM, and ADM) and datasets (CelebA-HQ, AFHQ-dog, LSUN-church, LSUN-bedroom, and METFACES).
[ AD11 ]

Natural and expressive human motion generation is the holy grail of computer animation.It is a challenging task, due to the diversity of possible motion, human perceptual sensitivity to it, and the difficulty of accurately describing it. Therefore, current generative solutions are either low-quality or limited in expressiveness. Diffusion models are promising candidates for the human motion domain since theyhave already shown remarkable generative capabilities in other domains, and their many-to-many nature. In this paper, we introduce Motion Diffusion Model (MDM), a carefully adapted classifier-free diffusion-based generative model for human motion data. MDM is transformer-based, combining insights from motion generation literature. A notable design-choice is that it predicts the sample itself rather than the noise in each step to facilitate the use of established geometric losses on the locations and velocities of the motion, such as the foot contact loss. As we demonstrate, MDM is a generic approach, enabling different modes of conditioning, and different generation tasks. We show that our model is trained with lightweight resources and yet achieves state-of-the-art results on leading benchmarks for text-to-motion, action-to-motion, and unconditioned motion generation.
[ AD10 ]
Increasing the replay ratio, the number of updates of an agent's parameters per environment interaction, is an appealing strategy for improving the sample efficiency of deep reinforcement learning algorithms. In this work, we show that fully or partially resetting the parameters of deep reinforcement learning agents causes better replay ratio scaling capabilities to emerge. We push the limits of the sample efficiency of carefully-modified algorithms by training them using an order of magnitude more updates than usual, significantly improving their performance in the Atari 100k and DeepMind Control Suite benchmarks. We then provide an analysis of the design choices required for favorable replay ratio scaling to be possible and discuss inherent limits and tradeoffs.
[ AD4 ]
Content creators compete for user attention. Their reach crucially depends on algorithmic choices made by developers on online platforms. To maximize exposure, many creators adapt strategically, as evidenced by examples like the sprawling search engine optimization industry. This begets competition for the finite user attention pool. We formalize these dynamics in what we call an exposure game, a model of incentives induced by modern algorithms including factorization and (deep) two-tower architectures. We prove that seemingly innocuous algorithmic choices—e.g., non-negative vs. unconstrained factorization—significantly affect the existence and character of (Nash) equilibria in exposure games. We proffer use of creator behavior models like ours for an (ex-ante) pre-deployment audit. Such an audit can identify misalignment between desirable and incentivized content, and thus complement post-hoc measures like content filtering and moderation. To this end, we propose tools for numerically finding equilibria in exposure games, and illustrate results of an audit on the MovieLens and LastFM datasets. Among else, we find that the strategically produced content exhibits strong dependence between algorithmic exploration and content diversity, and between model expressivity and bias towards gender-based user and creator groups.
[ AD10 ]
One of the grand challenges of reinforcement learning is the ability to generalize to new tasks. However, general agents require a set of rich, diverse tasks to train on. Designing a `foundation environment' for such tasks is tricky -- the ideal environment would support a range of emergent phenomena, an expressive task space, and fast runtime. To take a step towards addressing this research bottleneck, this work presents Powderworld, a lightweight yet expressive simulation environment running directly on the GPU. Within Powderworld, two motivating task distributions are presented, one for world-modelling and one for reinforcement learning. Each contains hand-designed test tasks to examine generalization. Experiments indicate that increasing the environment's complexity improves generalization for world models, yet causes reinforcement learning agents to struggle. Powderworld aims to support the study of generalization by providing a source of diverse tasks arising from the same core rules.
[ Auditorium ]
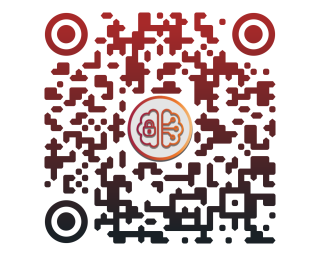
[ AD12 ]
Recent breakthroughs in text-to-image synthesis have been driven by diffusion models trained on billions of image-text pairs. Adapting this approach to 3D synthesis would require large-scale datasets of labeled 3D or multiview data and efficient architectures for denoising 3D data, neither of which currently exist. In this work, we circumvent these limitations by using a pretrained 2D text-to-image diffusion model to perform text-to-3D synthesis. We introduce a loss based on probability density distillation that enables the use of a 2D diffusion model as a prior for optimization of a parametric image generator. Using this loss in a DeepDream-like procedure, we optimize a randomly-initialized 3D model (a Neural Radiance Field, or NeRF) via gradient descent such that its 2D renderings from random angles achieve a low loss. The resulting 3D model of the given text can be viewed from any angle, relit by arbitrary illumination, or composited into any 3D environment. Our approach requires no 3D training data and no modifications to the image diffusion model, demonstrating the effectiveness of pretrained image diffusion models as priors.
[ AD1 ]
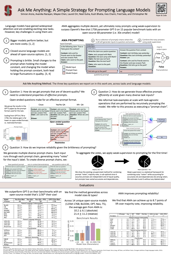
Large language models (LLMs) transfer well to new tasks out-of-the-box simply given a natural language prompt that demonstrates how to perform the task and no additional training. Prompting is a brittle process wherein small modifications to the prompt can cause large variations in the model predictions, and therefore significant effort is dedicated towards designing a painstakingly crafted "perfect prompt" for a task. To mitigate the high degree of effort, we instead ask whether collecting multiple decent, yet imperfect, prompts and aggregating them can lead to a high quality prompting strategy. Our observations motivate our proposed method, Ask Me Anything (AMA). We first develop an understanding of the effective prompt formats, finding question-answering (QA) prompts, which encourage open-ended generation ("Who went to the park?") tend to outperform those that restrict the model outputs ("John went to the park. True or False?"). AMA recursively uses the LLM to transform task inputs to the effective QA format. AM generates multiple questions per input and applies these prompts to collect several noisy "votes" for the input's true label. We find the prompts have varying accuracies and dependencies and thus propose to use weak supervision, a procedure for combining the noisy predictions, to produce the …
[ AD11 ]

Neural Motion Planners (NMPs) have emerged as a promising tool for solving robot navigation tasks in complex environments. However, these methods often require expert data for learning, which limits their application to scenarios where data generation is time-consuming. Recent developments have also led to physics-informed deep neural models capable of representing complex dynamical Partial Differential Equations (PDEs). Inspired by these developments, we propose Neural Time Fields (NTFields) for robot motion planning in cluttered scenarios. Our framework represents a wave propagation model generating continuous arrival time to find path solutions informed by a nonlinear first-order PDE called Eikonal Equation. We evaluate our method in various cluttered 3D environments, including the Gibson dataset, and demonstrate its ability to solve motion planning problems for 4-DOF and 6-DOF robot manipulators where the traditional grid-based Eikonal planners often face the curse of dimensionality. Furthermore, the results show that our method exhibits high success rates and significantly lower computational times than the state-of-the-art methods, including NMPs that require training data from classical planners.
[ AD12 ]
[ AD10 ]
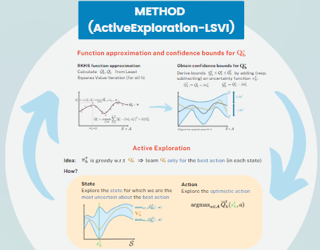
Many real-world reinforcement learning tasks require control of complex dynamical systems that involve both costly data acquisition processes and large state spaces. In cases where the expensive transition dynamics can be readily evaluated at specified states (e.g., via a simulator), agents can operate in what is often referred to as planning with a \emph{generative model}. We propose the AE-LSVI algorithm for best policy identification, a novel variant of the kernelized least-squares value iteration (LSVI) algorithm that combines optimism with pessimism for active exploration (AE). AE-LSVI provably identifies a near-optimal policy \emph{uniformly} over an entire state space and achieves polynomial sample complexity guarantees that are independent of the number of states. When specialized to the recently introduced offline contextual Bayesian optimization setting, our algorithm achieves improved sample complexity bounds. Experimentally, we demonstrate that AE-LSVI outperforms other RL algorithms in a variety of environments when robustness to the initial state is required.
[ AD4 ]
With the advent of large datasets, offline reinforcement learning is a promising framework for learning good decision-making policies without the need to interact with the real environment.However, offline RL requires the dataset to be reward-annotated, which presents practical challenges when reward engineering is difficult or when obtaining reward annotations is labor-intensive.In this paper, we introduce Optimal Transport Relabeling (OTR), an imitation learning algorithm that can automatically relabel offline data of mixed and unknown quality with rewards from a few good demonstrations. OTR's key idea is to use optimal transport to compute an optimal alignment between an unlabeled trajectory in the dataset and an expert demonstration to obtain a similarity measure that can be interpreted as a reward, which can then be used by an offline RL algorithm to learn the policy. OTR is easy to implement and computationally efficient. On D4RL benchmarks, we demonstrate that OTR with a single demonstration can consistently match the performance of offline RL with ground-truth rewards.
[ AD1 ]
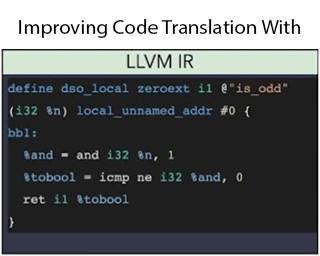
In this paper, we leverage low-level compiler intermediate representations (IR) code translation. Traditional transpilers rely on syntactic information and handcrafted rules, which limits their applicability and produces unnatural-looking code. Applying neural machine translation (NMT) approaches to code has successfully broadened the set of programs on which one can get a natural-looking translation. However, they treat the code as sequences of text tokens, and still do not differentiate well enough between similar pieces of code which have different semantics in different languages. The consequence is low quality translation, reducing the practicality of NMT, and stressing the need for an approach significantly increasing its accuracy. Here we propose to augment code translation with IRs, specifically LLVM IR, with results on the C++, Java, Rust, and Go languages. Our method improves upon the state of the art for unsupervised code translation, increasing the number of correct translations by 11% on average, and up to 79% for the Java → Rust pair with greedy decoding. With beam search, it increases the number of correct translations by 5.5% in average. We extend previous test sets for code translation, by adding hundreds of Go and Rust functions. Additionally, we train models with high performance on the …
[ AD11 ]
We propose Unified-IO, a model that performs a large variety of AI tasks spanning classical computer vision tasks, including pose estimation, object detection, depth estimation and image generation, vision-and-language tasks such as region captioning and referring expression, to natural language processing tasks such as question answering and paraphrasing. Developing a single unified model for such a large variety of tasks poses unique challenges due to the heterogeneous inputs and outputs pertaining to each task, including RGB images, per-pixel maps, binary masks, bounding boxes, and language. We achieve this unification by homogenizing every supported input and output into a sequence of discrete vocabulary tokens. This common representation across all tasks allows us to train a single transformer-based architecture, jointly on over 90 diverse datasets in the vision and language fields. Unified-IO is the first model capable of performing all 7 tasks on the GRIT benchmark and produces strong results across 16 diverse benchmarks like NYUv2-Depth, ImageNet, VQA2.0, OK-VQA, Swig, VizWizGround, BoolQ, and SciTail, with no task-specific fine-tuning. Code and pre-trained models will be made publicly available.
[ Auditorium ]
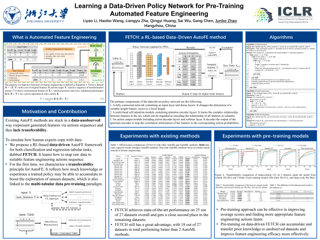
Feature engineering is widely acknowledged to be pivotal in tabular data analysis and prediction. Automated feature engineering (AutoFE) emerged to automate this process managed by experienced data scientists and engineers conventionally. In this area, most — if not all — prior work adopted an identical framework from the neural architecture search (NAS) method. While feasible, we posit that the NAS framework very much contradicts the way how human experts cope with the data since the inherent Markov decision process (MDP) setup differs. We point out that its data-unobserved setup consequentially results in an incapability to generalize across different datasets as well as also high computational cost. This paper proposes a novel AutoFE framework Feature Set Data-Driven Search (FETCH), a pipeline mainly for feature generation and selection. Notably, FETCH is built on a brand-new data-driven MDP setup using the tabular dataset as the state fed into the policy network. Further, we posit that the crucial merit of FETCH is its transferability where the yielded policy network trained on a variety of datasets is indeed capable to enact feature engineering on unseen data, without requiring additional exploration. To the best of our knowledge, this is a pioneer attempt to build a tabular …
[ AD11 ]
Recent work has shown exciting promise in updating large language models with new memories, so as to replace obsolete information or add specialized knowledge. However, this line of work is predominantly limited to updating single associations. We develop MEMIT, a method for directly updating a language model with many memories, demonstrating experimentally that it can scale up to thousands of associations for GPT-J (6B) and GPT-NeoX (20B), exceeding prior work by an order of magnitude. Our code and data will be open-sourced upon publication.
[ AD10 ]
How well do reward functions learned with inverse reinforcement learning (IRL) generalize? We illustrate that state-of-the-art IRL algorithms, which maximize a maximum-entropy objective, learn rewards that overfit to the demonstrations. Such rewards struggle to provide meaningful rewards for states not covered by the demonstrations, a major detriment when using the reward to learn policies in new situations. We introduce BC-IRL a new inverse reinforcement learning method that learns reward functions that generalize better when compared to maximum-entropy IRL approaches. In contrast to the MaxEnt framework, which learns to maximize rewards around demonstrations, BC-IRL updates reward parameters such that the policy trained with the new reward matches the expert demonstrations better. We show that BC-IRL learns rewards that generalize better on an illustrative simple task and two continuous robotic control tasks, achieving over twice the success rate of baselines in challenging generalization settings.
[ AD1 ]
Simultaneous machine translation (SiMT) outputs the target sequence while receiving the source sequence, and hence learning when to start translating each target token is the core challenge for SiMT task. However, it is non-trivial to learn the optimal moment among many possible moments of starting translating, as the moments of starting translating always hide inside the model and can only be supervised with the observed target sequence. In this paper, we propose a Hidden Markov Transformer (HMT), which treats the moments of starting translating as hidden events and the target sequence as the corresponding observed events, thereby organizing them as a hidden Markov model. HMT explicitly models multiple moments of starting translating as the candidate hidden events, and then selects one to generate the target token. During training, by maximizing the marginal likelihood of the target sequence over multiple moments of starting translating, HMT learns to start translating at the moments that target tokens can be generated more accurately. Experiments on multiple SiMT benchmarks show that HMT outperforms strong baselines and achieves state-of-the-art performance.
[ Auditorium ]
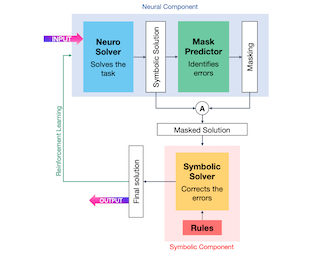
The integration of hard constraints on neural network outputs is a very desirable capability. This allows to instill trust in AI by guaranteeing the sanity of that neural network predictions with respect to domain knowledge. Recently, this topic has received a lot of attention. However, all the existing methods usually either impose the constraints in a "weak" form at training time, with no guarantees at inference, or fail to provide a general framework that supports different tasks and constraint types. We tackle this open problem from a neuro-symbolic perspective. Our pipeline enhances a conventional neural predictor with (1) a symbolic reasoning module capable of correcting structured prediction errors and (2) a neural attention module that learns to direct the reasoning effort to focus on potential prediction errors, while keeping other outputs unchanged. This framework provides an appealing trade-off between the efficiency of constraint-free neural inference and the prohibitive cost of exhaustive reasoning at inference time. We show that our method outperforms the state of the art on visual-Sudoku, and can also benefit visual scene graph prediction. Furthermore, it can improve the performance of existing neuro-symbolic systems that lack our explicit reasoning during inference.
[ AD10 ]
Many important tasks involve some notion of long-term progress in multiple phases: e.g. to clean a shelf it must be cleared of items, cleaning products applied, and then the items placed back on the shelf. In this work, we explore the use of expert demonstrations in long-horizon tasks to learn a monotonically increasing function that summarizes progress. This function can then be used to aid agent exploration in environments with sparse rewards. As a case study we consider the NetHack environment, which requires long-term progress at a variety of scales and is far from being solved by existing approaches. In this environment, we demonstrate that by learning a model of long-term progress from expert data containing only observations, we can achieve efficient exploration in challenging sparse tasks, well beyond what is possible with current state-of-the-art approaches. We have made the curated gameplay dataset used in this work available at https://github.com/deepmind/nao_top10.
[ AD11 ]
A good automatic evaluation metric for language generation ideally correlates highly with human judgements of text quality. Yet, there is a dearth of such metrics, which inhibits the rapid and efficient progress of language generators. One exception is the recently proposed Mauve. In theory, Mauve measures an information-theoretic divergence between two probability distributions over strings: one representing the language generator under evaluation; the other representing the true natural language distribution. Mauve's authors argue that its success comes from the qualitative properties of their proposed divergence. Yet in practice, as this divergence is uncomputable, Mauve approximates it by measuring the divergence between multinomial distributions over clusters instead, where cluster assignments are attained by grouping strings based on a pretrained language model's embeddings. As we show, however, this is not a tight approximation---in either theory or practice. This begs the question: why does Mauve work so well? In this work, we show that \mauve was right for the wrong reasons, and that its newly proposed divergence is not necessary for its high performance. In fact, classical divergences paired with its proposed cluster-based approximation may actually serve as better evaluation metrics. We finish the paper with a probing analysis; this analysis leads us …
[ AD1 ]
Large language models (LLMs) have been shown to be capable of impressive few-shot generalisation to new tasks. However, they still tend to perform poorly on multi-step logical reasoning problems. Here we carry out a comprehensive evaluation of LLMs on 46 tasks that probe different aspects of logical reasoning. We show that language models tend to perform fairly well at single step inference or entailment tasks, but struggle to chain together multiple reasoning steps to solve more complex problems. In light of this, we propose a Selection-Inference (SI) framework that exploits pre-trained LLMs as general processing modules, and alternates between selection and inference to generate a series of interpretable, casual reasoning steps leading to the final answer. We show that a 7B parameter LLM used within the SI framework in a 5-shot generalisation setting, with no fine-tuning, yields a performance improvement of over 100% compared to an equivalent vanilla baseline on a suite of 10 logical reasoning tasks. The same model in the same setting even outperforms a significantly larger 280B parameter baseline on the same suite of tasks. Moreover, answers produced by the SI framework are accompanied by a causal natural-language-based reasoning trace, which has important implications for the safety …
[ AD11 ]
Recent approaches in self-supervised learning of image representations can be categorized into different families of methods and, in particular, can be divided into contrastive and non-contrastive approaches. While differences between the two families have been thoroughly discussed to motivate new approaches, we focus more on the theoretical similarities between them. By designing contrastive and covariance based non-contrastive criteria that can be related algebraically and shown to be equivalent under limited assumptions, we show how close those families can be. We further study popular methods and introduce variations of them, allowing us to relate this theoretical result to current practices and show the influence (or lack thereof) of design choices on downstream performance. Motivated by our equivalence result, we investigate the low performance of SimCLR and show how it can match VICReg's with careful hyperparameter tuning, improving significantly over known baselines. We also challenge the popular assumption that non-contrastive methods need large output dimensions. Our theoretical and quantitative results suggest that the numerical gaps between contrastive and non-contrastive methods in certain regimes can be closed given better network design choices and hyperparameter tuning. The evidence shows that unifying different SOTA methods is an important direction to build a better understanding of …
[ AD12 ]
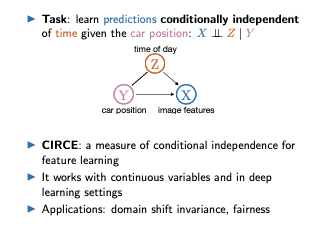
[ AD1 ]
We introduce SignNet and BasisNet---new neural architectures that are invariant to two key symmetries displayed by eigenvectors: (i) sign flips, since if v is an eigenvector then so is -v; and (ii) more general basis symmetries, which occur in higher dimensional eigenspaces with infinitely many choices of basis eigenvectors. We prove that under certain conditions our networks are universal, i.e., they can approximate any continuous function of eigenvectors with the desired invariances. When used with Laplacian eigenvectors, our networks are provably more expressive than existing spectral methods on graphs; for instance, they subsume all spectral graph convolutions, certain spectral graph invariants, and previously proposed graph positional encodings as special cases. Experiments show that our networks significantly outperform existing baselines on molecular graph regression, learning expressive graph representations, and learning neural fields on triangle meshes. Our code is available at https://github.com/cptq/SignNet-BasisNet.
[ AD10 ]

[ Auditorium ]
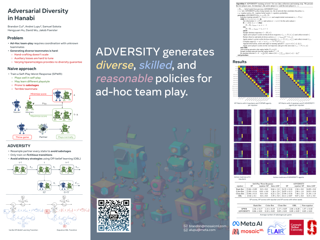
Many Dec-POMDPs admit a qualitatively diverse set of ''reasonable'' joint policies, where reasonableness is indicated by symmetry equivariance, non-sabotaging behaviour and the graceful degradation of performance when paired with ad-hoc partners. Some of the work in diversity literature is concerned with generating these policies. Unfortunately, existing methods fail to produce teams of agents that are simultaneously diverse, high performing, and reasonable. In this work, we propose a novel approach, adversarial diversity (ADVERSITY), which is designed for turn-based Dec-POMDPs with public actions. ADVERSITY relies on off-belief learning to encourage reasonableness and skill, and on ''repulsive'' fictitious transitions to encourage diversity. We use this approach to generate new agents with distinct but reasonable play styles for the card game Hanabi and open-source our agents to be used for future research on (ad-hoc) coordination.
[ AD1 ]
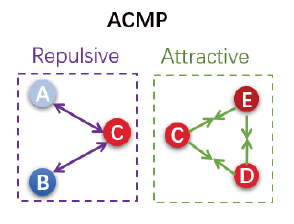
Neural message passing is a basic feature extraction unit for graph-structured data considering neighboring node features in network propagation from one layer to the next. We model such process by an interacting particle system with attractive and repulsive forces and the Allen-Cahn force arising in the modeling of phase transition. The dynamics of the system is a reaction-diffusion process which can separate particles without blowing up. This induces an Allen-Cahn message passing (ACMP) for graph neural networks where the numerical iteration for the particle system solution constitutes the message passing propagation. ACMP which has a simple implementation with a neural ODE solver can propel the network depth up to one hundred of layers with theoretically proven strictly positive lower bound of the Dirichlet energy. It thus provides a deep model of GNNs circumventing the common GNN problem of oversmoothing. GNNs with ACMP achieve state of the art performance for real-world node classification tasks on both homophilic and heterophilic datasets. Codes are available at https://github.com/ykiiiiii/ACMP
[ Auditorium ]
A common assumption when training embodied agents is that the impact of taking an action is stable; for instance, executing the move ahead'' action will always move the agent forward by a fixed distance, perhaps with some small amount of actuator-induced noise. This assumption is limiting; an agent may encounter settings that dramatically alter the impact of actions: a move ahead action on a wet floor may send the agent twice as far as it expects and using the same action with a broken wheel might transform the expected translation into a rotation. Instead of relying that the impact of an action stably reflects its pre-defined semantic meaning, we propose to model the impact of actions on-the-fly using latent embeddings. By combining these latent action embeddings with a novel, transformer-based, policy head, we design an Action Adaptive Policy (AAP). We evaluate our AAP on two challenging visual navigation tasks in the AI2-THOR and Habitat environments and show that our AAP is highly performant even when faced, at inference-time, with missing actions and, previously unseen, perturbed action spaces. Moreover, we observe significant improvement in robustness against these actions when evaluating in real-world scenarios.
[ AD11 ]
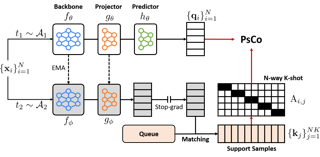
Unsupervised meta-learning aims to learn generalizable knowledge across a distribution of tasks constructed from unlabeled data. Here, the main challenge is how to construct diverse tasks for meta-learning without label information; recent works have proposed to create, e.g., pseudo-labeling via pretrained representations or creating synthetic samples via generative models. However, such a task construction strategy is fundamentally limited due to heavy reliance on the immutable pseudo-labels during meta-learning and the quality of the representations or the generated samples. To overcome the limitations, we propose a simple yet effective unsupervised meta-learning framework, coined Pseudo-supervised Contrast (PsCo), for few-shot classification. We are inspired by the recent self-supervised learning literature; PsCo utilizes a momentum network and a queue of previous batches to improve pseudo-labeling and construct diverse tasks in a progressive manner. Our extensive experiments demonstrate that PsCo outperforms existing unsupervised meta-learning methods under various in-domain and cross-domain few-shot classification benchmarks. We also validate that PsCo is easily scalable to a large-scale benchmark, while recent prior-art meta-schemes are not.
[ AD12 ]
[ AD10 ]
[ AD10 ]
[ AD11 ]

Pre-training representations (a.k.a. foundation models) has recently become a prevalent learning paradigm, where one first pre-trains a representation using large-scale unlabeled data, and then learns simple predictors on top of the representation using small labeled data from the downstream tasks. There are two key desiderata for the representation: label efficiency (the ability to learn an accurate classifier on top of the representation with a small amount of labeled data) and universality (usefulness across a wide range of downstream tasks). In this paper, we focus on one of the most popular instantiations of this paradigm: contrastive learning with linear probing, i.e., learning a linear predictor on the representation pre-trained by contrastive learning. We show that there exists a trade-off between the two desiderata so that one may not be able to achieve both simultaneously. Specifically, we provide analysis using a theoretical data model and show that, while more diverse pre-training data result in more diverse features for different tasks (improving universality), it puts less emphasis on task-specific features, giving rise to larger sample complexity for down-stream supervised tasks, and thus worse prediction performance. Guided by this analysis, we propose a contrastive regularization method to improve the trade-off. We validate our analysis …
[ Auditorium ]
Robots operating in the real world require both rich manipulation skills as well as the ability to semantically reason about when to apply those skills. Towards this goal, recent works have integrated semantic representations from large-scale pretrained vision-language (VL) models into manipulation models, imparting them with more general reasoning capabilities. However, we show that the conventional {\it pretraining-finetuning} pipeline for integrating such representations entangles the learning of domain-specific action information and domain-general visual information, leading to less data-efficient training and poor generalization to unseen objects and tasks. To this end, we propose \ours, a {\it modular} approach to better leverage pretrained VL models by exploiting the syntactic and semantic structures of language instructions. Our framework uses a semantic parser to recover an executable program, composed of functional modules grounded on vision and action across different modalities. Each functional module is realized as a combination of deterministic computation and learnable neural networks. Program execution produces parameters to general manipulation primitives for a robotic end-effector. The entire modular network can be trained with end-to-end imitation learning objectives. Experiments show that our model successfully disentangles action and perception, translating to improved zero-shot and compositional generalization in a variety of manipulation behaviors. Project webpage …
[ AD1 ]
Language models show a surprising range of capabilities, but the source of their apparent competence is unclear. Do these networks just memorize a collection of surface statistics, or do they rely on internal representations of the process that generates the sequences they see? We investigate this question by applying a variant of the GPT model to the task of predicting legal moves in a simple board game, Othello. Although the network has no a priori knowledge of the game or its rules, we uncover evidence of an emergent nonlinear internal representation of the board state. Interventional experiments indicate this representation can be used to control the output of the network and create "latent saliency maps" that can help explain predictions in human terms.
[ AD12 ]
Grokking, the unusual phenomenon for algorithmic datasets where generalization happens long after overfitting the training data, has remained elusive. We aim to understand grokking by analyzing the loss landscapes of neural networks, identifying the mismatch between training and test losses as the cause for grokking. We refer to this as the "LU mechanism" because training and test losses (against model weight norm) typically resemble "L" and "U", respectively. This simple mechanism can nicely explain many aspects of grokking: data size dependence, weight decay dependence, the emergence of representations, etc. Guided by the intuitive picture, we are able to induce grokking on tasks involving images, language and molecules, although the grokking signals are sometimes less dramatic. We attribute the dramatic nature of grokking for algorithmic datasets to representation learning.
[ AD10 ]
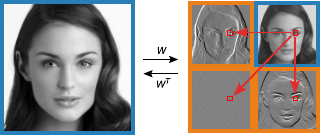
Deep neural networks can learn powerful prior probability models for images, as evidenced by the high-quality generations obtained with recent score-based diffusion methods. But the means by which these networks capture complex global statistical structure, apparently without suffering from the curse of dimensionality, remain a mystery. To study this, we incorporate diffusion methods into a multi-scale decomposition, reducing dimensionality by assuming a stationary local Markov model for wavelet coefficients conditioned on coarser-scale coefficients. We instantiate this model using convolutional neural networks (CNNs) with local receptive fields, which enforce both the stationarity and Markov properties. Global structures are captured using a CNN with receptive fields covering the entire (but small) low-pass image. We test this model on a dataset of face images, which are highly non-stationary and contain large-scale geometric structures.Remarkably, denoising, super-resolution, and image synthesis results all demonstrate that these structures can be captured with significantly smaller conditioning neighborhoods than required by a Markov model implemented in the pixel domain. Our results show that score estimation for large complex images can be reduced to low-dimensional Markov conditional models across scales, alleviating the curse of dimensionality.
[ AD1 ]
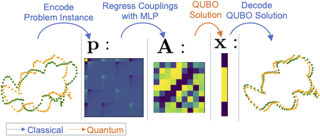
Modern quantum annealers can find high-quality solutions to combinatorial optimisation objectives given as quadratic unconstrained binary optimisation (QUBO) problems. Unfortunately, obtaining suitable QUBO forms in computer vision remains challenging and currently requires problem-specific analytical derivations. Moreover, such explicit formulations impose tangible constraints on solution encodings. In stark contrast to prior work, this paper proposes to learn QUBO forms from data through gradient backpropagation instead of deriving them. As a result, the solution encodings can be chosen flexibly and compactly. Furthermore, our methodology is general and virtually independent of the specifics of the target problem type. We demonstrate the advantages of learnt QUBOs on the diverse problem types of graph matching, 2D point cloud alignment and 3D rotation estimation. Our results are competitive with the previous quantum state of the art while requiring much fewer logical and physical qubits, enabling our method to scale to larger problems. The code and the new dataset are available at https://4dqv.mpi-inf.mpg.de/QuAnt/.
[ Auditorium ]
Inferring reward functions from human behavior is at the center of value alignment – aligning AI objectives with what we, humans, actually want. But doing so relies on models of how humans behave given their objectives. After decades of research in cognitive science, neuroscience, and behavioral economics, obtaining accurate human models remains an open research topic. This begs the question: how accurate do these models need to be in order for the reward inference to be accurate? On the one hand, if small errors in the model can lead to catastrophic error in inference, the entire framework of reward learning seems ill-fated, as we will never have perfect models of human behavior. On the other hand, if as our models improve, we can have a guarantee that reward accuracy also improves, this would show the benefit of more work on the modeling side. We study this question both theoretically and empirically. We do show that it is unfortunately possible to construct small adversarial biases in behavior that lead to arbitrarily large errors in the inferred reward. However, and arguably more importantly, we are also able to identify reasonable assumptions under which the reward inference error can be bounded linearly in …
[ AD11 ]
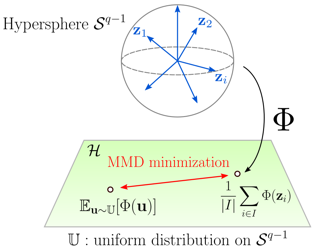
We introduce a regularization loss based on kernel mean embeddings with rotation-invariant kernels on the hypersphere (also known as dot-product kernels) for self-supervised learning of image representations. Besides being fully competitive with the state of the art, our method significantly reduces time and memory complexity for self-supervised training, making it implementable for very large embedding dimensions on existing devices and more easily adjustable than previous methods to settings with limited resources. Our work follows the major paradigm where the model learns to be invariant to some predefined image transformations (cropping, blurring, color jittering, etc.), while avoiding a degenerate solution by regularizing the embedding distribution. Our particular contribution is to propose a loss family promoting the embedding distribution to be close to the uniform distribution on the hypersphere, with respect to the maximum mean discrepancy pseudometric. We demonstrate that this family encompasses several regularizers of former methods, including uniformity-based and information-maximization methods, which are variants of our flexible regularization loss with different kernels. Beyond its practical consequences for state of the art self-supervised learning with limited resources, the proposed generic regularization approach opens perspectives to leverage more widely the literature on kernel methods in order to improve self-supervised learning methods.
[ AD12 ]
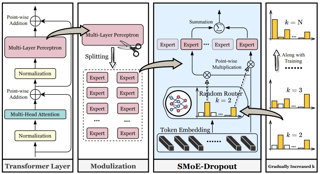
[ AD12 ]

[ AD1 ]
Modern deep learning involves training costly, highly overparameterized networks, thus motivating the search for sparser networks that require less compute and memory but can still be trained to the same accuracy as the full network (i.e. matching). Iterative magnitude pruning (IMP) is a state of the art algorithm that can find such highly sparse matching subnetworks, known as winning tickets. IMP operates by iterative cycles of training, masking a fraction of smallest magnitude weights, rewinding unmasked weights back to an early training point, and repeating. Despite its simplicity, the underlying principles for when and how IMP finds winning tickets remain elusive. In particular, what useful information does an IMP mask found at the end of training convey to a rewound network near the beginning of training? How does SGD allow the network to extract this information? And why is iterative pruning needed, i.e. why can't we prune to very high sparsities in one shot? We develop answers to these questions in terms of the geometry of the error landscape. First, we find that—at higher sparsities—pairs of pruned networks at successive pruning iterations are connected by a linear path with zero error barrier if and only if they are matching. This …
[ AD10 ]
[ AD11 ]
[ Auditorium ]
We are interested in solving a class of problems that seek to understand and adopt rational behavior from demonstrations. We may broadly classify these problems into four categories of reward identification, counterfactual analysis, behavior imitation, and behavior transfer. In this work, we make a key observation that knowing how changes in the underlying rewards affect the optimal behavior allows one to solve a variety of aforementioned problems. To a local approximation, this quantity is precisely captured by what we term the Bellman score, i.e gradient of log probabilities of the optimal policy with respect to the reward. We introduce the Bellman score operator which provably converges to the gradient of the infinite-horizon optimal Q-values with respect to the reward which can then be used to directly estimate the score. Guided by our theory, we derive a practical score-learning algorithm which can be used for score estimation in high-dimensional state-actions spaces. We show that score-learning can be used to reliably identify rewards, perform counterfactual predictions, achieve state-of-the-art behavior imitation, and transfer policies across environments.
[ Auditorium ]
Self-supervised pretraining has been extensively studied in language and vision domains, where a unified model can be easily adapted to various downstream tasks by pretraining representations without explicit labels. When it comes to sequential decision-making tasks, however, it is difficult to properly design such a pretraining approach that can cope with both high-dimensional perceptual information and the complexity of sequential control over long interaction horizons. The challenge becomes combinatorially more complex if we want to pretrain representations amenable to a large variety of tasks. To tackle this problem, in this work, we formulate a general pretraining-finetuning pipeline for sequential decision making, under which we propose a generic pretraining framework \textit{Self-supervised Multi-task pretrAining with contRol Transformer (SMART)}. By systematically investigating pretraining regimes, we carefully design a Control Transformer (CT) coupled with a novel control-centric pretraining objective in a self-supervised manner. SMART encourages the representation to capture the common essential information relevant to short-term control and long-term control, which is transferrable across tasks. We show by extensive experiments in DeepMind Control Suite that SMART significantly improves the learning efficiency among seen and unseen downstream tasks and domains under different learning scenarios including Imitation Learning (IL) and Reinforcement Learning (RL). Benefiting from the …
[ AD1 ]
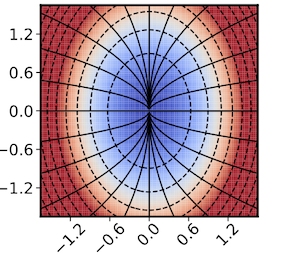
In this work, we explore the maximum-margin bias of quasi-homogeneous neural networks trained with gradient flow on an exponential loss and past a point of separability. We introduce the class of quasi-homogeneous models, which is expressive enough to describe nearly all neural networks with homogeneous activations, even those with biases, residual connections, and normalization layers, while structured enough to enable geometric analysis of its gradient dynamics. Using this analysis, we generalize the existing results of maximum-margin bias for homogeneous networks to this richer class of models. We find that gradient flow implicitly favors a subset of the parameters, unlike in the case of a homogeneous model where all parameters are treated equally. We demonstrate through simple examples how this strong favoritism toward minimizing an asymmetric norm can degrade the robustness of quasi-homogeneous models. On the other hand, we conjecture that this norm-minimization discards, when possible, unnecessary higher-order parameters, reducing the model to a sparser parameterization. Lastly, by applying our theorem to sufficiently expressive neural networks with normalization layers, we reveal a universal mechanism behind the empirical phenomenon of Neural Collapse.
[ AD10 ]
Evaluation metrics in image synthesis play a key role to measure performances of generative models. However, most metrics mainly focus on image fidelity. Existing diversity metrics are derived by comparing distributions, and thus they cannot quantify the diversity or rarity degree of each generated image. In this work, we propose a new evaluation metric, called `rarity score', to measure both image-wise uncommonness and model-wise diversified generation performance. We first show empirical observation that typical samples are close to each other and distinctive samples are far from each other in nearest-neighbor distances on latent spaces represented by feature extractor networks such as VGG16. We then show that one can effectively filter typical or distinctive samples with the proposed metric. We also use our metric to demonstrate that the extent to which different generative models produce rare images can be effectively compared. Further, our metric can be used to compare rarities between datasets that share the same concept such as CelebA-HQ and FFHQ. Finally, we analyze the use of metrics in different designs of feature extractors to better understand the relationship between feature spaces and resulting high-rarity images. Code will be publicly available for the research community.
[ AD12 ]
We present two new benchmarks, MBXP and Multilingual HumanEval, designed to evaluate code completion models in over 10 programming languages. These datasets are generated using a conversion framework that transpiles prompts and test cases from the original MBPP and HumanEval datasets into the corresponding data in the target language. By using these benchmarks, we are able to assess the performance of code generation models in a multi-lingual fashion, and discovered generalization ability of language models on out-of-domain languages, advantages of multi-lingual models over mono-lingual, the ability of few-shot prompting to teach the model new languages, and zero-shot translation abilities. In addition, we use our code generation model to perform large-scale bootstrapping to obtain synthetic canonical solutions in several languages, which can be used for other code-related evaluations such as code insertion, robustness, or summarization tasks.
[ AD11 ]

It is commonly believed that the implicit regularization of optimizers is needed for neural networks to generalize in the overparameterized regime. In this paper, we observe experimentally that this implicit regularization behavior is {\em generic}, i.e. it does not depend strongly on the choice of optimizer. We demonstrate this by training neural networks using several gradient-free optimizers, which do not benefit from properties that are often attributed to gradient-based optimizers. This includes a guess-and-check optimizer that generates uniformly random parameter vectors until finding one that happens to achieve perfect train accuracy, and a zeroth-order Pattern Search optimizer that uses no gradient computations. In the low sample and few-shot regimes, where zeroth order optimizers are most computationally tractable, we find that these non-gradient optimizers achieve test accuracy comparable to SGD. The code to reproduce results can be found at https://github.com/Ping-C/optimizer .
[ AD10 ]
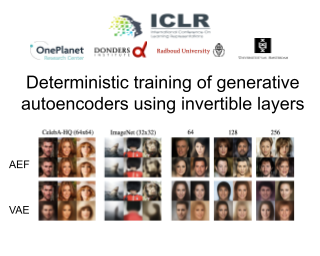
In this work, we provide a deterministic alternative to the stochastic variational training of generative autoencoders. We refer to these new generative autoencoders as AutoEncoders within Flows (AEF), since the encoder and decoder are defined as affine layers of an overall invertible architecture. This results in a deterministic encoding of the data, as opposed to the stochastic encoding of VAEs. The paper introduces two related families of AEFs. The first family relies on a partition of the ambient space and is trained by exact maximum-likelihood. The second family exploits a deterministic expansion of the ambient space and is trained by maximizing the log-probability in this extended space. This latter case leaves complete freedom in the choice of encoder, decoder and prior architectures, making it a drop-in replacement for the training of existing VAEs and VAE-style models. We show that these AEFs can have strikingly higher performance than architecturally identical VAEs in terms of log-likelihood and sample quality, especially for low dimensional latent spaces. Importantly, we show that AEF samples are substantially sharper than VAE samples.
[ Auditorium ]
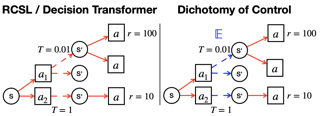
Future- or return-conditioned supervised learning is an emerging paradigm for offline reinforcement learning (RL), in which the future outcome (i.e., return) associated with a sequence of actions in an offline dataset is used as input to a policy trained to imitate those same actions. While return-conditioning is at the heart of popular algorithms such as decision transformer (DT), these methods tend to perform poorly in highly stochastic environments, where an occasional high return associated with a sequence of actions may be due more to the randomness of the environment than to the actions themselves. Such situations can lead to a learned policy that is inconsistent with its conditioning inputs; i.e., using the policy – while conditioned on a specific desired return – to act in the environment can lead to a distribution of real returns that is wildly different than desired. In this work, we propose the dichotomy of control (DoC), a future-conditioned supervised learning framework that separates mechanisms within a policy’s control (actions) from those outside of a policy’s control (environment stochasticity). We achieve this by conditioning the policy on a latent variable representation of the future and designing a mutual information constraint that removes any future information from …
[ AD12 ]
Designing expressive Graph Neural Networks (GNNs) is a central topic in learning graph-structured data. While numerous approaches have been proposed to improve GNNs with respect to the Weisfeiler-Lehman (WL) test, for most of them, there is still a lack of deep understanding of what additional power they can systematically and provably gain. In this paper, we take a fundamentally different perspective to study the expressive power of GNNs beyond the WL test. Specifically, we introduce a novel class of expressivity metrics via graph biconnectivity and highlight their importance in both theory and practice. As biconnectivity can be easily calculated using simple algorithms that have linear computational costs, it is natural to expect that popular GNNs can learn it easily as well. However, after a thorough review of prior GNN architectures, we surprisingly find that most of them are not expressive for any of these metrics. The only exception is the ESAN framework (Bevilacqua et al., 2022), for which we give a theoretical justification of its power. We proceed to introduce a principled and more efficient approach, called the Generalized Distance Weisfeiler-Lehman (GD-WL), which is provably expressive for all biconnectivity metrics. Practically, we show GD-WL can be implemented by a Transformer-like …
[ AD1 ]

Equivariance guarantees that a model's predictions capture key symmetries in data. When an image is translated or rotated, an equivariant model's representation of that image will translate or rotate accordingly. The success of convolutional neural networks has historically been tied to translation equivariance directly encoded in their architecture. The rising success of vision transformers, which have no explicit architectural bias towards equivariance, challenges this narrative and suggests that augmentations and training data might also play a significant role in their performance. In order to better understand the role of equivariance in recent vision models, we apply the Lie derivative, a method for measuring equivariance with strong mathematical foundations and minimal hyperparameters. Using the Lie derivative, we study the equivariance properties of hundreds of pretrained models, spanning CNNs, transformers, and Mixer architectures. The scale of our analysis allows us to separate the impact of architecture from other factors like model size or training method. Surprisingly, we find that many violations of equivariance can be linked to spatial aliasing in ubiquitous network layers, such as pointwise non-linearities, and that as models get larger and more accurate they tend to display more equivariance, regardless of architecture. For example, transformers can be more equivariant …
[ AD11 ]
Optimal transport has emerged as a powerful tool for a variety of problems in machine learning, and it is frequently used to enforce distributional constraints. In this context, existing methods often use either a Wasserstein metric, or else they apply concurrent barycenter approaches when more than two distributions are considered. In this paper, we leverage multi-marginal optimal transport (MMOT), where we take advantage of a procedure that computes a generalized earth mover's distance as a sub-routine. We show that not only is our algorithm computationally more efficient compared to other barycentric-based distance methods, but it has the additional advantage that gradients used for backpropagation can be efficiently computed during the forward pass computation itself, which leads to substantially faster model training. We provide technical details about this new regularization term and its properties, and we present experimental demonstrations of faster runtimes when compared to standard Wasserstein-style methods. Finally, on a range of experiments designed to assess effectiveness at enforcing fairness, we demonstrate our method compares well with alternatives.
[ AD12 ]
In deep reinforcement learning (RL), useful information about the state is inherently tied to its possible future successors. Consequently, encoding features that capture the hierarchical relationships between states into the model's latent representations is often conducive to recovering effective policies. In this work, we study a new class of deep RL algorithms that promote encoding such relationships by using hyperbolic space to model latent representations. However, we find that a naive application of existing methodology from the hyperbolic deep learning literature leads to fatal instabilities due to the non-stationarity and variance characterizing common gradient estimators in RL. Hence, we design a new general method that directly addresses such optimization challenges and enables stable end-to-end learning with deep hyperbolic representations. We empirically validate our framework by applying it to popular on-policy and off-policy RL algorithms on the Procgen and Atari 100K benchmarks, attaining near universal performance and generalization benefits. Given its natural fit, we hope this work will inspire future RL research to consider hyperbolic representations as a standard tool.
[ Auditorium ]

Complex reasoning problems contain states that vary in the computational cost required to determine the right action plan. To take advantage of this property, we propose Adaptive Subgoal Search (AdaSubS), a search method that adaptively adjusts the planning horizon. To this end, AdaSubS generates diverse sets of subgoals at different distances. A verification mechanism is employed to filter out unreachable subgoals swiftly, making it possible to focus on feasible further subgoals. In this way, AdaSubS benefits from the efficiency of planning with longer-term subgoals and the fine control with shorter-term ones, and thus scales well to difficult planning problems. We show that AdaSubS significantly surpasses hierarchical planning algorithms on three complex reasoning tasks: Sokoban, the Rubik’s Cube, and the inequality-proving benchmark INT.
[ AD10 ]
[ AD11 ]
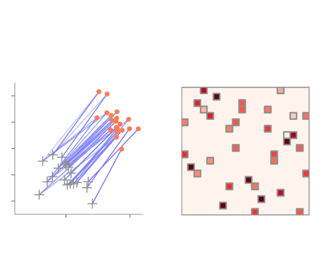
[ AD1 ]
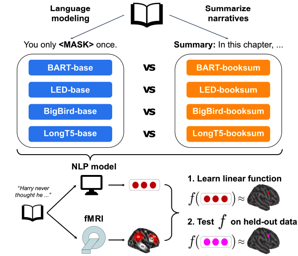
Building systems that achieve a deeper understanding of language is one of the central goals of natural language processing (NLP). Towards this goal, recent works have begun to train language models on narrative datasets which require extracting the most critical information by integrating across long contexts. However, it is still an open question whether these models are learning a deeper understanding of the text, or if the models are simply learning a heuristic to complete the task. This work investigates this further by turning to the one language processing system that truly understands complex language: the human brain. We show that training language models for deeper narrative understanding results in richer representations that have improved alignment to human brain activity. We further find that the improvements in brain alignment are larger for character names than for other discourse features, which indicates that these models are learning important narrative elements. Taken together, these results suggest that this type of training can indeed lead to deeper language understanding. These findings have consequences both for cognitive neuroscience by revealing some of the significant factors behind brain-NLP alignment, and for NLP by highlighting that understanding of long-range context can be improved beyond language modeling.
[ AD11 ]
[ AD1 ]

Acquiring labeled data is challenging in many machine learning applications with limited budgets. Active learning gives a procedure to select the most informative data points and improve data efficiency by reducing the cost of labeling. The info-max learning principle maximizing mutual information such as BALD has been successful and widely adapted in various active learning applications. However, this pool-based specific objective inherently introduces a redundant selection and further requires a high computational cost for batch selection. In this paper, we design and propose a new uncertainty measure, Balanced Entropy Acquisition (BalEntAcq), which captures the information balance between the uncertainty of underlying softmax probability and the label variable. To do this, we approximate each marginal distribution by Beta distribution. Beta approximation enables us to formulate BalEntAcq as a ratio between an augmented entropy and the marginalized joint entropy. The closed-form expression of BalEntAcq facilitates parallelization by estimating two parameters in each marginal Beta distribution. BalEntAcq is a purely standalone measure without requiring any relational computations with other data points. Nevertheless, BalEntAcq captures a well-diversified selection near the decision boundary with a margin, unlike other existing uncertainty measures such as BALD, Entropy, or Mean Standard Deviation (MeanSD). Finally, we demonstrate that our …
[ AD12 ]

Dropped into an unknown environment, what should an agent do to quickly learn about the environment and how to accomplish diverse tasks within it? We address this question within the goal-conditioned reinforcement learning paradigm, by identifying how the agent should set its goals at training time to maximize exploration. We propose "Planning Exploratory Goals" (PEG), a method that sets goals for each training episode to directly optimize an intrinsic exploration reward. PEG first chooses goal commands such that the agent's goal-conditioned policy, at its current level of training, will end up in states with high exploration potential. It then launches an exploration policy starting at those promising states. To enable this direct optimization, PEG learns world models and adapts sampling-based planning algorithms to "plan goal commands". In challenging simulated robotics environments including a multi-legged ant robot in a maze, and a robot arm on a cluttered tabletop, PEG exploration enables more efficient and effective training of goal-conditioned policies relative to baselines and ablations. Our ant successfully navigates a long maze, and the robot arm successfully builds a stack of three blocks upon command. Website: https://sites.google.com/view/exploratory-goals
[ Auditorium ]
Class imbalance problems frequently occur in real-world tasks, and conventional deep learning algorithms are well known for performance degradation on imbalanced training datasets. To mitigate this problem, many approaches have aimed to balance among given classes by re-weighting or re-sampling training samples. These re-balancing methods increase the impact of minority classes and reduce the influence of majority classes on the output of models. However, the extracted representations may be of poor quality owing to the limited number of minority samples. To handle this restriction, several methods have been developed that increase the representations of minority samples by leveraging the features of the majority samples. Despite extensive recent studies, no deep analysis has been conducted on determination of classes to be augmented and strength of augmentation has been conducted. In this study, we first investigate the correlation between the degree of augmentation and class-wise performance, and find that the proper degree of augmentation must be allocated for each class to mitigate class imbalance problems. Motivated by this finding, we propose a simple and efficient novel curriculum, which is designed to find the appropriate per-class strength of data augmentation, called CUDA: CUrriculum of Data Augmentation for long-tailed recognition. CUDA can simply be …
[ AD4 ]
[ AD10 ]
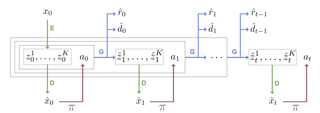
Deep reinforcement learning agents are notoriously sample inefficient, which considerably limits their application to real-world problems. Recently, many model-based methods have been designed to address this issue, with learning in the imagination of a world model being one of the most prominent approaches. However, while virtually unlimited interaction with a simulated environment sounds appealing, the world model has to be accurate over extended periods of time. Motivated by the success of Transformers in sequence modeling tasks, we introduce IRIS, a data-efficient agent that learns in a world model composed of a discrete autoencoder and an autoregressive Transformer. With the equivalent of only two hours of gameplay in the Atari 100k benchmark, IRIS achieves a mean human normalized score of 1.046, and outperforms humans on 10 out of 26 games, setting a new state of the art for methods without lookahead search. To foster future research on Transformers and world models for sample-efficient reinforcement learning, we release our code and models at https://github.com/eloialonso/iris.
[ AD11 ]

Auxiliary objectives, supplementary learning signals that are introduced to help aid learning on data-starved or highly complex end-tasks, are commonplace in machine learning. Whilst much work has been done to formulate useful auxiliary objectives, their construction is still an art which proceeds by slow and tedious hand-design. Intuition for how and when these objectives improve end-task performance has also had limited theoretical backing. In this work, we present an approach for automatically generating a suite of auxiliary objectives. We achieve this by deconstructing existing objectives within a novel unified taxonomy, identifying connections between them, and generating new ones based on the uncovered structure. Next, we theoretically formalize widely-held intuitions about how auxiliary learning improves generalization on the end-task. This leads us to a principled and efficient algorithm for searching the space of generated objectives to find those most useful to a specified end-task.With natural language processing (NLP) as our domain of study, we demonstrate that our automated auxiliary learning pipeline leads to strong improvements over competitive baselines across continued training experiments on a pre-trained model on 5 NLP end-tasks.
[ AD12 ]
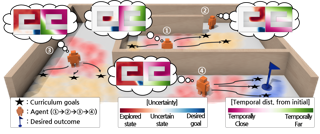
Current reinforcement learning (RL) often suffers when solving a challenging exploration problem where the desired outcomes or high rewards are rarely observed. Even though curriculum RL, a framework that solves complex tasks by proposing a sequence of surrogate tasks, shows reasonable results, most of the previous works still have difficulty in proposing curriculum due to the absence of a mechanism for obtaining calibrated guidance to the desired outcome state without any prior domain knowledge. To alleviate it, we propose an uncertainty \& temporal distance-aware curriculum goal generation method for the outcome-directed RL via solving a bipartite matching problem. It could not only provide precisely calibrated guidance of the curriculum to the desired outcome states but also bring much better sample efficiency and geometry-agnostic curriculum goal proposal capability compared to previous curriculum RL methods. We demonstrate that our algorithm significantly outperforms these prior methods in a variety of challenging navigation tasks and robotic manipulation tasks in a quantitative and qualitative way.
[ AD1 ]
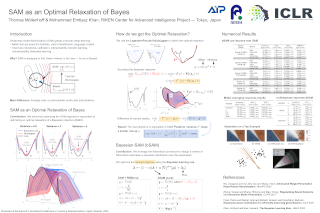
Sharpness-aware minimization (SAM) and related adversarial deep-learning methods can drastically improve generalization, but their underlying mechanisms are not yet fully understood. Here, we establish SAM as a relaxation of the Bayes objective where the expected negative-loss is replaced by the optimal convex lower bound, obtained by using the so-called Fenchel biconjugate. The connection enables a new Adam-like extension of SAM to automatically obtain reasonable uncertainty estimates, while sometimes also improving its accuracy. By connecting adversarial and Bayesian methods, our work opens a new path to robustness.
[ AD10 ]
The ability to continuously acquire new knowledge and skills is crucial for autonomous agents. Existing methods are typically based on either fixed-size models that struggle to learn a large number of diverse behaviors, or growing-size models that scale poorly with the number of tasks. In this work, we aim to strike a better balance between scalability and performance by designing a method whose size grows adaptively depending on the task sequence. We introduce Continual Subspace of Policies (CSP), a new approach that incrementally builds a subspace of policies for training a reinforcement learning agent on a sequence of tasks. The subspace's high expressivity allows CSP to perform well for many different tasks while growing more slowly than the number of tasks. Our method does not suffer from forgetting and also displays positive transfer to new tasks. CSP outperforms a number of popular baselines on a wide range of scenarios from two challenging domains, Brax (locomotion) and Continual World (robotic manipulation). Interactive visualizations of the subspace can be found at https://share.streamlit.io/continual-subspace/policies/main.
[ AD4 ]
[ Auditorium ]
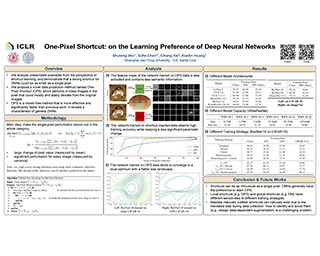
[ Auditorium ]
Deep regression networks are widely used to tackle the problem of predicting a continuous value for a given input. Task-specialized approaches for training regression networks have shown significant improvement over generic approaches, such as direct regression. More recently, a generic approach based on regression by binary classification using binary-encoded labels has shown significant improvement over direct regression. The space of label encodings for regression is large. Lacking heretofore have been automated approaches to find a good label encoding for a given application. This paper introduces Regularized Label Encoding Learning (RLEL) for end-to-end training of an entire network and its label encoding. RLEL provides a generic approach for tackling regression. Underlying RLEL is our observation that the search space of label encodings can be constrained and efficiently explored by using a continuous search space of real-valued label encodings combined with a regularization function designed to encourage encodings with certain properties. These properties balance the probability of classification error in individual bits against error correction capability. Label encodings found by RLEL result in lower or comparable errors to manually designed label encodings. Applying RLEL results in 10.9% and 12.4% improvement in Mean Absolute Error (MAE) over direct regression and multiclass classification, respectively. …
[ AD12 ]
In off-policy deep reinforcement learning with continuous action spaces, exploration is often implemented by injecting action noise into the action selection process. Popular algorithms based on stochastic policies, such as SAC or MPO, inject white noise by sampling actions from uncorrelated Gaussian distributions. In many tasks, however, white noise does not provide sufficient exploration, and temporally correlated noise is used instead. A common choice is Ornstein-Uhlenbeck (OU) noise, which is closely related to Brownian motion (red noise). Both red noise and white noise belong to the broad family of colored noise. In this work, we perform a comprehensive experimental evaluation on MPO and SAC to explore the effectiveness of other colors of noise as action noise. We find that pink noise, which is halfway between white and red noise, significantly outperforms white noise, OU noise, and other alternatives on a wide range of environments. Thus, we recommend it as the default choice for action noise in continuous control.
[ AD4 ]
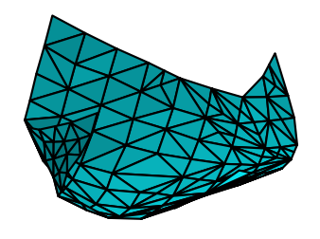
Simulating the time evolution of physical systems is pivotal in many scientific and engineering problems. An open challenge in simulating such systems is their multi-resolution dynamics: a small fraction of the system is extremely dynamic, and requires very fine-grained resolution, while a majority of the system is changing slowly and can be modeled by coarser spatial scales. Typical learning-based surrogate models use a uniform spatial scale, which needs to resolve to the finest required scale and can waste a huge compute to achieve required accuracy. In this work, we introduce Learning controllable Adaptive simulation for Multi-resolution Physics (LAMP) as the first full deep learning-based surrogate model that jointly learns the evolution model and optimizes appropriate spatial resolutions that devote more compute to the highly dynamic regions. LAMP consists of a Graph Neural Network (GNN) for learning the forward evolution, and a GNN-based actor-critic for learning the policy of spatial refinement and coarsening. We introduce learning techniques that optimizes LAMP with weighted sum of error and computational cost as objective, allowing LAMP to adapt to varying relative importance of error vs. computation tradeoff at inference time. We evaluate our method in a 1D benchmark of nonlinear PDEs and a challenging 2D …
[ AD10 ]
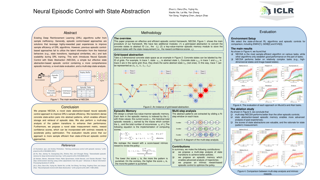
Existing Deep Reinforcement Learning (DRL) algorithms suffer from sample inefficiency. Generally, episodic control-based approaches are solutions that leverage highly rewarded past experiences to improve sample efficiency of DRL algorithms. However, previous episodic control-based approaches fail to utilize the latent information from the historical behaviors (\eg, state transitions, topological similarities, \etc) and lack scalability during DRL training. This work introduces Neural Episodic Control with State Abstraction (NECSA), a simple but effective state abstraction-based episodic control containing a more comprehensive episodic memory, a novel state evaluation, and a multi-step state analysis. We evaluate our approach to the MuJoCo and Atari tasks in OpenAI gym domains. The experimental results indicate that NECSA achieves higher sample efficiency than the state-of-the-art episodic control-based approaches. Our data and code are available at the project website\footnote{\url{https://sites.google.com/view/drl-necsa}}.
[ AD11 ]
Learning with few labeled tabular samples is often an essential requirement for industrial machine learning applications as varieties of tabular data suffer from high annotation costs or have difficulties in collecting new samples for novel tasks. Despite the utter importance, such a problem is quite under-explored in the field of tabular learning, and existing few-shot learning schemes from other domains are not straightforward to apply, mainly due to the heterogeneous characteristics of tabular data. In this paper, we propose a simple yet effective framework for few-shot semi-supervised tabular learning, coined Self-generated Tasks from UNlabeled Tables (STUNT). Our key idea is to self-generate diverse few-shot tasks by treating randomly chosen columns as a target label. We then employ a meta-learning scheme to learn generalizable knowledge with the constructed tasks. Moreover, we introduce an unsupervised validation scheme for hyperparameter search (and early stopping) by generating a pseudo-validation set using STUNT from unlabeled data. Our experimental results demonstrate that our simple framework brings significant performance gain under various tabular few-shot learning benchmarks, compared to prior semi- and self-supervised baselines. Code is available at https://github.com/jaehyun513/STUNT.
[ AD1 ]
The Generative Flow Network is a probabilistic framework where an agent learns a stochastic policy for object generation, such that the probability of generating an object is proportional to a given reward function. Its effectiveness has been shown in discovering high-quality and diverse solutions, compared to reward-maximizing reinforcement learning-based methods. Nonetheless, GFlowNets only learn from rewards of the terminal states, which can limit its applicability. Indeed, intermediate rewards play a critical role in learning, for example from intrinsic motivation to provide intermediate feedback even in particularly challenging sparse reward tasks. Inspired by this, we propose Generative Augmented Flow Networks (GAFlowNets), a novel learning framework to incorporate intermediate rewards into GFlowNets. We specify intermediate rewards by intrinsic motivation to tackle the exploration problem in sparse reward environments. GAFlowNets can leverage edge-based and state-based intrinsic rewards in a joint way to improve exploration. Based on extensive experiments on the GridWorld task, we demonstrate the effectiveness and efficiency of GAFlowNet in terms of convergence, performance, and diversity of solutions. We further show that GAFlowNet is scalable to a more complex and large-scale molecule generation domain, where it achieves consistent and significant performance improvement.
[ AD11 ]
Masked Autoencoder (MAE) is a prevailing self-supervised learning method that achieves promising results in model pre-training. However, when the various downstream tasks have data distributions different from the pre-training data, the semantically irrelevant pre-training information might result in negative transfer, impeding MAE’s scalability. To address this issue, we propose a novel MAE-based pre-training paradigm, Mixture of Cluster-conditional Experts (MoCE), which can be trained once but provides customized pre-training models for diverse downstream tasks. Different from the mixture of experts (MoE), our MoCE trains each expert only with semantically relevant images by using cluster-conditional gates. Thus, each downstream task can be allocated to its customized model pre-trained with data most similar to the downstream data. Experiments on a collection of 11 downstream tasks show that MoCE outperforms the vanilla MAE by 2.45\% on average. It also obtains new state-of-the-art self-supervised learning results on detection and segmentation.
[ AD12 ]
Learning policies from previously recorded data is a promising direction for real-world robotics tasks, as online learning is often infeasible. Dexterous manipulation in particular remains an open problem in its general form. The combination of offline reinforcement learning with large diverse datasets, however, has the potential to lead to a breakthrough in this challenging domain analogously to the rapid progress made in supervised learning in recent years. To coordinate the efforts of the research community toward tackling this problem, we propose a benchmark including: i) a large collection of data for offline learning from a dexterous manipulation platform on two tasks, obtained with capable RL agents trained in simulation; ii) the option to execute learned policies on a real-world robotic system and a simulation for efficient debugging. We evaluate prominent open-sourced offline reinforcement learning algorithms on the datasets and provide a reproducible experimental setup for offline reinforcement learning on real systems.
[ AD1 ]
Estimating the 3DoF rotation from a single RGB image is an important yet challenging problem. Probabilistic rotation regression has raised more and more attention with the benefit of expressing uncertainty information along with the prediction. Though modeling noise using Gaussian-resembling Bingham distribution and matrix Fisher distribution is natural, they are shown to be sensitive to outliers for the nature of quadratic punishment to deviations. In this paper, we draw inspiration from multivariate Laplace distribution and propose a novel Rotation Laplace distribution on SO(3). Rotation Laplace distribution is robust to the disturbance of outliers and enforces much gradient to the low-error region, resulting in a better convergence. Our extensive experiments show that our proposed distribution achieves state-of-the-art performance for rotation regression tasks over both probabilistic and non-probabilistic baselines. Our project page is at pku-epic.github.io/RotationLaplace.
[ AD10 ]
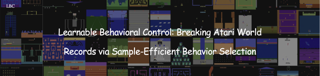
The exploration problem is one of the main challenges in deep reinforcement learning (RL). Recent promising works tried to handle the problem with population-based methods, which collect samples with diverse behaviors derived from a population of different exploratory policies. Adaptive policy selection has been adopted for behavior control. However, the behavior selection space is largely limited by the predefined policy population, which further limits behavior diversity. In this paper, we propose a general framework called Learnable Behavioral Control (LBC) to address the limitation, which a) enables a significantly enlarged behavior selection space via formulating a hybrid behavior mapping from all policies; b) constructs a unified learnable process for behavior selection. We introduce LBC into distributed off-policy actor-critic methods and achieve behavior control via optimizing the selection of the behavior mappings with bandit-based meta-controllers. Our agents have achieved 10077.52% mean human normalized score and surpassed 24 human world records within 1B training frames in the Arcade Learning Environment, which demonstrates our significant state-of-the-art (SOTA) performance without degrading the sample efficiency.
[ Auditorium ]
Disentangling complex data to its latent factors of variation is a fundamental task in representation learning. Existing work on sequential disentanglement mostly provides two factor representations, i.e., it separates the data to time-varying and time-invariant factors. In contrast, we consider multifactor disentanglement in which multiple (more than two) semantic disentangled components are generated. Key to our approach is a strong inductive bias where we assume that the underlying dynamics can be represented linearly in the latent space. Under this assumption, it becomes natural to exploit the recently introduced Koopman autoencoder models. However, disentangled representations are not guaranteed in Koopman approaches, and thus we propose a novel spectral loss term which leads to structured Koopman matrices and disentanglement. Overall, we propose a simple and easy to code new deep model that is fully unsupervised and it supports multifactor disentanglement. We showcase new disentangling abilities such as swapping of individual static factors between characters, and an incremental swap of disentangled factors from the source to the target. Moreover, we evaluate our method extensively on two factor standard benchmark tasks where we significantly improve over competing unsupervised approaches, and we perform competitively in comparison to weakly- and self-supervised state-of-the-art approaches. The code is …
[ AD4 ]
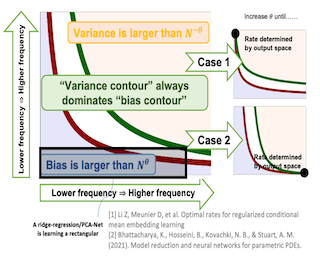
Learning mappings between infinite-dimensional function spaces have achieved empirical success in many disciplines of machine learning, including generative modeling, functional data analysis, causal inference, and multi-agent reinforcement learning. In this paper, we study the statistical limit of learning a Hilbert-Schmidt operator between two infinite-dimensional Sobolev reproducing kernel Hilbert spaces. We establish the information-theoretic lower bound in terms of the Sobolev Hilbert-Schmidt norm and show that a regularization that learns the spectral components below the bias contour and ignores the ones above the variance contour can achieve the optimal learning rate. At the same time, the spectral components between the bias and variance contours give us flexibility in designing computationally feasible machine learning algorithms. Based on this observation, we develop a multilevel kernel operator learning algorithm that is optimal when learning linear operators between infinite-dimensional function spaces.
[ Auditorium ]
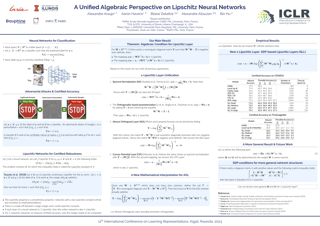
Important research efforts have focused on the design and training of neural networks with a controlled Lipschitz constant. The goal is to increase and sometimes guarantee the robustness against adversarial attacks. Recent promising techniques draw inspirations from different backgrounds to design 1-Lipschitz neural networks, just to name a few: convex potential layers derive from the discretization of continuous dynamical systems, Almost-Orthogonal-Layer proposes a tailored method for matrix rescaling. However, it is today important to consider the recent and promising contributions in the field under a common theoretical lens to better design new and improved layers. This paper introduces a novel algebraic perspective unifying various types of 1-Lipschitz neural networks, including the ones previously mentioned, along with methods based on orthogonality and spectral methods. Interestingly, we show that many existing techniques can be derived and generalized via finding analytical solutions of a common semidefinite programming (SDP) condition. We also prove that AOL biases the scaled weight to the ones which are close to the set of orthogonal matrices in a certain mathematical manner. Moreover, our algebraic condition, combined with the Gershgorin circle theorem, readily leads to new and diverse parameterizations for 1-Lipschitz network layers. Our approach, called SDP-based Lipschitz Layers (SLL), …
[ AD11 ]
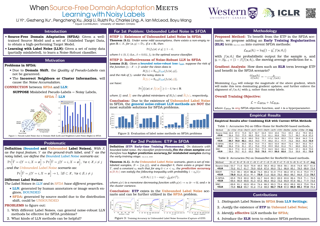
Recent state-of-the-art source-free domain adaptation (SFDA) methods have focused on learning meaningful cluster structures in the feature space, which have succeeded in adapting the knowledge from source domain to unlabeled target domain without accessing the private source data. However, existing methods rely on the pseudo-labels generated by source models that can be noisy due to domain shift. In this paper, we study SFDA from the perspective of learning with label noise (LLN). Unlike the label noise in the conventional LLN scenario, we prove that the label noise in SFDA follows a different distribution assumption. We also prove that such a difference makes existing LLN methods that rely on their distribution assumptions unable to address the label noise in SFDA. Empirical evidence suggests that only marginal improvements are achieved when applying the existing LLN methods to solve the SFDA problem. On the other hand, although there exists a fundamental difference between the label noise in the two scenarios, we demonstrate theoretically that the early-time training phenomenon (ETP), which has been previously observed in conventional label noise settings, can also be observed in the SFDA problem. Extensive experiments demonstrate significant improvements to existing SFDA algorithms by leveraging ETP to address the label …
[ AD1 ]

Previous studies have shown that leveraging "domain index" can significantly boost domain adaptation performance (Wang et al., 2020; Xu et al., 2022). However, such domain indices are not always available. To address this challenge, we first provide a formal definition of domain index from the probabilistic perspective, and then propose an adversarial variational Bayesian framework that infers domain indices from multi-domain data, thereby providing additional insight on domain relations and improving domain adaptation performance. Our theoretical analysis shows that our adversarial variational Bayesian framework finds the optimal domain index at equilibrium. Empirical results on both synthetic and real data verify that our model can produce interpretable domain indices which enable us to achieve superior performance compared to state-of-the-art domain adaptation methods. Code is available at https://github.com/Wang-ML-Lab/VDI.
[ AD10 ]
[ AD12 ]
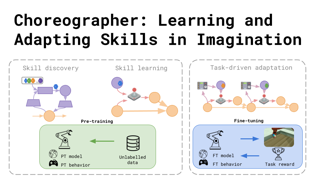
Unsupervised skill learning aims to learn a rich repertoire of behaviors without external supervision, providing artificial agents with the ability to control and influence the environment. However, without appropriate knowledge and exploration, skills may provide control only over a restricted area of the environment, limiting their applicability. Furthermore, it is unclear how to leverage the learned skill behaviors for adapting to downstream tasks in a data-efficient manner. We present Choreographer, a model-based agent that exploits its world model to learn and adapt skills in imagination. Our method decouples the exploration and skill learning processes, being able to discover skills in the latent state space of the model. During adaptation, the agent uses a meta-controller to evaluate and adapt the learned skills efficiently by deploying them in parallel in imagination. Choreographer is able to learn skills both from offline data, and by collecting data simultaneously with an exploration policy. The skills can be used to effectively adapt to downstream tasks, as we show in the URL benchmark, where we outperform previous approaches from both pixels and states inputs. The skills also explore the environment thoroughly, finding sparse rewards more frequently, as shown in goal-reaching tasks from the DMC Suite and Meta-World. …
[ AD4 ]

Population dynamics is the study of temporal and spatial variation in the size of populations of organisms and is a major part of population ecology. One of the main difficulties in analyzing population dynamics is that we can only obtain observation data with coarse time intervals from fixed-point observations due to experimental costs or measurement constraints. Recently, modeling population dynamics by using continuous normalizing flows (CNFs) and dynamic optimal transport has been proposed to infer the sample trajectories from a fixed-point observed population. While the sample behavior in CNFs is deterministic, the actual sample in biological systems moves in an essentially random yet directional manner. Moreover, when a sample moves from point A to point B in dynamical systems, its trajectory typically follows the principle of least action in which the corresponding action has the smallest possible value. To satisfy these requirements of the sample trajectories, we formulate the Lagrangian Schrödinger bridge (LSB) problem and propose to solve it approximately by modeling the advection-diffusion process with regularized neural SDE. We also develop a model architecture that enables faster computation of the loss function. Experimental results show that the proposed method can efficiently approximate the population-level dynamics even for high-dimensional data …
[ AD1 ]
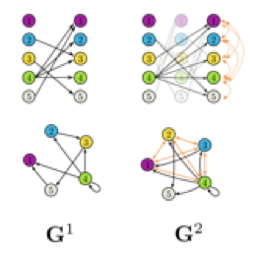
[ Auditorium ]
While large-scale sequence modelling from offline data has led to impressive performance gains in natural language generation and image generation, directly translating such ideas to robotics has been challenging. One critical reason for this is that uncurated robot demonstration data, i.e. play data, collected from non-expert human demonstrators are often noisy, diverse, and distributionally multi-modal. This makes extracting useful, task-centric behaviors from such data a difficult generative modelling problem. In this work, we present Conditional Behavior Transformers (C-BeT), a method that combines the multi-modal generation ability of Behavior Transformer with future-conditioned goal specification. On a suite of simulated benchmark tasks, we find that C-BeT improves upon prior state-of-the-art work in learning from play data by an average of 45.7%. Further, we demonstrate for the first time that useful task-centric behaviors can be learned on a real-world robot purely from play data without any task labels or reward information. Robot videos are best viewed on our project website: play-to-policy.github.io
[ AD11 ]
Test-time adaptation (TTA) has shown to be effective at tackling distribution shifts between training and testing data by adapting a given model on test samples. However, the online model updating of TTA may be unstable and this is often a key obstacle preventing existing TTA methods from being deployed in the real world. Specifically, TTA may fail to improve or even harm the model performance when test data have: 1) mixed distribution shifts, 2) small batch sizes, and 3) online imbalanced label distribution shifts, which are quite common in practice. In this paper, we investigate the unstable reasons and find that the batch norm layer is a crucial factor hindering TTA stability. Conversely, TTA can perform more stably with batch-agnostic norm layers, i.e., group or layer norm. However, we observe that TTA with group and layer norms does not always succeed and still suffers many failure cases. By digging into the failure cases, we find that certain noisy test samples with large gradients may disturb the model adaption and result in collapsed trivial solutions, i.e., assigning the same class label for all samples. To address the above collapse issue, we propose a sharpness-aware and reliable entropy minimization method, called SAR, …
[ AD10 ]

Recent improvements in conditional generative modeling have made it possible to generate high-quality images from language descriptions alone. We investigate whether these methods can directly address the problem of sequential decision-making. We view decision-making not through the lens of reinforcement learning (RL), but rather through conditional generative modeling. To our surprise, we find that our formulation leads to policies that can outperform existing offline RL approaches across standard benchmarks. By modeling a policy as a return-conditional generative model, we avoid the need for dynamic programming and subsequently eliminate many of the complexities that come with traditional offline RL. We further demonstrate the advantages of modeling policies as conditional generative models by considering two other conditioning variables: constraints and skills. Conditioning on a single constraint or skill during training leads to behaviors at test-time that can satisfy several constraints together or demonstrate a composition of skills. Our results illustrate that conditional generative modeling is a powerful tool for decision-making.
[ AD4 ]
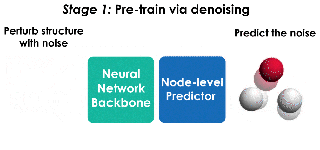
Many important problems involving molecular property prediction from 3D structures have limited data, posing a generalization challenge for neural networks. In this paper, we describe a pre-training technique based on denoising that achieves a new state-of-the-art in molecular property prediction by utilizing large datasets of 3D molecular structures at equilibrium to learn meaningful representations for downstream tasks. Relying on the well-known link between denoising autoencoders and score-matching, we show that the denoising objective corresponds to learning a molecular force field -- arising from approximating the Boltzmann distribution with a mixture of Gaussians -- directly from equilibrium structures. Our experiments demonstrate that using this pre-training objective significantly improves performance on multiple benchmarks, achieving a new state-of-the-art on the majority of targets in the widely used QM9 dataset. Our analysis then provides practical insights into the effects of different factors -- dataset sizes, model size and architecture, and the choice of upstream and downstream datasets -- on pre-training.
[ AD12 ]
Meta-reinforcement learning has widely been used as a learning-to-learn framework to solve unseen tasks with limited experience. However, the aspect of constraint violations has not been adequately addressed in the existing works, making their application restricted in real-world settings. In this paper, we study the problem of meta-safe reinforcement learning (meta-SRL) through the CMDP-within-online framework. We obtain task-averaged regret guarantees for the reward maximization (optimality gap) and constraint violations using gradient-based meta-learning and show that the task-averaged optimality gap and constraint satisfaction improve with task-similarity in the static environment, or task-relatedness in the changing environment. Several technical challenges arise when making this framework practical while still having strong theoretical guarantees. To address these challenges, we propose a meta-algorithm that performs inexact online learning on the upper bounds of intra-task optimality gap and constraint violations estimated by off-policy stationary distribution corrections. Furthermore, we enable the learning rates to be adapted for every task and extend our approach to settings with the dynamically changing task environments. Finally, experiments are conducted to demonstrate the effectiveness of our approach. The proposed theoretical framework is the first to handle the nonconvexity and stochastic nature of within-task CMDPs, while exploiting inter-task dependency for multi-task safe learning.
[ AD11 ]
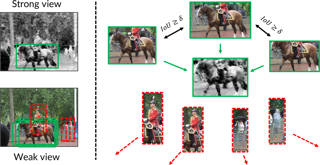
The use of pretrained deep neural networks represents an attractive way to achieve strong results with few data available. When specialized in dense problems such as object detection, learning local rather than global information in images has proven to be more efficient. However, for unsupervised pretraining, the popular contrastive learning requires a large batch size and, therefore, a lot of resources. To address this problem, we are interested in transformer-based object detectors that have recently gained traction in the community with good performance and with the particularity of generating many diverse object proposals. In this work, we present Proposal Selection Contrast (ProSeCo), a novel unsupervised overall pretraining approach that leverages this property. ProSeCo uses the large number of object proposals generated by the detector for contrastive learning, which allows the use of a smaller batch size, combined with object-level features to learn local information in the images. To improve the effectiveness of the contrastive loss, we introduce the object location information in the selection of positive examples to take into account multiple overlapping object proposals. When reusing pretrained backbone, we advocate for consistency in learning local information between the backbone and the detection head. We show that our method outperforms …
[ AD10 ]
[ AD1 ]
Discovering causal relationships between different variables from time series data has been a long-standing challenge for many domains. For example, in stock markets, the announcement of acquisitions from leading companies may have immediate effects on stock prices and increase the uncertainty of the future market due to this past action. To discover causal relations in such case, the model needs to consider non-linear relations between variables, instantaneous effect and the change of noise distribution due to past actions. We name the latter as history-dependent noise. However, previous works do not offer a solution addressing all these problems together. In this paper, we propose a structural equation model, called Rhino, which combines vector auto-regression, deep learning and variational inference to model non-linear relationships with instantaneous effects while allowing the noise distribution to be modulated by history observations. Theoretically, we prove the structural identifiability of Rhino. Our empirical results from extensive synthetic experiments and two real-world benchmarks demonstrate better discovery performance compared to relevant baselines, with ablation studies revealing its robustness under model misspecification.
[ AD12 ]
Offline reinforcement learning (RL) promises the ability to learn effective policies solely using existing, static datasets, without any costly online interaction. To do so, offline RL methods must handle distributional shift between the dataset and the learned policy. The most common approach is to learn conservative, or lower-bound, value functions, which underestimate the return of OOD actions. However, such methods exhibit one notable drawback: policies optimized on such value functions can only behave according to a fixed, possibly suboptimal, degree of conservatism. However, this can be alleviated if we instead are able to learn policies for varying degrees of conservatism at training time and devise a method to dynamically choose one of them during evaluation. To do so, in this work, we propose learning value functions that additionally condition on the degree of conservatism, which we dub confidence-conditioned value functions. We derive a new form of a Bellman backup that simultaneously learns Q-values for any degree of confidence with high probability. By conditioning on confidence, our value functions enable adaptive strategies during online evaluation by controlling for confidence level using the history of observations thus far. This approach can be implemented in practice by conditioning the Q-function from existing conservative …
[ Auditorium ]
The success of deep learning is due in large part to our ability to solve certain massive non-convex optimization problems with relative ease. Though non-convex optimization is NP-hard, simple algorithms -- often variants of stochastic gradient descent -- exhibit surprising effectiveness in fitting large neural networks in practice. We argue that neural network loss landscapes often contain (nearly) a single basin after accounting for all possible permutation symmetries of hidden units a la Entezari et al. 2021. We introduce three algorithms to permute the units of one model to bring them into alignment with a reference model in order to merge the two models in weight space. This transformation produces a functionally equivalent set of weights that lie in an approximately convex basin near the reference model. Experimentally, we demonstrate the single basin phenomenon across a variety of model architectures and datasets, including the first (to our knowledge) demonstration of zero-barrier linear mode connectivity between independently trained ResNet models on CIFAR-10. Additionally, we identify intriguing phenomena relating model width and training time to mode connectivity. Finally, we discuss shortcomings of the linear mode connectivity hypothesis, including a counterexample to the single basin theory.
[ AD4 ]
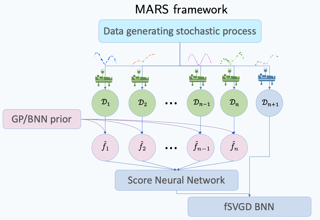
Meta-learning aims to extract useful inductive biases from a set of related datasets. In Bayesian meta-learning, this is typically achieved by constructing a prior distribution over neural network parameters. However, specifying families of computationally viable prior distributions over the high-dimensional neural network parameters is difficult. As a result, existing approaches resort to meta-learning restrictive diagonal Gaussian priors, severely limiting their expressiveness and performance. To circumvent these issues, we approach meta-learning through the lens of functional Bayesian neural network inference which views the prior as a stochastic process and performs inference in the function space. Specifically, we view the meta-training tasks as samples from the data-generating process and formalize meta-learning as empirically estimating the law of this stochastic process. Our approach can seamlessly acquire and represent complex prior knowledge by meta-learning the score function of the data-generating process marginals instead of parameter space priors. In a comprehensive benchmark, we demonstrate that our method achieves state-of-the-art performance in terms of predictive accuracy and substantial improvements in the quality of uncertainty estimates.
[ Auditorium ]
We propose Algorithm Distillation (AD), a method for distilling reinforcement learning (RL) algorithms into neural networks by modeling their training histories with a causal sequence model. Algorithm Distillation treats learning to reinforcement learn as an across-episode sequential prediction problem. A dataset of learning histories is generated by a source RL algorithm, and then a causal transformer is trained by autoregressively predicting actions given their preceding learning histories as context. Unlike sequential policy prediction architectures that distill post-learning or expert sequences, AD is able to improve its policy entirely in-context without updating its network parameters. We demonstrate that AD can reinforcement learn in-context in a variety of environments with sparse rewards, combinatorial task structure, and pixel-based observations, and find that AD learns a more data-efficient RL algorithm than the one that generated the source data.
[ AD12 ]
Modern Deep Reinforcement Learning (RL) algorithms require estimates of the maximal Q-value, which are difficult to compute in continuous domains with an infinite number of possible actions. In this work, we introduce a new update rule for online and offline RL which directly models the maximal value using Extreme Value Theory (EVT), drawing inspiration from economics. By doing so, we avoid computing Q-values using out-of-distribution actions which is often a substantial source of error. Our key insight is to introduce an objective that directly estimates the optimal soft-value functions (LogSumExp) in the maximum entropy RL setting without needing to sample from a policy. Using EVT, we derive our \emph{Extreme Q-Learning} framework and consequently online and, for the first time, offline MaxEnt Q-learning algorithms, that do not explicitly require access to a policy or its entropy. Our method obtains consistently strong performance in the D4RL benchmark, outperforming prior works by \emph{10+ points} on the challenging Franka Kitchen tasks while offering moderate improvements over SAC and TD3 on online DM Control tasks. Visualizations and code can be found on our website.
[ AD11 ]
In this paper, we show that recent advances in self-supervised representation learning enable unsupervised object discovery and semantic segmentation with a performance that matches the state of the field on supervised semantic segmentation 10 years ago. We propose a methodology based on unsupervised saliency masks and self-supervised feature clustering to kickstart object discovery followed by training a semantic segmentation network on pseudo-labels to bootstrap the system on images with multiple objects. We show that while being conceptually simple our proposed baseline is surprisingly strong. We present results on PASCAL VOC that go far beyond the current state of the art (50.0 mIoU), and we report for the first time results on MS COCO for the whole set of 81 classes: our method discovers 34 categories with more than 20% IoU, while obtaining an average IoU of 19.6 for all 81 categories.
[ AD10 ]
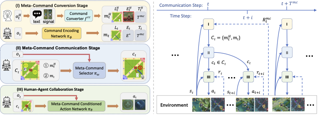
MOBA games, e.g., Dota2 and Honor of Kings, have been actively used as the testbed for the recent AI research on games, and various AI systems have been developed at the human level so far. However, these AI systems mainly focus on how to compete with humans, less on exploring how to collaborate with humans. To this end, this paper makes the first attempt to investigate human-agent collaboration in MOBA games. In this paper, we propose to enable humans and agents to collaborate through explicit communication by designing an efficient and interpretable Meta-Command Communication-based framework, dubbed MCC, for accomplishing effective human-agent collaboration in MOBA games. The MCC framework consists of two pivotal modules: 1) an interpretable communication protocol, i.e., the Meta-Command, to bridge the communication gap between humans and agents; 2) a meta-command value estimator, i.e., the Meta-Command Selector, to select a valuable meta-command for each agent to achieve effective human-agent collaboration. Experimental results in Honor of Kings demonstrate that MCC agents can collaborate reasonably well with human teammates and even generalize to collaborate with different levels and numbers of human teammates. Videos are available at https://sites.google.com/view/mcc-demo.
[ AD11 ]
Neural networks often exhibit emergent behavior in which qualitatively new capabilities that arise from scaling up the number of parameters, training data, or even the number of steps. One approach to understanding emergence is to find the continuous \textit{progress measures} that underlie the seemingly discontinuous qualitative changes. In this work, we argue that progress measures can be found via mechanistic interpretability---that is, by reverse engineering learned models into components and measuring the progress of each component over the course of training. As a case study, we study small transformers trained on a modular arithmetic tasks with emergent grokking behavior. We fully reverse engineer the algorithm learned by these networks, which uses discrete fourier transforms and trigonometric identities to convert addition to rotation about a circle. After confirming the algorithm via ablation, we then use our understanding of the algorithm to define progress measures that precede the grokking phase transition on this task. We see our result as demonstrating both that it is possible to fully reverse engineer trained networks, and that doing so can be invaluable to understanding their training dynamics.
[ Auditorium ]
Recurrent neural networks (RNNs) are well suited for solving sequence tasks in resource-constrained systems due to their expressivity and low computational requirements. However, there is still a need to bridge the gap between what RNNs are capable of in terms of efficiency and performance and real-world application requirements. The memory and computational requirements arising from propagating the activations of all the neurons at every time step to every connected neuron, together with the sequential dependence of activations, contribute to the inefficiency of training and using RNNs. We propose a solution inspired by biological neuron dynamics that makes the communication between RNN units sparse and discrete. This makes the backward pass with backpropagation through time (BPTT) computationally sparse and efficient as well. We base our model on the gated recurrent unit (GRU), extending it with units that emit discrete events for communication triggered by a threshold so that no information is communicated to other units in the absence of events. We show theoretically that the communication between units, and hence the computation required for both the forward and backward passes, scales with the number of events in the network. Our model achieves efficiency without compromising task performance, demonstrating competitive performance compared …
[ AD12 ]
[ AD10 ]

We investigate how multimodal prompt engineering can use language as the intermediate representation to combine complementary knowledge from different pretrained (potentially multimodal) language models for a variety of tasks. This approach is both distinct from and complementary to the dominant paradigm of joint multimodal training. It also recalls a traditional systems-building view as in classical NLP pipelines, but with prompting large pretrained multimodal models. We refer to these as Socratic Models (SMs): a modular class of systems in which multiple pretrained models may be composed zero-shot via multimodal-informed prompting to capture new multimodal capabilities, without additional finetuning. We show that these systems provide competitive state-of-the-art performance for zero-shot image captioning and video-to-text retrieval, and also enable new applications such as (i) answering free-form questions about egocentric video, (ii) engaging in multimodal assistive dialogue with people (e.g., for cooking recipes), and (iii) robot perception and planning. We hope this work provides (a) results for stronger zero-shot baseline performance with analysis also highlighting their limitations, (b) new perspectives for building multimodal systems powered by large pretrained models, and (c) practical application advantages in certain regimes limited by data scarcity, training compute, or model access.
[ AD1 ]
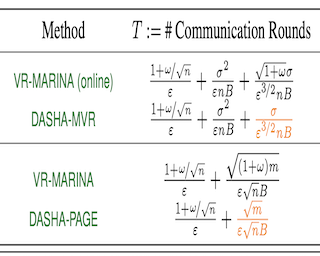
[ AD12 ]
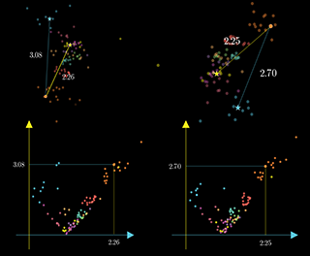
Neural networks embed the geometric structure of a data manifold lying in a high-dimensional space into latent representations. Ideally, the distribution of the data points in the latent space should depend only on the task, the data, the loss, and other architecture-specific constraints. However, factors such as the random weights initialization, training hyperparameters, or other sources of randomness in the training phase may induce incoherent latent spaces that hinder any form of reuse. Nevertheless, we empirically observe that, under the same data and modeling choices, the angles between the encodings within distinct latent spaces do not change. In this work, we propose the latent similarity between each sample and a fixed set of anchors as an alternative data representation, demonstrating that it can enforce the desired invariances without any additional training. We show how neural architectures can leverage these relative representations to guarantee, in practice, invariance to latent isometries and rescalings, effectively enabling latent space communication: from zero-shot model stitching to latent space comparison between diverse settings. We extensively validate the generalization capability of our approach on different datasets, spanning various modalities (images, text, graphs), tasks (e.g., classification, reconstruction) and architectures (e.g., CNNs, GCNs, transformers).
[ AD1 ]
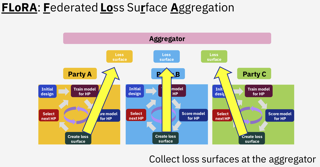
We address the problem of hyper-parameter optimization (HPO) for federated learning (FL-HPO). We introduce Federated Loss SuRface Aggregation (FLoRA), a general FL-HPO solution framework that can address use cases of tabular data and any Machine Learning (ML) model including gradient boosting training algorithms, SVMs, neural networks, among others and thereby further expands the scope of FL-HPO. FLoRA enables single-shot FL-HPO: identifying a single set of good hyper-parameters that are subsequently used in a single FL training. Thus, it enables FL-HPO solutions with minimal additional communication overhead compared to FL training without HPO. Utilizing standard smoothness assumptions, we theoretically characterize the optimality gap of FLoRA for any convex and non-convex loss functions, which explicitly accounts for the heterogeneous nature of the parties' local data distributions, a dominant characteristic of FL systems. Our empirical evaluation of FLoRA for multiple FL algorithms on seven OpenML datasets demonstrates significant model accuracy improvements over the baselines, and robustness to increasing number of parties involved in FL-HPO training.
[ Auditorium ]
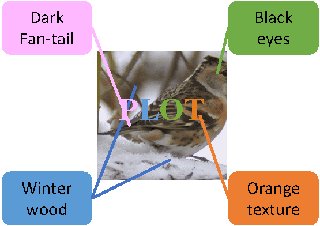
With the increasing attention to large vision-language models such as CLIP, there has been a significant amount of effort dedicated to building efficient prompts. Unlike conventional methods of only learning one single prompt, we propose to learn multiple comprehensive prompts to describe diverse characteristics of categories such as intrinsic attributes or extrinsic contexts. However, directly matching each prompt to the same visual feature is problematic, as it pushes the prompts to converge to one point. To solve this problem, we propose to apply optimal transport to match the vision and text modalities. Specifically, we first model images and the categories with visual and textual feature sets. Then, we apply a two-stage optimization strategy to learn the prompts. In the inner loop, we optimize the optimal transport distance to align visual features and prompts by the Sinkhorn algorithm, while in the outer loop, we learn the prompts by this distance from the supervised data. Extensive experiments are conducted on the few-shot recognition task and the improvement demonstrates the superiority of our method. The code is available at https://github.com/CHENGY12/PLOT.
[ AD11 ]
Models for image segmentation, node classification and many other tasks map a single input to multiple labels. By perturbing this single shared input (e.g. the image) an adversary can manipulate several predictions (e.g. misclassify several pixels). Collective robustness certification is the task of provably bounding the number of robust predictions under this threat model. The only dedicated method that goes beyond certifying each output independently is limited to strictly local models, where each prediction is associated with a small receptive field. We propose a more general collective robustness certificate for all types of models. We further show that this approach is beneficial for the larger class of softly local models, where each output is dependent on the entire input but assigns different levels of importance to different input regions (e.g. based on their proximity in the image). The certificate is based on our novel localized randomized smoothing approach, where the random perturbation strength for different input regions is proportional to their importance for the outputs. Localized smoothing Pareto-dominates existing certificates on both image segmentation and node classification tasks, simultaneously offering higher accuracy and stronger certificates.
[ AD10 ]
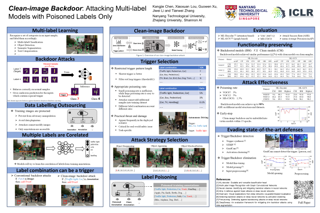
Multi-label models have been widely used in various applications including image annotation and object detection. The fly in the ointment is its inherent vulnerability to backdoor attacks due to the adoption of deep learning techniques. However, all existing backdoor attacks exclusively require to modify training inputs (e.g., images), which may be impractical in real-world applications. In this paper, we aim to break this wall and propose the first clean-image backdoor attack, which only poisons the training labels without touching the training samples. Our key insight is that in a multi-label learning task, the adversary can just manipulate the annotations of training samples consisting of a specific set of classes to activate the backdoor. We design a novel trigger exploration method to find convert and effective triggers to enhance the attack performance. We also propose three target label selection strategies to achieve different goals. Experimental results indicate that our clean-image backdoor can achieve a 98% attack success rate while preserving the model's functionality on the benign inputs. Besides, the proposed clean-image backdoor can evade existing state-of-the-art defenses.
[ AD12 ]
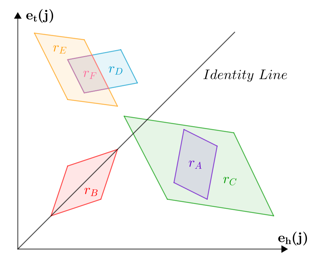
[ Auditorium ]
While some state-of-the-art artificial neural network systems in computer vision are strikingly accurate models of the corresponding primate visual processing, there are still many discrepancies between these models and the behavior of primates on object recognition tasks. Many current models suffer from extreme sensitivity to adversarial attacks and often do not align well with the image-by-image behavioral error patterns observed in humans. Previous research has provided strong evidence that primate object recognition behavior can be very accurately predicted by neural population activity in the inferior temporal (IT) cortex, a brain area in the late stages of the visual processing hierarchy. Therefore, here we directly test whether making the late stage representations of models more similar to that of macaque IT produces new models that exhibit more robust, primate-like behavior. We conducted chronic, large-scale multi-electrode recordings across the IT cortex in six non-human primates (rhesus macaques). We then use these data to fine-tune (end-to-end) the model "IT" representations such that they are more aligned with the biological IT representations, while preserving accuracy on object recognition tasks. We generate a cohort of models with a range of IT similarity scores validated on held-out animals across two image sets with distinct statistics. Across …
[ AD1 ]

[ AD11 ]
Despite recent success of deep learning models in research settings, their application in sensitive domains remains limited because of their opaque decision-making processes. Taking to this challenge, people have proposed various eXplainable AI (XAI) techniques designed to calibrate trust and understandability of black-box models, with the vast majority of work focused on supervised learning. Here, we focus on making an "interpretable-by-design" deep reinforcement learning agent which is forced to use human-friendly prototypes in its decisions, thus making its reasoning process clear. Our proposed method, dubbed Prototype-Wrapper Network (PW-Net), wraps around any neural agent backbone, and results indicate that it does not worsen performance relative to black-box models. Most importantly, we found in a user study that PW-Nets supported better trust calibration and task performance relative to standard interpretability approaches and black-boxes.
[ AD10 ]
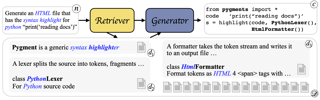
Publicly available source-code libraries are continuously growing and changing. This makes it impossible for models of codeto keep current with all available APIs by simply training these models on existing code repositories. Thus, existing models inherently cannot generalize to using unseen functions and libraries, because these would never appear in the training data. In contrast, when human programmers use functions and libraries for the first time, they frequently refer to textual resources such as code manuals and documentation, to explore and understand the available functionality. Inspired by this observation, we introduce DocPrompting: a natural-language-to-code generation approach that explicitly leverages documentation by (1) retrieving the relevant documentation pieces given an NL intent, and (2) generating code based on the NL intent and the retrieved documentation. DocPrompting is general: it can be applied to any programming language and is agnostic to the underlying neural model. We demonstrate that DocPrompting consistently improves NL-to-code models: DocPrompting improves strong base models such as CodeT5 by 2.85% in pass@1 (52% relative gain) and 4.39% in pass@10 (30% relative gain) in execution-based evaluation on the popular Python CoNaLa benchmark; on a new Bash dataset tldr, DocPrompting improves CodeT5 and GPT-Neo1.3B by up to absolute 6.9% exact match.
[ AD1 ]
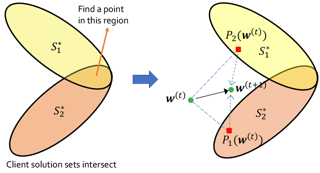
Federated Averaging (FedAvg) remains the most popular algorithm for Federated Learning (FL) optimization due to its simple implementation, stateless nature, and privacy guarantees combined with secure aggregation. Recent work has sought to generalize the vanilla averaging in FedAvg to a generalized gradient descent step by treating client updates as pseudo-gradients and using a server step size. While the use of a server step size has been shown to provide performance improvement theoretically, the practical benefit of the server step size has not been seen in most existing works. In this work, we present FedExP, a method to adaptively determine the server step size in FL based on dynamically varying pseudo-gradients throughout the FL process. We begin by considering the overparameterized convex regime, where we reveal an interesting similarity between FedAvg and the Projection Onto Convex Sets (POCS) algorithm. We then show how FedExP can be motivated as a novel extension to the extrapolation mechanism that is used to speed up POCS. Our theoretical analysis later also discusses the implications of FedExP in underparameterized and non-convex settings. Experimental results show that FedExP consistently converges faster than FedAvg and competing baselines on a range of realistic FL datasets.
[ AD11 ]
[ AD10 ]
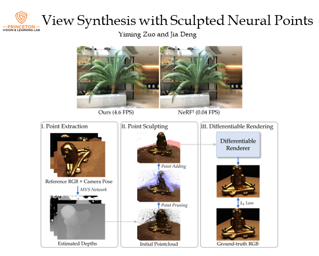
We address the task of view synthesis, generating novel views of a scene given a set of images as input. In many recent works such as NeRF (Mildenhall et al., 2020), the scene geometry is parameterized using neural implicit representations (i.e., MLPs). Implicit neural representations have achieved impressive visual quality but have drawbacks in computational efficiency. In this work, we propose a new approach that performs view synthesis using point clouds. It is the first point-based method that achieves better visual quality than NeRF while being 100× faster in rendering speed. Our approach builds on existing works on differentiable point-based rendering but introduces a novel technique we call “Sculpted Neural Points (SNP)”, which significantly improves the robustness to errors and holes in the reconstructed point cloud. We further propose to use view-dependent point features based on spherical harmonics to capture non-Lambertian surfaces, and new designs in the point-based rendering pipeline that further boost the performance. Finally, we show that our system supports fine-grained scene editing. Code is available at https://github.com/princeton-vl/SNP.
[ Auditorium ]
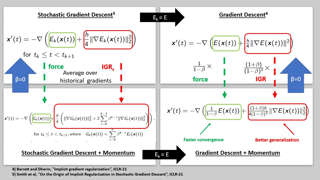
[ AD12 ]
Existing methods for isolating hard subpopulations and spurious correlations in datasets often require human intervention. This can make these methods labor-intensive and dataset-specific. To address these shortcomings, we present a scalable method for automatically distilling a model's failure modes. Specifically, we harness linear classifiers to identify consistent error patterns, and, in turn, induce a natural representation of these failure modes as directions within the feature space. We demonstrate that this framework allows us to discover and automatically caption challenging subpopulations within the training dataset. Moreover, by combining our framework with off-the-shelf diffusion models, we can generate images that are especially challenging for the analyzed model, and thus can be used to perform synthetic data augmentation that helps remedy the model's failure modes.
[ AD1 ]
The message passing-based graph neural networks (GNNs) have achieved great success in many real-world applications.However, training GNNs on large-scale graphs suffers from the well-known neighbor explosion problem, i.e., the exponentially increasing dependencies of nodes with the number of message passing layers. Subgraph-wise sampling methods---a promising class of mini-batch training techniques---discard messages outside the mini-batches in backward passes to avoid the neighbor explosion problem at the expense of gradient estimation accuracy. This poses significant challenges to their convergence analysis and convergence speeds, which seriously limits their reliable real-world applications. To address this challenge, we propose a novel subgraph-wise sampling method with a convergence guarantee, namely Local Message Compensation (LMC). To the best of our knowledge, LMC is the {\it first} subgraph-wise sampling method with provable convergence. The key idea of LMC is to retrieve the discarded messages in backward passes based on a message passing formulation of backward passes. By efficient and effective compensations for the discarded messages in both forward and backward passes, LMC computes accurate mini-batch gradients and thus accelerates convergence. We further show that LMC converges to first-order stationary points of GNNs. Experiments on large-scale benchmark tasks demonstrate that LMC significantly outperforms state-of-the-art subgraph-wise sampling methods in terms …
[ Auditorium ]

Self-attention is key to the remarkable success of transformers in sequence modeling tasks including many applications in natural language processing and computer vision. Like neural network layers, these attention mechanisms are often developed by heuristics and experience. To provide a principled framework for constructing attention layers in transformers, we show that the self-attention corresponds to the support vector expansion derived from a support vector regression problem, whose primal formulation has the form of a neural network layer. Using our framework, we derive popular attention layers used in practice and propose two new attentions: 1) the Batch Normalized Attention (Attention-BN) derived from the batch normalization layer and 2) the Attention with Scaled Head (Attention-SH) derived from using less training data to fit the SVR model. We empirically demonstrate the advantages of the Attention-BN and Attention-SH in reducing head redundancy, increasing the model's accuracy, and improving the model's efficiency in a variety of practical applications including image and time-series classification.
[ AD11 ]
How should we intervene on an unknown structural equation model to maximize a downstream variable of interest? This setting, also known as causal Bayesian optimization (CBO), has important applications in medicine, ecology, and manufacturing. Standard Bayesian optimization algorithms fail to effectively leverage the underlying causal structure. Existing CBO approaches assume noiseless measurements and do not come with guarantees. We propose the {\em model-based causal Bayesian optimization algorithm (MCBO)} that learns a full system model instead of only modeling intervention-reward pairs. MCBO propagates epistemic uncertainty about the causal mechanisms through the graph and trades off exploration and exploitation via the optimism principle. We bound its cumulative regret, and obtain the first non-asymptotic bounds for CBO. Unlike in standard Bayesian optimization, our acquisition function cannot be evaluated in closed form, so we show how the reparameterization trick can be used to apply gradient-based optimizers. The resulting practical implementation of MCBO compares favorably with state-of-the-art approaches empirically.
[ AD12 ]
Many Graph Neural Networks (GNNs) perform poorly compared to simple heuristics on Link Prediction (LP) tasks. This is due to limitations in expressive power such as the inability to count triangles (the backbone of most LP heuristics) and because they can not distinguish automorphic nodes (those having identical structural roles). Both expressiveness issues can be alleviated by learning link (rather than node) representations and incorporating structural features such as triangle counts. Since explicit link representations are often prohibitively expensive, recent works resorted to subgraph-based methods, which have achieved state-of-the-art performance for LP, but suffer from poor efficiency due to high levels of redundancy between subgraphs. We analyze the components of subgraph GNN (SGNN) methods for link prediction. Based on our analysis, we propose a novel full-graph GNN called ELPH (Efficient Link Prediction with Hashing) that passes subgraph sketches as messages to approximate the key components of SGNNs without explicit subgraph construction. ELPH is provably more expressive than Message Passing GNNs (MPNNs). It outperforms existing SGNN models on many standard LP benchmarks while being orders of magnitude faster. However, it shares the common GNN limitation that it is only efficient when the dataset fits in GPU memory. Accordingly, we develop a …
[ AD10 ]
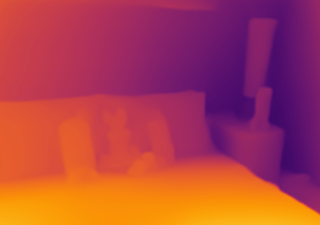
We introduce VA-DepthNet, a simple, effective, and accurate deep neural network approach for the single-image depth prediction (SIDP) problem. The proposed approach advocates using classical first-order variational constraints for this problem. While state-of-the-art deep neural network methods for SIDP learn the scene depth from images in a supervised setting, they often overlook the invaluable invariances and priors in the rigid scene space, such as the regularity of the scene. The paper's main contribution is to reveal the benefit of classical and well-founded variational constraints in the neural network design for the SIDP task. It is shown that imposing first-order variational constraints in the scene space together with popular encoder-decoder-based network architecture design provides excellent results for the supervised SIDP task. The imposed first-order variational constraint makes the network aware of the depth gradient in the scene space, i.e., regularity. The paper demonstrates the usefulness of the proposed approach via extensive evaluation and ablation analysis over several benchmark datasets, such as KITTI, NYU Depth V2, and SUN RGB-D. The VA-DepthNet at test time shows considerable improvements in depth prediction accuracy compared to the prior art and is accurate also at high-frequency regions in the scene space. At the time of writing …
[ AD11 ]
We introduce Corrupted Image Modeling (CIM) for self-supervised visual pre-training. CIM uses an auxiliary generator with a small trainable BEiT to corrupt the input image instead of using artificial [MASK] tokens, where some patches are randomly selected and replaced with plausible alternatives sampled from the BEiT output distribution. Given this corrupted image, an enhancer network learns to either recover all the original image pixels, or predict whether each visual token is replaced by a generator sample or not. The generator and the enhancer are simultaneously trained and synergistically updated. After pre-training, the enhancer can be used as a high-capacity visual encoder for downstream tasks. CIM is a general and flexible visual pre-training framework that is suitable for various network architectures. For the first time, CIM demonstrates that both ViT and CNN can learn rich visual representations using a unified, non-Siamese framework. Experimental results show that our approach achieves compelling results in vision benchmarks, such as ImageNet classification and ADE20K semantic segmentation.
[ AD10 ]
Vision-language models such as CLIP have shown promising performance on a variety of recognition tasks using the standard zero-shot classification procedure -- computing similarity between the query image and the embedded words for each category. By only using the category name, they neglect to make use of the rich context of additional information that language affords. The procedure gives no intermediate understanding of why a category is chosen, and furthermore provides no mechanism for adjusting the criteria used towards this decision. We present an alternative framework for classification with VLMs, which we call classification by description. We ask VLMs to check for descriptive features rather than broad categories: to find a tiger, look for its stripes; its claws; and more. By basing decisions on these descriptors, we can provide additional cues that encourage using the features we want to be used. In the process, we can get a clear idea of what the model thinks" it is seeing to make its decision; it gains some level of inherent explainability. We query large language models (e.g., GPT-3) for these descriptors to obtain them in a scalable way. Extensive experiments show our framework has numerous advantages past interpretability. We show improvements in …
[ AD12 ]

It is unclear how changing the learning rule of a deep neural network alters its learning dynamics and representations. To gain insight into the relationship between learned features, function approximation, and the learning rule, we analyze infinite-width deep networks trained with gradient descent (GD) and biologically-plausible alternatives including feedback alignment (FA), direct feedback alignment (DFA), and error modulated Hebbian learning (Hebb), as well as gated linear networks (GLN). We show that, for each of these learning rules, the evolution of the output function at infinite width is governed by a time varying effective neural tangent kernel (eNTK). In the lazy training limit, this eNTK is static and does not evolve, while in the rich mean-field regime this kernel's evolution can be determined self-consistently with dynamical mean field theory (DMFT). This DMFT enables comparisons of the feature and prediction dynamics induced by each of these learning rules. In the lazy limit, we find that DFA and Hebb can only learn using the last layer features, while full FA can utilize earlier layers with a scale determined by the initial correlation between feedforward and feedback weight matrices. In the rich regime, DFA and FA utilize a temporally evolving and depth-dependent NTK. Counterintuitively, …
[ AD1 ]
This paper presents a systematic study of multi-objective online learning. We first formulate the framework of Multi-Objective Online Convex Optimization, which encompasses a novel multi-objective regret. This regret is built upon a sequence-wise extension of the commonly used discrepancy metric Pareto suboptimality gap in zero-order multi-objective bandits. We then derive an equivalent form of the regret, making it amenable to be optimized via first-order iterative methods. To motivate the algorithm design, we give an explicit example in which equipping OMD with the vanilla min-norm solver for gradient composition will incur a linear regret, which shows that merely regularizing the iterates, as in single-objective online learning, is not enough to guarantee sublinear regrets in the multi-objective setting. To resolve this issue, we propose a novel min-regularized-norm solver that regularizes the composite weights. Combining min-regularized-norm with OMD results in the Doubly Regularized Online Mirror Multiple Descent algorithm. We further derive the multi-objective regret bound for the proposed algorithm, which matches the optimal bound in the single-objective setting. Extensive experiments on real-world datasets verify the effectiveness of the proposed algorithm.
[ Auditorium ]
Recent studies have started to explore the integration of logical knowledge into deep learning via encoding logical constraints as an additional loss function. However, existing approaches tend to vacuously satisfy logical constraints through shortcuts, failing to fully exploit the knowledge. In this paper, we present a new framework for learning with logical constraints. Specifically, we address the shortcut satisfaction issue by introducing dual variables for logical connectives, encoding how the constraint is satisfied. We further propose a variational framework where the encoded logical constraint is expressed as a distributional loss that is compatible with the model's original training loss. The theoretical analysis shows that the proposed approach bears some nice properties, and the experimental evaluations demonstrate its superior performance in both model generalizability and constraint satisfaction.
[ Auditorium ]
We consider the problem of training a deep neural network on a given classification task, e.g., ImageNet-1K (IN1K), so that it excels at both the training task as well as at other (future) transfer tasks. These two seemingly contradictory properties impose a trade-off between improving the model’s generalization and maintaining its performance on the original task. Models trained with self-supervised learning tend to generalize better than their supervised counterparts for transfer learning; yet, they still lag behind supervised models on IN1K. In this paper, we propose a supervised learning setup that leverages the best of both worlds. We extensively analyze supervised training using multi-scale crops for data augmentation and an expendable projector head, and reveal that the design of the projector allows us to control the trade-off between performance on the training task and transferability. We further replace the last layer of class weights with class prototypes computed on the fly using a memory bank and derive two models: t-ReX that achieves a new state of the art for transfer learning and outperforms top methods such as DINO and PAWS on IN1K, and t-ReX* that matches the highly optimized RSB-A1 model on IN1K while performing better on transfer tasks.Code and …
[ AD12 ]
Pruning neural networks at initialization (PaI) has received an upsurge of interest due to its end-to-end saving potential. PaI is able to find sparse subnetworks at initialization that can achieve comparable performance to the full networks. These methods can surpass the trivial baseline of random pruning but suffer from a significant performance gap compared to post-training pruning. Previous approaches firmly rely on weights, gradients, and sanity checks as primary signals when conducting PaI analysis. To better understand the underlying mechanism of PaI, we propose to interpret it through the lens of the Ramanujan Graph - a class of expander graphs that are sparse while being highly connected. It is often believed there should be a strong correlation between the Ramanujan graph and PaI since both are about finding sparse and well-connected neural networks. However, the finer-grained link relating highly sparse and connected networks to their relative performance (i.e., ranking of difference sparse structures at the same specific global sparsity) is still missing. We observe that not only the Ramanujan property for sparse networks shows no significant relationship to PaI’s relative performance, but maximizing it can also lead to the formation of pseudo-random graphs with no structural meanings. We reveal the …
[ AD11 ]
From human physiology to environmental evolution, important processes in nature often exhibit meaningful and strong periodic or quasi-periodic changes. Due to their inherent label scarcity, learning useful representations for periodic tasks with limited or no supervision is of great benefit. Yet, existing self-supervised learning (SSL) methods overlook the intrinsic periodicity in data, and fail to learn representations that capture periodic or frequency attributes. In this paper, we present SimPer, a simple contrastive SSL regime for learning periodic information in data. To exploit the periodic inductive bias, SimPer introduces customized augmentations, feature similarity measures, and a generalized contrastive loss for learning efficient and robust periodic representations. Extensive experiments on common real-world tasks in human behavior analysis, environmental sensing, and healthcare domains verify the superior performance of SimPer compared to state-of-the-art SSL methods, highlighting its intriguing properties including better data efficiency, robustness to spurious correlations, and generalization to distribution shifts.
[ AD10 ]
Many machine learning problems today have multiple objective functions. They appear either in learning with multiple criteria where learning has to make a trade-off between multiple performance metrics such as fairness, safety and accuracy; or, in multi-task learning where multiple tasks are optimized jointly, sharing inductive bias between them. This problems are often tackled by the multi-objective optimization framework. However, existing stochastic multi-objective gradient methods and its variants (e.g., MGDA, PCGrad, CAGrad, etc.) all adopt a biased noisy gradient direction, which leads to degraded empirical performance. To this end, we develop a stochastic multi-objective gradient correction (MoCo) method for multi-objective optimization. The unique feature of our method is that it can guarantee convergence without increasing the batch size even in the nonconvex setting. Simulations on multi-task supervised and reinforcement learning demonstrate the effectiveness of our method relative to the state-of-the-art methods.
[ AD1 ]

Effective data-driven PDE forecasting methods often rely on fixed spatial and / or temporal discretizations. This raises limitations in real-world applications like weather prediction where flexible extrapolation at arbitrary spatiotemporal locations is required. We address this problem by introducing a new data-driven approach, DINo, that models a PDE's flow with continuous-time dynamics of spatially continuous functions. This is achieved by embedding spatial observations independently of their discretization via Implicit Neural Representations in a small latent space temporally driven by a learned ODE. This separate and flexible treatment of time and space makes DINo the first data-driven model to combine the following advantages. It extrapolates at arbitrary spatial and temporal locations; it can learn from sparse irregular grids or manifolds; at test time, it generalizes to new grids or resolutions. DINo outperforms alternative neural PDE forecasters in a variety of challenging generalization scenarios on representative PDE systems.
[ AD12 ]
Advances in the expressivity of pretrained models have increased interest in the design of adaptation protocols which enable safe and effective transfer learning. Going beyond conventional linear probing (LP) and fine tuning (FT) strategies, protocols that can effectively control feature distortion, i.e., the failure to update features orthogonal to the in-distribution, have been found to achieve improved out-of-distribution generalization (OOD). In order to limit this distortion, the LP+FT protocol, which first learns a linear probe and then uses this initialization for subsequent FT, was proposed. However, in this paper, we find when adaptation protocols (LP, FT, LP+FT) are also evaluated on a variety of safety objectives (e.g., calibration, robustness, etc.), a complementary perspective to feature distortion is helpful to explain protocol behavior. To this end, we study the susceptibility of protocols to simplicity bias (SB), i.e. the well-known propensity of deep neural networks to rely upon simple features, as SB has recently been shown to underlie several problems in robust generalization. Using a synthetic dataset, we demonstrate the susceptibility of existing protocols to SB. Given the strong effectiveness of LP+FT, we then propose modified linear probes that help mitigate SB, and lead to better initializations for subsequent FT. We verify …
[ Auditorium ]
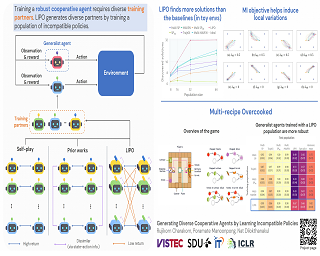
Training a robust cooperative agent requires diverse partner agents. However, obtaining those agents is difficult. Previous works aim to learn diverse behaviors by changing the state-action distribution of agents. But, without information about the task's goal, the diversified agents are not guided to find other important, albeit sub-optimal, solutions: the agents might learn only variations of the same solution. In this work, we propose to learn diverse behaviors via policy compatibility. Conceptually, policy compatibility measures whether policies of interest can coordinate effectively. We theoretically show that incompatible policies are not similar. Thus, policy compatibility—which has been used exclusively as a measure of robustness—can be used as a proxy for learning diverse behaviors. Then, we incorporate the proposed objective into a population-based training scheme to allow concurrent training of multiple agents. Additionally, we use state-action information to induce local variations of each policy. Empirically, the proposed method consistently discovers more solutions than baseline methods across various multi-goal cooperative environments. Finally, in multi-recipe Overcooked, we show that our method produces populations of behaviorally diverse agents, which enables generalist agents trained with such a population to be more robust.See our project page at https://bit.ly/marl-lipo
[ AD11 ]
[ Auditorium ]
Language models are defined over a finite set of inputs, which creates a vocabulary bottleneck when we attempt to scale the number of supported languages. Tackling this bottleneck results in a trade-off between what can be represented in the embedding matrix and computational issues in the output layer. This paper introduces PIXEL, the Pixel-based Encoder of Language, which suffers from neither of these issues. PIXEL is a pretrained language model that renders text as images, making it possible to transfer representations across languages based on orthographic similarity or the co-activation of pixels. PIXEL is trained to reconstruct the pixels of masked patches instead of predicting a distribution over tokens. We pretrain the 86M parameter PIXEL model on the same English data as BERT and evaluate on syntactic and semantic tasks in typologically diverse languages, including various non-Latin scripts. We find that PIXEL substantially outperforms BERT on syntactic and semantic processing tasks on scripts that are not found in the pretraining data, but PIXEL is slightly weaker than BERT when working with Latin scripts. Furthermore, we find that PIXEL is more robust than BERT to orthographic attacks and linguistic code-switching, further confirming the benefits of modelling language with pixels.
[ AD1 ]
[ AD4 ]
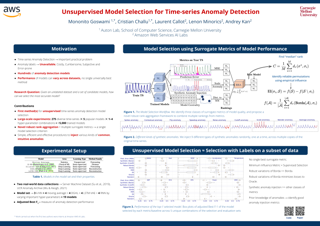
[ AD11 ]
Deformable object manipulation (DOM) is a long-standing challenge in robotics and has attracted significant interest recently. This paper presents DaXBench, a differentiable simulation framework for DOM. While existing work often focuses on a specific type of deformable objects, DaXBench supports fluid, rope, cloth ...; it provides a general-purpose benchmark to evaluate widely different DOM methods, including planning, imitation learning, and reinforcement learning. DaXBench combines recent advances in deformable object simulation with JAX, a high-performance computational framework. All DOM tasks in DaXBench are wrapped with the OpenAI Gym API for easy integration with DOM algorithms. We hope that DaXBench provides to the research community a comprehensive, standardized benchmark and a valuable tool to support the development and evaluation of new DOM methods. The code and video are available online.
[ AD10 ]
Though end-to-end neural approaches have recently been dominating NLP tasks in both performance and ease-of-use, they lack interpretability and robustness. We propose Binder, a training-free neural-symbolic framework that maps the task input to a program, which (1) allows binding a unified API of language model (LM) functionalities to a programming language (e.g., SQL, Python) to extend its grammar coverage and thus tackle more diverse questions, (2) adopts an LM as both the program parser and the underlying model called by the API during execution, and (3) requires only a few in-context exemplar annotations. Specifically, we employ GPT-3 Codex as the LM. In the parsing stage, with only a few in-context exemplars, Codex is able to identify the part of the task input that cannot be answerable by the original programming language, correctly generate API calls to prompt Codex to solve the unanswerable part, and identify where to place the API calls while being compatible with the original grammar. In the execution stage, Codex can perform versatile functionalities (e.g., commonsense QA, information extraction) given proper prompts in the API calls. Binder achieves state-of-the-art results on WikiTableQuestions and TabFact datasets, with explicit output programs that benefit human debugging. Note that previous best …
[ AD12 ]
Gradient-based learning algorithms have an implicit \emph{simplicity bias} which in effect can limit the diversity of predictors being sampled by the learning procedure. This behavior can hinder the transferability of trained models by (i) favoring the learning of simpler but spurious features --- present in the training data but absent from the test data --- and (ii) by only leveraging a small subset of predictive features. Such an effect is especially magnified when the test distribution does not exactly match the train distribution---referred to as the Out of Distribution (OOD) generalization problem. However, given only the training data, it is not always possible to apriori assess if a given feature is spurious or transferable. Instead, we advocate for learning an ensemble of models which capture a diverse set of predictive features. Towards this, we propose a new algorithm D-BAT (Diversity-By-disAgreement Training), which enforces agreement among the models on the training data, but disagreement on the OOD data. We show how D-BAT naturally emerges from the notion of generalized discrepancy, as well as demonstrate in multiple experiments how the proposed method can mitigate shortcut-learning, enhance uncertainty and OOD detection, as well as improve transferability.
[ Auditorium ]
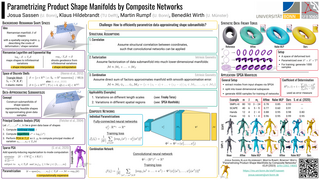
Parametrizations of data manifolds in shape spaces can be computed using the rich toolbox of Riemannian geometry. This, however, often comes with high computational costs, which raises the question if one can learn an efficient neural network approximation. We show that this is indeed possible for shape spaces with a special product structure, namely those smoothly approximable by a direct sum of low-dimensional manifolds. Our proposed architecture leverages this structure by separately learning approximations for the low-dimensional factors and a subsequent combination. After developing the approach as a general framework, we apply it to a shape space of triangular surfaces. Here, typical examples of data manifolds are given through datasets of articulated models and can be factorized, for example, by a Sparse Principal Geodesic Analysis (SPGA). We demonstrate the effectiveness of our proposed approach with experiments on synthetic data as well as manifolds extracted from data via SPGA.
[ AD10 ]
We consider the task of generating realistic 3D shapes, which is useful for a variety of applications such as automatic scene generation and physical simulation. Compared to other 3D representations like voxels and point clouds, meshes are more desirable in practice, because (1) they enable easy and arbitrary manipulation of shapes for relighting and simulation, and (2) they can fully leverage the power of modern graphics pipelines which are mostly optimized for meshes. Previous scalable methods for generating meshes typically rely on sub-optimal post-processing, and they tend to produce overly-smooth or noisy surfaces without fine-grained geometric details. To overcome these shortcomings, we take advantage of the graph structure of meshes and use a simple yet very effective generative modeling method to generate 3D meshes. Specifically, we represent meshes with deformable tetrahedral grids, and then train a diffusion model on this direct parameterization. We demonstrate the effectiveness of our model on multiple generative tasks.
[ AD12 ]
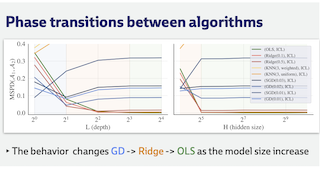
[ AD1 ]
[ AD4 ]
Over-parameterized residual networks (ResNets) are amongst the most successful convolutional neural architectures for image processing. Here we study their properties through their Gaussian Process and Neural Tangent kernels. We derive explicit formulas for these kernels, analyze their spectra, and provide bounds on their implied condition numbers. Our results indicate that (1) with ReLU activation, the eigenvalues of these residual kernels decay polynomially at a similar rate compared to the same kernels when skip connections are not used, thus maintaining a similar frequency bias; (2) however, residual kernels are more locally biased. Our analysis further shows that the matrices obtained by these residual kernels yield favorable condition numbers at finite depths than those obtained without the skip connections, enabling therefore faster convergence of training with gradient descent.
[ AD11 ]
[ AD4 ]
While large language models (LLMs) have demonstrated impressive capabilities across tasks in language understanding and interactive decision making, their abilities for reasoning (e.g. chain-of-thought prompting) and acting (e.g. action plan generation) have primarily been studied as separate topics. In this paper, we explore the use of LLMs to generate both reasoning traces and task-specific actions in an interleaved manner, allowing for greater synergy between the two: reasoning traces help the model induce, track, and update action plans as well as handle exceptions, while actions allow it to interface with external sources, such as knowledge bases or environments, to gather additional information. We apply our approach, named ReAct, to a diverse set of language and decision making tasks and demonstrate its effectiveness over state-of-the-art baselines, as well as improved human interpretability and trustworthiness over methods without reasoning or acting components. Concretely, on question answering (HotpotQA) and fact verification (Fever), ReAct overcomes issues of hallucination and error propagation prevalent in chain-of-thought reasoning by interacting with a simple Wikipedia API, and generates human-like task-solving trajectories that are more interpretable than baselines without reasoning traces. On two interactive decision making benchmarks (ALFWorld and WebShop), ReAct outperforms imitation and reinforcement learning methods by an absolute …
[ AD12 ]
We study the problem of choosing algorithm hyper-parameters in unsupervised domain adaptation, i.e., with labeled data in a source domain and unlabeled data in a target domain, drawn from a different input distribution. We follow the strategy to compute several models using different hyper-parameters, and, to subsequently compute a linear aggregation of the models. While several heuristics exist that follow this strategy, methods are still missing that rely on thorough theories for bounding the target error. In this turn, we propose a method that extends weighted least squares to vector-valued functions, e.g., deep neural networks. We show that the target error of the proposed algorithm is asymptotically not worse than twice the error of the unknown optimal aggregation. We also perform a large scale empirical comparative study on several datasets, including text, images, electroencephalogram, body sensor signals and signals from mobile phones. Our method outperforms deep embedded validation (DEV) and importance weighted validation (IWV) on all datasets, setting a new state-of-the-art performance for solving parameter choice issues in unsupervised domain adaptation with theoretical error guarantees. We further study several competitive heuristics, all outperforming IWV and DEV on at least five datasets. However, our method outperforms each heuristic on at least …
[ AD10 ]

Crossmodal knowledge distillation (KD) extends traditional knowledge distillation to the area of multimodal learning and demonstrates great success in various applications. To achieve knowledge transfer across modalities, a pretrained network from one modality is adopted as the teacher to provide supervision signals to a student network learning from the other modality. In contrast to the empirical success reported in prior works, the working mechanism of crossmodal KD remains a mystery. In this paper, we present a thorough understanding of crossmodal KD. We begin by providing two failure cases and demonstrate that KD is not a universal cure in crossmodal knowledge transfer. We then present the modality Venn diagram to understand modality relationships and the modality focusing hypothesis revealing the decisive factor in the efficacy of crossmodal KD. Experimental results on 6 multimodal datasets help justify our hypothesis, diagnose failure cases, and point directions to improve crossmodal knowledge transfer in the future.
[ AD11 ]
As large language models (LLMs) grow larger and more sophisticated, assessing their "reasoning" capabilities in natural language grows more challenging. Recent question answering (QA) benchmarks that attempt to assess reasoning are often limited by a narrow scope of covered situations and subject matters. We introduce WikiWhy, a QA dataset built around a novel auxiliary task: explaining why an answer is true in natural language. WikiWhy contains over 9,000 "why" question-answer-rationale triples, grounded on Wikipedia facts across a diverse set of topics. Each rationale is a set of supporting statements connecting the question to the answer. WikiWhy serves as a benchmark for the reasoning capabilities of LLMs because it demands rigorous explicit rationales for each answer to demonstrate the acquisition of implicit commonsense knowledge, which is unlikely to be easily memorized. GPT-3 baselines achieve only 38.7% human-evaluated correctness in the end-to-end answer & explain condition, leaving significant room for future improvements.
[ AD1 ]
We formally study how \emph{ensemble} of deep learning models can improve test accuracy, and how the superior performance of ensemble can be distilled into a single model using \emph{knowledge distillation}. We consider the challenging case where the ensemble is simply an average of the outputs of a few independently trained neural networks with the \emph{same} architecture, trained using the \emph{same} algorithm on the \emph{same} data set, and they only differ by the random seeds used in the initialization.We show that ensemble/knowledge distillation in \emph{deep learning} works very differently from traditional learning theory (such as boosting or NTKs). We develop a theory showing that when data has a structure we refer to as multi-view'', then ensemble of independently trained neural networks can provably improve test accuracy, and such superior test accuracy can also be provably distilled into a single model. Our result sheds light on how ensemble works in deep learning in a way that is completely different from traditional theorems, and how the
dark knowledge'' is hidden in the outputs of the ensemble and can be used in distillation.
[ Auditorium ]
Deep learning vision systems are widely deployed across applications where reliability is critical. However, even today's best models can fail to recognize an object when its pose, lighting, or background varies. While existing benchmarks surface examples challenging for models, they do not explain why such mistakes arise. To address this need, we introduce ImageNet-X—a set of sixteen human annotations of factors such as pose, background, or lighting the entire ImageNet-1k validation set as well as a random subset of 12k training images. Equipped with ImageNet-X, we investigate 2,200 current recognition models and study the types of mistakes as a function of model’s (1) architecture, e.g. transformer vs. convolutional, (2) learning paradigm, e.g. supervised vs. self-supervised, and (3) training procedures, e.g., data augmentation. Regardless of these choices, we find models have consistent failure modes across ImageNet-X categories. We also find that while data augmentation can improve robustness to certain factors, they induce spill-over effects to other factors. For example, color-jitter augmentation improves robustness to color and brightness, but surprisingly hurts robustness to pose. Together, these insights suggest to advance the robustness of modern vision models, future research should focus on collecting additional data and understanding data augmentation schemes. Along with these …
[ AD1 ]
[ AD12 ]
This paper novelly breaks down with ignorable loss an RNN layer into a sequence of simple RNNs, each of which can be further rewritten into a lightweight positional encoding matrix of a self-attention, named the Recurrence Encoding Matrix (REM). Thus, recurrent dynamics introduced by the RNN layer can be encapsulated into the positional encodings of a multihead self-attention, and this makes it possible to seamlessly incorporate these recurrent dynamics into a Transformer, leading to a new module, Self-Attention with Recurrence (RSA). The proposed module can leverage the recurrent inductive bias of REMs to achieve a better sample efficiency than its corresponding baseline Transformer, while the self-attention is used to model the remaining non-recurrent signals. The relative proportions of these two components are controlled by a data-driven gated mechanism, and the effectiveness of RSA modules are demonstrated by four sequential learning tasks.
[ Auditorium ]
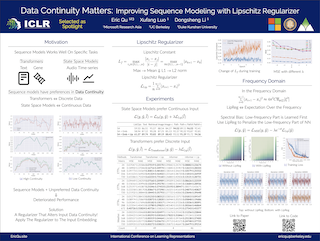
Sequence modeling is a core problem in machine learning, and various neural networks have been designed to process different types of sequence data. However, few attempts have been made to understand the inherent data property of sequence data, neglecting the critical factor that may significantly affect the performance of sequence modeling. In this paper, we theoretically and empirically analyze a generic property of sequence data, i.e., continuity, and connect this property with the performance of deep models. First, we empirically observe that different kinds of models for sequence modeling prefer data with different continuity. Then, we theoretically analyze the continuity preference of different models in both time and frequency domains. To further utilize continuity to improve sequence modeling, we propose a simple yet effective Lipschitz Regularizer, that can flexibly adjust data continuity according to model preferences, and bring very little extra computational cost. Extensive experiments on various tasks demonstrate that altering data continuity via Lipschitz Regularizer can largely improve the performance of many deep models for sequence modeling.
[ AD11 ]

A multitude of work has shown that machine learning-based medical diagnosis systems can be biased against certain subgroups of people. This has motivated a growing number of bias mitigation algorithms that aim to address fairness issues in machine learning. However, it is difficult to compare their effectiveness in medical imaging for two reasons. First, there is little consensus on the criteria to assess fairness. Second, existing bias mitigation algorithms are developed under different settings, e.g., datasets, model selection strategies, backbones, and fairness metrics, making a direct comparison and evaluation based on existing results impossible. In this work, we introduce MEDFAIR, a framework to benchmark the fairness of machine learning models for medical imaging. MEDFAIR covers eleven algorithms from various categories, ten datasets from different imaging modalities, and three model selection criteria. Through extensive experiments, we find that the under-studied issue of model selection criterion can have a significant impact on fairness outcomes; while in contrast, state-of-the-art bias mitigation algorithms do not significantly improve fairness outcomes over empirical risk minimization (ERM) in both in-distribution and out-of-distribution settings. We evaluate fairness from various perspectives and make recommendations for different medical application scenarios that require different ethical principles. Our framework provides a reproducible …
[ AD10 ]
Although Transformer architectures have been successfully applied to graph data with the advent of Graph Transformer, current design of Graph Transformer still heavily relies on human labor and expertise knowledge to decide proper neural architectures and suitable graph encoding strategies at each Transformer layer. In literature, there have been some works on automated design of Transformers focusing on non-graph data such as texts and images without considering graph encoding strategies, which fail to handle the non-euclidean graph data. In this paper, we study the problem of automated graph Transformer, for the first time. However, solving these problems poses the following challenges: i) how can we design a unified search space for graph Transformer, and ii) how to deal with the coupling relations between Transformer architectures and the graph encodings of each Transformer layer. To address these challenges, we propose Automated Graph Transformer (AutoGT), a neural architecture search framework that can automatically discover the optimal graph Transformer architectures by joint optimization of Transformer architecture and graph encoding strategies. Specifically, we first propose a unified graph Transformer formulation that can represent most of state-of-the-art graph Transformer architectures. Based upon the unified formulation, we further design the graph Transformer search space that includes …
[ AD4 ]
When deployed for risk-sensitive tasks, deep neural networks must be able to detect instances with labels from outside the distribution for which they were trained.In this paper we present a novel framework to benchmark the ability of image classifiers to detect class-out-of-distribution instances(i.e., instances whose true labels do not appear in the training distribution) at various levels of detection difficulty.We apply this technique to ImageNet, and benchmark 525 pretrained, publicly available, ImageNet-1k classifiers. The code for generating a benchmark for any ImageNet-1k classifier, along with the benchmarks prepared for the above-mentioned 525 models is available at https://github.com/mdabbah/COOD_benchmarking.The usefulness of the proposed framework and its advantage over alternative existing benchmarks is demonstrated by analyzing the results obtained for these models, which reveals numerous novel observations including:(1) knowledge distillation consistently improves class-out-of-distribution (C-OOD) detection performance; (2) a subset of ViTs performs better C-OOD detection than any other model; (3) the language–-vision CLIP model achieves good zero-shot detection performance, with its best instance outperforming 96% of all other models evaluated; (4) accuracy and in-distribution ranking are positively correlated to C-OOD detection; and (5) we compare various confidence functions for C-OOD detection.Our companion paper, also published in ICLR 2023 (What Can We Learn From …
[ AD10 ]
Most existing Image Restoration (IR) models are task-specific, which can not be generalized to different degradation operators. In this work, we propose the Denoising Diffusion Null-Space Model (DDNM), a novel zero-shot framework for arbitrary linear IR problems, including but not limited to image super-resolution, colorization, inpainting, compressed sensing, and deblurring. DDNM only needs a pre-trained off-the-shelf diffusion model as the generative prior, without any extra training or network modifications. By refining only the null-space contents during the reverse diffusion process, we can yield diverse results satisfying both data consistency and realness. We further propose an enhanced and robust version, dubbed DDNM+, to support noisy restoration and improve restoration quality for hard tasks. Our experiments on several IR tasks reveal that DDNM outperforms other state-of-the-art zero-shot IR methods. We also demonstrate that DDNM+ can solve complex real-world applications, e.g., old photo restoration.
[ AD12 ]
[ AD11 ]
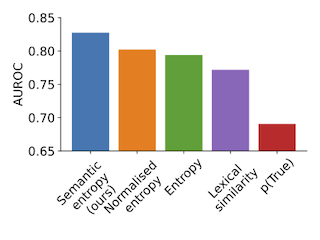
We introduce a method to measure uncertainty in large language models. For tasks like question answering, it is essential to know when we can trust the natural language outputs of foundation models. We show that measuring uncertainty in natural language is challenging because of "semantic equivalence"—different sentences can mean the same thing. To overcome these challenges we introduce semantic entropy—an entropy which incorporates linguistic invariances created by shared meanings. Our method is unsupervised, uses only a single model, and requires no modifications to off-the-shelf language models. In comprehensive ablation studies we show that the semantic entropy is more predictive of model accuracy on question answering data sets than comparable baselines.
[ AD4 ]
Deep Ensembles (DE) are a prominent approach for achieving excellent performance on key metrics such as accuracy, calibration, uncertainty estimation, and out-of-distribution detection. However, hardware limitations of real-world systems constrain to smaller ensembles and lower-capacity networks, significantly deteriorating their performance and properties. We introduce Packed-Ensembles (PE), a strategy to design and train lightweight structured ensembles by carefully modulating the dimension of their encoding space. We leverage grouped convolutions to parallelize the ensemble into a single shared backbone and forward pass to improve training and inference speeds. PE is designed to operate within the memory limits of a standard neural network. Our extensive research indicates that PE accurately preserves the properties of DE, such as diversity, and performs equally well in terms of accuracy, calibration, out-of-distribution detection, and robustness to distribution shift. We make our code available at https://github.com/ENSTA-U2IS/torch-uncertainty.
[ AD1 ]
[ Auditorium ]
Neural Algorithmic Reasoning is an emerging area of machine learning which seeks to infuse algorithmic computation in neural networks, typically by training neural models to approximate steps of classical algorithms. In this context, much of the current work has focused on learning reachability and shortest path graph algorithms, showing that joint learning on similar algorithms is beneficial for generalisation. However, when targeting more complex problems, such "similar" algorithms become more difficult to find. Here, we propose to learn algorithms by exploiting duality of the underlying algorithmic problem. Many algorithms solve optimisation problems. We demonstrate that simultaneously learning the dual definition of these optimisation problems in algorithmic learning allows for better learning and qualitatively better solutions. Specifically, we exploit the max-flow min-cut theorem to simultaneously learn these two algorithms over synthetically generated graphs, demonstrating the effectiveness of the proposed approach. We then validate the real-world utility of our dual algorithmic reasoner by deploying it on a challenging brain vessel classification task, which likely depends on the vessels’ flow properties. We demonstrate a clear performance gain when using our model within such a context, and empirically show that learning the max-flow and min-cut algorithms together is critical for achieving such a result.
[ AD12 ]
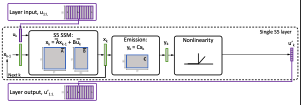
[ AD10 ]

Graph neural network (GNN) is a powerful learning approach for graph-based recommender systems. Recently, GNNs integrated with contrastive learning have shown superior performance in recommendation with their data augmentation schemes, aiming at dealing with highly sparse data. Despite their success, most existing graph contrastive learning methods either perform stochastic augmentation (e.g., node/edge perturbation) on the user-item interaction graph, or rely on the heuristic-based augmentation techniques (e.g., user clustering) for generating contrastive views. We argue that these methods cannot well preserve the intrinsic semantic structures and are easily biased by the noise perturbation. In this paper, we propose a simple yet effective graph contrastive learning paradigm LightGCL that mitigates these issues impairing the generality and robustness of CL-based recommenders. Our model exclusively utilizes singular value decomposition for contrastive augmentation, which enables the unconstrained structural refinement with global collaborative relation modeling. Experiments conducted on several benchmark datasets demonstrate the significant improvement in performance of our model over the state-of-the-arts. Further analyses demonstrate the superiority of LightGCL's robustness against data sparsity and popularity bias. The source code of our model is available at https://github.com/HKUDS/LightGCL.
[ Auditorium ]
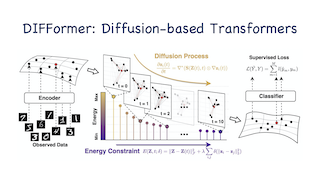
Real-world data generation often involves complex inter-dependencies among instances, violating the IID-data hypothesis of standard learning paradigms and posing a challenge for uncovering the geometric structures for learning desired instance representations. To this end, we introduce an energy constrained diffusion model which encodes a batch of instances from a dataset into evolutionary states that progressively incorporate other instances' information by their interactions. The diffusion process is constrained by descent criteria w.r.t. a principled energy function that characterizes the global consistency of instance representations over latent structures. We provide rigorous theory that implies closed-form optimal estimates for the pairwise diffusion strength among arbitrary instance pairs, which gives rise to a new class of neural encoders, dubbed as DIFFormer (diffusion-based Transformers), with two instantiations: a simple version with linear complexity for prohibitive instance numbers, and an advanced version for learning complex structures. Experiments highlight the wide applicability of our model as a general-purpose encoder backbone with superior performance in various tasks, such as node classification on large graphs, semi-supervised image/text classification, and spatial-temporal dynamics prediction. The codes are available at https://github.com/qitianwu/DIFFormer.
[ AD11 ]
Post hoc auditing of model fairness suffers from potential drawbacks: (1) auditing may be highly sensitive to the test samples chosen; (2) the model and/or its training data may need to be shared with an auditor thereby breaking confidentiality. We address these issues by instead providing a certificate that demonstrates that the learning algorithm itself is fair, and hence, as a consequence, so too is the trained model. We introduce a method to provide a confidential proof of fairness for training, in the context of widely used decision trees, which we term Confidential-PROFITT. We propose novel fair decision tree learning algorithms along with customized zero-knowledge proof protocols to obtain a proof of fairness that can be audited by a third party. Using zero-knowledge proofs enables us to guarantee confidentiality of both the model and its training data. We show empirically that bounding the information gain of each node with respect to the sensitive attributes reduces the unfairness of the final tree. In extensive experiments on the COMPAS, Communities and Crime, Default Credit, and Adult datasets, we demonstrate that a company can use Confidential-PROFITT to certify the fairness of their decision tree to an auditor in less than 2 minutes, thus …
[ AD1 ]
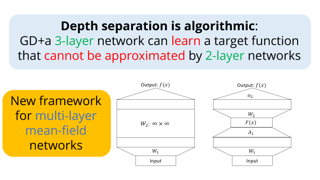
Depth separation—why a deeper network is more powerful than a shallow one—has been a major problem in deep learning theory. Previous results often focus on representation power, for example, Safran et al. (2019) constructed a function that is easy to approximate using a 3-layer network but not approximable by any 2-layer network. In this paper, we show that this separation is in fact algorithmic: one can learn the function constructed by Safran et al. (2019) using an overparametrized network with polynomially many neurons efficiently. Our result relies on a new way of extending the mean-field limit to multilayer networks, and a decomposition of loss that factors out the error introduced by the discretization of infinite-width mean-field networks.
[ AD12 ]
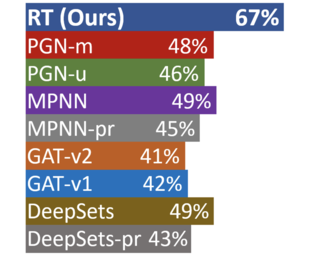
Transformers flexibly operate over sets of real-valued vectors representing task-specific entities and their attributes, where each vector might encode one word-piece token and its position in a sequence, or some piece of information that carries no position at all. As set processors, transformers are at a disadvantage in reasoning over more general graph-structured data where nodes represent entities and edges represent relations between entities. To address this shortcoming, we generalize transformer attention to consider and update edge vectors in each transformer layer. We evaluate this relational transformer on a diverse array of graph-structured tasks, including the large and challenging CLRS Algorithmic Reasoning Benchmark. There, it dramatically outperforms state-of-the-art graph neural networks expressly designed to reason over graph-structured data. Our analysis demonstrates that these gains are attributable to relational attention's inherent ability to leverage the greater expressivity of graphs over sets.
[ AD11 ]
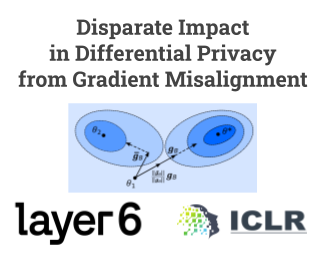
As machine learning becomes more widespread throughout society, aspects including data privacy and fairness must be carefully considered, and are crucial for deployment in highly regulated industries. Unfortunately, the application of privacy enhancing technologies can worsen unfair tendencies in models. In particular, one of the most widely used techniques for private model training, differentially private stochastic gradient descent (DPSGD), frequently intensifies disparate impact on groups within data. In this work we study the fine-grained causes of unfairness in DPSGD and identify gradient misalignment due to inequitable gradient clipping as the most significant source. This observation leads us to a new method for reducing unfairness by preventing gradient misalignment in DPSGD.
[ AD10 ]
To obtain, deterministic guarantees of adversarial robustness, specialized training methods are used. We propose, SABR, a novel such certified training method, based on the key insight that propagating interval bounds for a small but carefully selected subset of the adversarial input region is sufficient to approximate the worst-case loss over the whole region while significantly reducing approximation errors. We show in an extensive empirical evaluation that SABR outperforms existing certified defenses in terms of both standard and certifiable accuracies across perturbation magnitudes and datasets, pointing to a new class of certified training methods promising to alleviate the robustness-accuracy trade-off.
[ Auditorium ]
Class-incremental few-shot learning, where new sets of classes are provided sequentially with only a few training samples, presents a great challenge due to catastrophic forgetting of old knowledge and overfitting caused by lack of data. During finetuning on new classes, the performance on previous classes deteriorates quickly even when only a small fraction of parameters are updated, since the previous knowledge is broadly associated with most of the model parameters in the original parameter space. In this paper, we introduce WaRP, the \textit{weight space rotation process}, which transforms the original parameter space into a new space so that we can push most of the previous knowledge compactly into only a few important parameters. By properly identifying and freezing these key parameters in the new weight space, we can finetune the remaining parameters without affecting the knowledge of previous classes. As a result, WaRP provides an additional room for the model to effectively learn new classes in future incremental sessions. Experimental results confirm the effectiveness of our solution and show the improved performance over the state-of-the-art methods.
[ AD1 ]
Learning high-dimensional distributions is often done with explicit likelihood modeling or implicit modeling via minimizing integral probability metrics (IPMs). In this paper, we expand this learning paradigm to stochastic orders, namely, the convex or Choquet order between probability measures. Towards this end, exploiting the relation between convex orders and optimal transport, we introduce the Choquet-Toland distance between probability measures, that can be used as a drop-in replacement for IPMs. We also introduce the Variational Dominance Criterion (VDC) to learn probability measures with dominance constraints, that encode the desired stochastic order between the learned measure and a known baseline. We analyze both quantities and show that they suffer from the curse of dimensionality and propose surrogates via input convex maxout networks (ICMNs), that enjoy parametric rates. We provide a min-max framework for learning with stochastic orders and validate it experimentally on synthetic and high-dimensional image generation, with promising results. Finally, our ICMNs class of convex functions and its derived Rademacher Complexity are of independent interest beyond their application in convex orders. Code to reproduce experimental results is available at https://github.com/yair-schiff/stochastic-orders-ICMN.
[ Auditorium ]
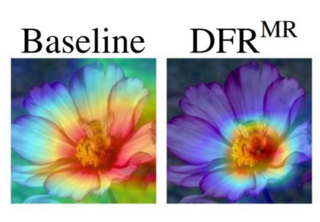
Neural network classifiers can largely rely on simple spurious features, such as image backgrounds, to make predictions. However, even in these cases, we show that they still often learn core features associated with the desired attributes of the data, contrary to recent findings. Inspired by this insight, we demonstrate that simple last layer retraining can match or outperform state-of-the-art approaches on spurious correlation benchmarks, but with profoundly lower complexity and computational expenses. Moreover, we show that last layer retraining on large ImageNet-trained models can also significantly reduce reliance on background and texture information, improving robustness to covariate shift, after only minutes of training on a single GPU.
[ AD10 ]
[ AD12 ]
Human visual perception can easily generalize to out-of-distributed visual data, which is far beyond the capability of modern machine learning models. Domain generalization (DG) aims to close this gap, with existing DG methods mainly focusing on the loss function design. In this paper, we propose to explore an orthogonal direction, i.e., the design of the backbone architecture. It is motivated by an empirical finding that transformer-based models trained with empirical risk minimization (ERM) outperform CNN-based models employing state-of-the-art (SOTA) DG algorithms on multiple DG datasets. We develop a formal framework to characterize a network's robustness to distribution shifts by studying its architecture's alignment with the correlations in the dataset. This analysis guides us to propose a novel DG model built upon vision transformers, namely \emph{Generalizable Mixture-of-Experts (GMoE)}. Extensive experiments on DomainBed demonstrate that GMoE trained with ERM outperforms SOTA DG baselines by a large margin. Moreover, GMoE is complementary to existing DG methods and its performance is substantially improved when trained with DG algorithms.
[ AD1 ]
Discontinuous solutions arise in a large class of hyperbolic and advection-dominated PDEs. This paper investigates, both theoretically and empirically, the operator learning of PDEs with discontinuous solutions. We rigorously prove, in terms of lower approximation bounds, that methods which entail a linear reconstruction step (e.g. DeepONets or PCA-Nets) fail to efficiently approximate the solution operator of such PDEs. In contrast, we show that certain methods employing a non-linear reconstruction mechanism can overcome these fundamental lower bounds and approximate the underlying operator efficiently. The latter class includes Fourier Neural Operators and a novel extension of DeepONets termed shift-DeepONets. Our theoretical findings are confirmed by empirical results for advection equations, inviscid Burgers’ equation and the compressible Euler equations of gas dynamics.